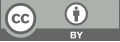
Optimization of Relevant Functions of Urban Computing in the Direction of Traffic Flow Prediction
- 1 School of Traffic & Transportation Engineering, Central South University, Changsha, Hunan, China
* Author to whom correspondence should be addressed.
Abstract
As an important application direction of urban computing, traffic flow prediction plays an important role in modern traffic management, urban planning and sustainable development. In recent years, many cutting-edge studies in the field of traffic flow prediction have had a significant impact and promoted the development of practical applications in this field. This paper mainly focuses on the research results of various traffic prediction directions. According to the actual environment and the functional characteristics of the research results, the research is classified into three aspects: data acquisition, feature engineering, and prediction model optimization. It also summarizes the optimization effects of research on traffic flow prediction in sensor data acquisition, data outlier processing, neural network prediction technology, etc. This paper first proposes three important aspects that affect traffic flow prediction and classifies recent research results. Then, the functions and impacts are analyzed from various aspects, and the advantages and progress of the research results are analyzed by comparing most mainstream methods. Then, the problems and limitations of the research are analyzed and discussed in combination with the actual road environment. Finally, the future research direction and development trend of this field are prospected, and the full text is summarized.
Keywords
urban computing, traffic flow forecasting, predictive models, feature engineering, Data acquisition
[1]. Zheng, Y., Capra, L., Wolfson, O., & Yang, H. (2014). Urban computing: concepts, methodologies, and applications. ACM Transactions on Intelligent Systems and Technology (TIST), 5(3), 1-55.
[2]. Wu, H., Wen, H., Zhang, G., Xia, Y., Wang, K., Liang, Y., ... & Wang, K. (2024). DynST: Dynamic Sparse Training for Resource-Constrained Spatio-Temporal Forecasting. arXiv preprint arXiv:2403.02914.
[3]. Zheng, Y., Koh, H. Y., Jin, M., Chi, L., Wang, H., Phan, K. T., ... & Xiang, W. (2024). Graph spatiotemporal process for multivariate time series anomaly detection with missing values. Information Fusion, 106, 102255.
[4]. Du, S., Yang, T., Teng, F., Zhang, J., Li, T., & Zheng, Y. (2024). Multi-scale feature enhanced spatio-temporal learning for traffic flow forecasting: knowledge-based Systems, 294, 111787.
[5]. Wang, R., Liu, Y., Gong, Y., Liu, W., Chen, M., Yin, Y., & Zheng, Y. (2023, December). Fine-grained Urban Flow Inference with Unobservable Data via Space-Time Attraction Learning. In 2023 IEEE International Conference on Data Mining (ICDM) (pp. 1367-1372). IEEE.
[6]. Savvides, A., Han, C. C., & Strivastava, M. B. (2001, July). Dynamic fine-grained localization in ad-hoc networks of sensors. In Proceedings of the 7th annual International Conference on Mobile Computing and Networking (pp. 166-179).
[7]. Vilela, J., Kashino, Z., Ly, R., Nejat, G., & Benhabib, B. (2016). A dynamic approach to sensor network deployment for mobile-target detection in unstructured, expanding search areas. IEEE Sensors Journal, 16(11), 4405-4417.
[8]. Ma, Z., Meng, C., Ren, H., Ruan, S., Bao, J., Wang, X., ... & Zheng, Y. (2023, August). SAInf: Stay Area Inference of Vehicles using Surveillance Camera Records. In Proceedings of the 29th ACM SIGKDD Conference on Knowledge Discovery and Data Mining (pp. 4595-4604).
[9]. Zheng, Y., Koh, H. Y., Jin, M., Chi, L., Wang, H., Phan, K. T., ... & Xiang, W. (2024). Graph spatiotemporal process for multivariate time series anomaly detection with missing values. Information Fusion, 106, 102255.
[10]. Ke, S., Pan, Z., He, T., Liang, Y., Zhang, J., & Zheng, Y. (2023). AutoSTG+: An automatic framework to discover the optimal network for spatio-temporal graph prediction. Artificial Intelligence, 318, 103899.
[11]. Ma, Z., Tu, Z., Chen, X., Zhang, Y., Xia, D., Zhou, G., ... & Gong, J. (2024, May). More Than Routing: Joint GPS and Route Modeling for Refine Trajectory Representation Learning. In Proceedings of the ACM on Web Conference 2024 (pp. 3064-3075).
[12]. Zheng, Y. (2015). Trajectory data mining: an overview. ACM Transactions on Intelligent Systems and Technology (TIST), 6(3), 1-41.
[13]. Feng, S., Wei, S., Zhang, J., Li, Y., Ke, J., Chen, G., ... & Yang, H. (2023). A macro-micro spatio-temporal neural network for traffic prediction. Transportation research part C: emerging technologies, 156, 104331.
[14]. Li, X., Gong, Y., Liu, W., Yin, Y., Zheng, Y., & Nie, L. (2024). Dual-track spatio-temporal learning for urban flow prediction with adaptive normalization. Artificial Intelligence, 328, 104065. [15] Wang, K., Wu, H., Duan, Y., Zhang, G., Wang, K., Peng, X., ... & Wang, Y. (2024). NuwaDynamics: Discovering and Updating in Causal Spatio-Temporal Modeling. In The Twelfth International Conference on Learning Representations.
Cite this article
Zhang,R. (2025). Optimization of Relevant Functions of Urban Computing in the Direction of Traffic Flow Prediction. Applied and Computational Engineering,140,24-30.
Data availability
The datasets used and/or analyzed during the current study will be available from the authors upon reasonable request.
Disclaimer/Publisher's Note
The statements, opinions and data contained in all publications are solely those of the individual author(s) and contributor(s) and not of EWA Publishing and/or the editor(s). EWA Publishing and/or the editor(s) disclaim responsibility for any injury to people or property resulting from any ideas, methods, instructions or products referred to in the content.
About volume
Volume title: Proceedings of the 3rd International Conference on Mechatronics and Smart Systems
© 2024 by the author(s). Licensee EWA Publishing, Oxford, UK. This article is an open access article distributed under the terms and
conditions of the Creative Commons Attribution (CC BY) license. Authors who
publish this series agree to the following terms:
1. Authors retain copyright and grant the series right of first publication with the work simultaneously licensed under a Creative Commons
Attribution License that allows others to share the work with an acknowledgment of the work's authorship and initial publication in this
series.
2. Authors are able to enter into separate, additional contractual arrangements for the non-exclusive distribution of the series's published
version of the work (e.g., post it to an institutional repository or publish it in a book), with an acknowledgment of its initial
publication in this series.
3. Authors are permitted and encouraged to post their work online (e.g., in institutional repositories or on their website) prior to and
during the submission process, as it can lead to productive exchanges, as well as earlier and greater citation of published work (See
Open access policy for details).