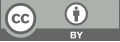
Load Forecasting Method Based on QPSO-LSTM
- 1 South-Central Minzu University, Hubei Province of China
* Author to whom correspondence should be addressed.
Abstract
With the continuous expansion of the power system scale and the increasing growth of electricity demand, accurate load forecasting is of great significance for the safe, stable operation and economic dispatch of the power system. Traditional load forecasting methods have certain limitations in handling complex load data and are difficult to meet actual needs. This paper proposes a load forecasting method based on the Quantum Particle Swarm Optimization(QPSO) algorithm and the Long Short-Term Memory(LSTM) network. Firstly, the load data is pre-processed, including normalization and dataset division. Then, the LSTM model is used to learn the time-series characteristics of the load data. At the same time, the QPSO algorithm is adopted to optimize the hyperparameters of the LSTM model to improve the prediction performance of the model. Through practical example analysis, it is shown that this method can effectively improve the accuracy and stability of load forecasting. Compared with traditional methods, it performs better in handling complex load data and can provide a more reliable decision-making basis for the planning and operation of the power system.
Keywords
Load Forecasting,Long Short-Term Memory , Quantum-behaved Particle Swarm Optimization
[1]. Qian Yushu, Kong Yuting, Huang Cong. Review of Research on Power Load Forecasting[J]. Sichuan Electric Power Technology, 2023, 46(04): 3-43+58.
[2]. Li Xiaoliang, Li Ziping, Liu Jiazheng. Research on Medium-and Long - Term Load Forecasting in a Certain Area Based on Tim -Series Analysis[J]. Modern Industrial Economy and Informationization, 2024, 14(07): 282-283+287.
[3]. Wang Yanling, Wu Mengkai. Medium - and Long - Term Load Forecasting Model Based on Partial Least Squares Regression under the New Economic Normal[J]. Electric Power Automation Equipment, 2018, 38(03): 13-139.
[4]. Ma Yangyang, Zhang Jiangong, Chen Yu, et al. Analysis of Load Forecasting for Distribution Network Planning Based on Improved Grey Model[J]. Qinghai Electric Power, 2024, 43(02): 48-52+64.
[5]. Jiang Haiyang, Zhou Ruibing, Wang Shuogang, et al. Short - Term Load Forecasting of the Power Grid in Hohhot Area Based on Big Data and BP Neural Network[J]. Power Big Data, 2020, 23(11): 47-54.
Cite this article
Li,W. (2025). Load Forecasting Method Based on QPSO-LSTM. Applied and Computational Engineering,142,18-22.
Data availability
The datasets used and/or analyzed during the current study will be available from the authors upon reasonable request.
Disclaimer/Publisher's Note
The statements, opinions and data contained in all publications are solely those of the individual author(s) and contributor(s) and not of EWA Publishing and/or the editor(s). EWA Publishing and/or the editor(s) disclaim responsibility for any injury to people or property resulting from any ideas, methods, instructions or products referred to in the content.
About volume
Volume title: Proceedings of MSS 2025 Symposium: Automation and Smart Technologies in Petroleum Engineering
© 2024 by the author(s). Licensee EWA Publishing, Oxford, UK. This article is an open access article distributed under the terms and
conditions of the Creative Commons Attribution (CC BY) license. Authors who
publish this series agree to the following terms:
1. Authors retain copyright and grant the series right of first publication with the work simultaneously licensed under a Creative Commons
Attribution License that allows others to share the work with an acknowledgment of the work's authorship and initial publication in this
series.
2. Authors are able to enter into separate, additional contractual arrangements for the non-exclusive distribution of the series's published
version of the work (e.g., post it to an institutional repository or publish it in a book), with an acknowledgment of its initial
publication in this series.
3. Authors are permitted and encouraged to post their work online (e.g., in institutional repositories or on their website) prior to and
during the submission process, as it can lead to productive exchanges, as well as earlier and greater citation of published work (See
Open access policy for details).