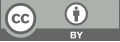
Behavioral Responses to AI Financial Advisors: Trust Dynamics and Decision Quality Among Retail Investors
- 1 Computer Science, California State University Long Beach, CA, USA
- 2 Computer Science, Rice University, TX, USA
- 3 Computer Science, University of California, San Diego, CA, USA
- 4 Computer Science, University of Texas at Dallas, Richardson, TX, USA
* Author to whom correspondence should be addressed.
Abstract
This study examines trust dynamics and decision quality among retail investors interacting with AI financial advisors. Using a convergent parallel mixed methods design incorporating surveys (n=428) and interviews (n=42), we investigate how trust forms, evolves, and influences investment outcomes. Results demonstrate that institutional affiliation (β=0.68, p<0.001) and perceived competence (β=0.57, p<0.001) significantly influence initial trust formation, while system explainability features substantially impact trust sustainability (high-explainability M=4.76 vs. low-explainability M=3.28, p<0.001, d=1.78). Longitudinal analysis reveals non-linear trust trajectories with distinct investor classes showing increasing (58.2%), stable (23.7%), or degrading (18.1%) patterns over nine months. AI-advised portfolios outperformed self-directed investments by 7.2% on risk-adjusted returns (Sharpe ratio: 0.83 vs. 0.65, p<0.01) with significant reductions in overconfidence bias (41.3%) and disposition effect (37.8%). Financial literacy moderates these benefits, with high-literacy investors showing smaller performance differentials between AI-advised and self-directed conditions compared to low-literacy participants. Transparency regarding system limitations demonstrates particularly strong effects on trust calibration, with 76.4% of participants in transparent conditions exhibiting appropriate reliance patterns versus 34.2% in non-disclosure conditions. These findings advance understanding of human-AI interaction in financial contexts and provide implications for designing trustworthy advisory systems that enhance retail investor outcomes.
Keywords
AI financial advisors, trust dynamics, decision quality, explainable AI
[1]. Pillai, D., Singh, A. S., Bhosale, T., & Doifode, A. (2023, August). A Perspective on the Application of Fintech as a Gateway for Financial Literacy. In 2023 International Conference on Information Technology (ICIT) (pp. 811-814). IEEE.
[2]. Srivastav, S., & Gupta, A. (2022, November). AI Application and Psychological Biases in Behavior Finance. In 2022 3rd International Conference on Computation, Automation and Knowledge Management (ICCAKM) (pp. 1-7). IEEE.
[3]. Embarak, O. (2023, May). Decoding the black box: A comprehensive review of explainable artificial intelligence. In 2023 9th International Conference on Information Technology Trends (ITT) (pp. 108-113). IEEE.
[4]. Fernando, E., & Touriano, D. (2018, November). Development and validation of instruments adoption FinTech services in Indonesia (Perspective of trust and risk). In 2018 International conference on sustainable information engineering and technology (SIET) (pp. 283-287). IEEE.
[5]. Pangavhane, P., Kolse, S., Avhad, P., Gadekar, T., Darwante, N. K., & Chaudhari, S. V. (2023, December). Transforming Finance Through Automation Using AI-Driven Personal Finance Advisors. In 2023 4th International Conference on Computation, Automation and Knowledge Management (ICCAKM) (pp. 1-5). IEEE.
[6]. Wei, M., Wang, S., Pu, Y., & Wu, J. (2024). Multi-Agent Reinforcement Learning for High-Frequency Trading Strategy Optimization. Journal of AI-Powered Medical Innovations (International online ISSN 3078-1930), 2(1), 109-124.
[7]. Ma, D., Jin, M., Zhou, Z., Wu, J., & Liu, Y. (2024). Deep Learning-Based ADL Assessment and Personalized Care Planning Optimization in Adult Day Health Center. Applied and Computational Engineering, 118, 14-22.
[8]. Ma, X., Bi, W., Li, M., Liang, P., & Wu, J. (2025). An Enhanced LSTM-based Sales Forecasting Model for Functional Beverages in Cross-Cultural Markets. Applied and Computational Engineering, 118, 55-63.
[9]. Sun, J., Zhou, S., Zhan, X., & Wu, J. (2024). Enhancing Supply Chain Efficiency with Time Series Analysis and Deep Learning Techniques.
[10]. Wang, P., Varvello, M., Ni, C., Yu, R., & Kuzmanovic, A. (2021, May). Web-lego: trading content strictness for faster webpages. In IEEE INFOCOM 2021-IEEE Conference on Computer Communications (pp. 1-10). IEEE.
[11]. Ni, C., Zhang, C., Lu, W., Wang, H., & Wu, J. (2024). Enabling Intelligent Decision Making and Optimization in Enterprises through Data Pipelines.
[12]. Zheng, Shuaiqi, Yining Zhang, and Yuexing Chen. "Leveraging Financial Sentiment Analysis for Detecting Abnormal Stock Market Volatility: An Evidence-Based Approach from Social Media Data." Academia Nexus Journal3.3 (2024).
[13]. Zhang, C., Lu, W., Ni, C., Wang, H., & Wu, J. (2024, June). Enhanced user interaction in operating systems through machine learning language models. In International Conference on Image, Signal Processing, and Pattern Recognition (ISPP 2024) (Vol. 13180, pp. 1623-1630). SPIE.
[14]. Wang, H., Wu, J., Zhang, C., Lu, W., & Ni, C. (2024). Intelligent security detection and defense in operating systems based on deep learning. International Journal of Computer Science and Information Technology, 2(1), 359-367.
[15]. Zheng, S., Zhang, Y., & Chen, Y. (2024). Leveraging Financial Sentiment Analysis for Detecting Abnormal Stock Market Volatility: An Evidence-Based Approach from Social Media Data. Academia Nexus Journal, 3(3).
[16]. Ma, D., & Ling, Z. (2024). Optimization of Nursing Staff Allocation in Elderly Care Institutions: A Time Series Data Analysis Approach. Annals of Applied Sciences, 5(1).
[17]. Diao, S., Wan, Y., Huang, D., Huang, S., Sadiq, T., Khan, M. S., ... & Mazhar, T. (2025). Optimizing Bi-LSTM networks for improved lung cancer detection accuracy. PloS one, 20(2), e0316136.
[18]. W. Xu, J. Xiao, and J. Chen, “Leveraging large language models to enhance personalized recommendations in e-commerce,” arXiv, arXiv:2410.12829, 2024.
Cite this article
Trinh,T.K.;Jia,G.;Cheng,C.;Ni,C. (2025). Behavioral Responses to AI Financial Advisors: Trust Dynamics and Decision Quality Among Retail Investors. Applied and Computational Engineering,144,69-79.
Data availability
The datasets used and/or analyzed during the current study will be available from the authors upon reasonable request.
Disclaimer/Publisher's Note
The statements, opinions and data contained in all publications are solely those of the individual author(s) and contributor(s) and not of EWA Publishing and/or the editor(s). EWA Publishing and/or the editor(s) disclaim responsibility for any injury to people or property resulting from any ideas, methods, instructions or products referred to in the content.
About volume
Volume title: Proceedings of the 3rd International Conference on Functional Materials and Civil Engineering
© 2024 by the author(s). Licensee EWA Publishing, Oxford, UK. This article is an open access article distributed under the terms and
conditions of the Creative Commons Attribution (CC BY) license. Authors who
publish this series agree to the following terms:
1. Authors retain copyright and grant the series right of first publication with the work simultaneously licensed under a Creative Commons
Attribution License that allows others to share the work with an acknowledgment of the work's authorship and initial publication in this
series.
2. Authors are able to enter into separate, additional contractual arrangements for the non-exclusive distribution of the series's published
version of the work (e.g., post it to an institutional repository or publish it in a book), with an acknowledgment of its initial
publication in this series.
3. Authors are permitted and encouraged to post their work online (e.g., in institutional repositories or on their website) prior to and
during the submission process, as it can lead to productive exchanges, as well as earlier and greater citation of published work (See
Open access policy for details).