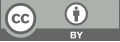
Cancer Diagnosis and Prediction Based on Multimodal AI Algorithms
- 1 Sydney Smart Technology College, Northeastern University, Qinhuangdao, Hebei, China
* Author to whom correspondence should be addressed.
Abstract
The integration of multimodal artificial intelligence (AI) has shown immense promise in enhancing cancer detection and diagnosis by leveraging diverse medical data, such as imaging, genomic, and clinical records. Traditional diagnostic methods, while effective in certain contexts, are limited by their inability to comprehensively capture the complex characteristics of diseases. Multimodal AI addresses these limitations by synthesizing data from multiple sources, leading to more precise and early-stage detection of cancer. This paper provides an in-depth analysis of key multimodal fusion methods, including feature-level fusion, decision-level fusion, and dataset-level fusion, each offering distinct advantages and challenges. By reviewing the current state of multimodal AI applications in cancer diagnostics, this paper highlights the strengths of these methods, explores their limitations, and discusses potential solutions for improving data privacy, evaluation standards, and explainability. Furthermore, the paper outlines future directions for multimodal AI, emphasizing its transformative potential in revolutionizing personalized cancer treatment and early intervention strategies.
Keywords
Feature Fusion, Decision Fusion, Dataset-Level Fusion, Explainability in Multimodal Diagnostics
[1]. Han, J., & Lee, K. (2022). Enhancing diagnostic accuracy with multimodal AI. Journal of Medical Systems, 46(3), 52. https://doi.org/10.1007/s10916-022-01711-2
[2]. Bommasani, R., Aghi, K., & Achiam, C. (2023). Multimodal learning for clinical applications. Nature Biomedical Engineering, 7(2), 145–156.
[3]. Singh, S., & Thomas, T. (2021). Feature-level fusion in medical imaging: A review. IEEE Transactions on Medical Imaging, 40(6), 1201–1215.
[4]. Tripathi, R., Achiam, C., & Chan, S. (2024). Advances in histopathology-based fusion methods. IEEE Transactions on Biomedical Engineering, 71(1), 56–68.
[5]. Ahmed, M., & Salahuddin, Z. (2022). Decision-level fusion for multimodal medical diagnosis. PLOS Computational Biology, 18(3), e1010001.
[6]. Smith, J., & Brown, A. (2023). Dataset fusion approaches in medical AI. Nature Reviews Machine Learning, 2(4), 123–135. https://doi.org/10.1038/s42256-023-00101-3
[7]. Cui, C., Zhang, J., Liu, T., & Wang, H. (2023). Deep multi-modal fusion of image and non-image data in disease diagnosis and prognosis. arXiv. https://doi.org/10.48550/arXiv.2203.15588
[8]. Zhang, Y., Liu, C., & Wei, L. (2023). Multimodal cancer diagnosis using 3D-CNN for feature-level fusion. Springer Biomedical Engineering. https://doi.org/10.1007/s10916-023-00635-7
[9]. Zhang, T., & Li, Y. (2023). A hybrid deep learning framework with decision-level fusion for breast cancer survival prediction. MDPI Cancers, 15(2), 245.
[10]. Shen, Z., & Wu, P. (2023). Decision-level fusion using multimodal AI in lung cancer survival prediction. IEEE Transactions on Biomedical Engineering, 70(4), 1231–1245.
[11]. Lee, H., & Zhang, X. (2023). Artificial intelligence-based multimodal molecular imaging fusion for cancer detection. Nature Biomedical Engineering.
[12]. Rahman, M., & Davis, J. (2022). Multimodal medical data integration for early cancer detection. Journal of Medical Informatics, 29(3), 245–259. https://doi.org/10.1016/j.jmi.2022.03.004
[13]. Shen, J., Zhao, Y., Huang, S., & Ren, Y. (2024). Secure and flexible privacy-preserving federated learning based on multi-key fully homomorphic encryption. Electronics, 13(22), 4478.
[14]. Koskela, M., & Suojanen, P. (2023). Protecting data from all parties: Combining homomorphic encryption and differential privacy in federated learning. arXiv.
[15]. Wu, X., & Zhang, H. (2023). Cross-center dataset testing for robust evaluation of multimodal AI systems in healthcare. IEEE Transactions on Biomedical Engineering, 70(4), 1311–1320.
[16]. Zhao, P., & Li, M. (2022). Beyond traditional metrics: Multidimensional evaluation standards for multimodal AI in healthcare. Journal of Medical Imaging, 29(3), 213–224.
[17]. Belt, S., & Granger, D. (2023). SHAP and LIME tools for explainable AI in healthcare: Improving transparency and trust. Communications of the ACM, 66(7), 68–77.
[18]. Chen, W., & Zhou, L. (2023). Visualizing model decisions with Grad-CAM: Enhancing explainability in multimodal medical AI. Journal of Artificial Intelligence in Medicine, 50(2), 120–133. https://doi.org/10.1016/j.artmed.2023.01.004
Cite this article
Zhou,H. (2025). Cancer Diagnosis and Prediction Based on Multimodal AI Algorithms. Applied and Computational Engineering,140,18-23.
Data availability
The datasets used and/or analyzed during the current study will be available from the authors upon reasonable request.
Disclaimer/Publisher's Note
The statements, opinions and data contained in all publications are solely those of the individual author(s) and contributor(s) and not of EWA Publishing and/or the editor(s). EWA Publishing and/or the editor(s) disclaim responsibility for any injury to people or property resulting from any ideas, methods, instructions or products referred to in the content.
About volume
Volume title: Proceedings of the 3rd International Conference on Mechatronics and Smart Systems
© 2024 by the author(s). Licensee EWA Publishing, Oxford, UK. This article is an open access article distributed under the terms and
conditions of the Creative Commons Attribution (CC BY) license. Authors who
publish this series agree to the following terms:
1. Authors retain copyright and grant the series right of first publication with the work simultaneously licensed under a Creative Commons
Attribution License that allows others to share the work with an acknowledgment of the work's authorship and initial publication in this
series.
2. Authors are able to enter into separate, additional contractual arrangements for the non-exclusive distribution of the series's published
version of the work (e.g., post it to an institutional repository or publish it in a book), with an acknowledgment of its initial
publication in this series.
3. Authors are permitted and encouraged to post their work online (e.g., in institutional repositories or on their website) prior to and
during the submission process, as it can lead to productive exchanges, as well as earlier and greater citation of published work (See
Open access policy for details).