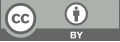
Analysis Model Integrating Traffic Volume and Temperature for Traffic Flow Prediction
- 1 College of Metropolitan Transportation, Beijing University of Technology, Beijing, China
* Author to whom correspondence should be addressed.
Abstract
Rising global vehicle numbers lead to traffic congestion, which is influenced by temperature. Nowadays, temperature forecasting has reached a high level of sophistication, so it is feasible to predict traffic volume based on the forecasting temperatures. To predict the traffic volume, collected the traffic and weather data of I-94 Interstate highway. Based on the data, the simple linear regression model (LR), polynomial regression model (PR) and random forest (RF) were applied. With the comparison between simulation performances, RF demonstrated the highest accuracy, with a correlation coefficient (R2) of 0.8364. To bolster the simulation performances of regression models, more constraints should be involved such as rainfall, snowfall, and cloud cover, should also be considered. Besides, more sophisticated algorithms, such as regression-enhanced random forests (RERFs) and improved random forest classifiers (RFC), should be applied as well. In conclusion, as more related factors are involved, the performance of regression models will be better, which serve for traffic management effectively, with accurate traffic flow prediction.
Keywords
traffic volume, temperature, regression model, simulation
[1]. Zahoor A, Mehr F, Mao G, et al. (2023). The carbon neutrality feasibility of worldwide and in China's transportation sector by E-car and renewable energy sources before 2060[J]. Journal of Energy Storage, 61: 106696.
[2]. Moody J, Farr E, Papagelis M, et al. (2021). The value of car ownership and use in the United States[J]. Nature Sustainability, 4(9): 769-774.
[3]. Li X, Yin X, Huang X, et al. (2024). Multi-dynamic residual graph convolutional network with global feature enhancement for traffic flow prediction[J]. International Journal of Machine Learning and Cybernetics, 1-17.
[4]. Bayati A, Nguyen K K, Cheriet M. (2020). Gaussian process regression ensemble model for network traffic prediction[J]. IEEE Access, 8: 176540-176554.
[5]. Li D.(2020). Predicting short-term traffic flow in urban based on multivariate linear regression model[J]. Journal of Intelligent & Fuzzy Systems, 39(2): 1417-1427.
[6]. Yu J, Feng Z, Ju L, et al. (2024). Traffic Flow Prediction Method Based on Improved Optimization Algorithm Mixed Kernel Extreme Learning Machine[C]//Proceedings of the International Conference on Algorithms, Software Engineering, and Network Security. 54-58.
[7]. Huang X, Ma C, Zhao Y, et al. (2023). A hybrid model of neural network with VMD–CNN–GRU for traffic flow prediction[J]. International Journal of Modern Physics C, 34(12): 2350159.
[8]. Yu Z, Yang J, Huang H H. (2024). Smoothing regression and impact measures for accidents of traffic flows[J]. Journal of applied statistics, 51(6): 1041-1056.
[9]. Abbass K, Qasim M Z, Song H, et al. (2022). A review of the global climate change impacts, adaptation, and sustainable mitigation measures[J]. Environmental Science and Pollution Research, 29(28): 42539-42559.
[10]. Arnell N W, Lowe J A, Challinor A J, et al. (2019). Global and regional impacts of climate change at different levels of global temperature increase[J]. Climatic Change, 155: 377-391.
[11]. Obradovich N, Fowler J H. Climate change may alter human physical activity patterns[J]. (2017). Nature Human Behaviour, 1(5): 0097.
[12]. Zhou X, Yu Z, Yuan L, et al. (2020). Measuring accessibility of healthcare facilities for populations with multiple transportation modes considering residential transportation mode choice[J]. ISPRS International Journal of Geo-Information, 9(6): 394.
[13]. Johnstone C, Sulungu E D. (2023). Application of neural network in prediction of temperature: a review[J]. Neural computing and applications, 2021, 33(18): 11487-11498.
Cite this article
Liu,Y. (2025). Analysis Model Integrating Traffic Volume and Temperature for Traffic Flow Prediction. Applied and Computational Engineering,140,31-37.
Data availability
The datasets used and/or analyzed during the current study will be available from the authors upon reasonable request.
Disclaimer/Publisher's Note
The statements, opinions and data contained in all publications are solely those of the individual author(s) and contributor(s) and not of EWA Publishing and/or the editor(s). EWA Publishing and/or the editor(s) disclaim responsibility for any injury to people or property resulting from any ideas, methods, instructions or products referred to in the content.
About volume
Volume title: Proceedings of the 3rd International Conference on Mechatronics and Smart Systems
© 2024 by the author(s). Licensee EWA Publishing, Oxford, UK. This article is an open access article distributed under the terms and
conditions of the Creative Commons Attribution (CC BY) license. Authors who
publish this series agree to the following terms:
1. Authors retain copyright and grant the series right of first publication with the work simultaneously licensed under a Creative Commons
Attribution License that allows others to share the work with an acknowledgment of the work's authorship and initial publication in this
series.
2. Authors are able to enter into separate, additional contractual arrangements for the non-exclusive distribution of the series's published
version of the work (e.g., post it to an institutional repository or publish it in a book), with an acknowledgment of its initial
publication in this series.
3. Authors are permitted and encouraged to post their work online (e.g., in institutional repositories or on their website) prior to and
during the submission process, as it can lead to productive exchanges, as well as earlier and greater citation of published work (See
Open access policy for details).