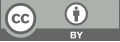
Research on Key Technologies, System Construction and Application Outlook of Big Data Governance
- 1 School of Software, Shandong University, Jinan, Shandong, China, 250000
* Author to whom correspondence should be addressed.
Abstract
In the era of digital transformation, big data has emerged as a pivotal resource driving innovation across various sectors. The effective utilization of big data, however,is fundamentally contingent upon robust governance frameworks. This paper conducts a comprehensive exploration of the multifaceted domain of big data governance, with a focus on three critical dimensions: key technological implementations, policy-system architecture, and application prospects. By employing a combination of literature review, case analysis, and comparative research methods, it addresses several key research questions, such as how to improve the efficiency and quality of data management in big data governance, how to construct a scientific and reasonable policy system, and how to better apply big data governance in different scenarios. The research findings demonstrate that advanced techniques, particularly data wrangling, play an indispensable role in data preprocessing and quality enhancement. Furthermore, the study reveals that a sophisticated, multi-tiered policy system involving multiple stakeholders is progressively evolving in the realm of policy-system construction. In terms of application, big data governance has achieved remarkable results in areas such as government decision-making and business operations, but challenges remain. Overall, this research provides valuable insights for promoting the development and application of big data governance.
Keywords
Big data governance, Data wrangling, Policy system, Application scenarios
[1]. Jian Y, Huaijie Z, Jianxing Y, et al. A panoramic framework for big data governance [J]. Big Data.,2020, 6 (1): 19 - 26.
[2]. Wenhong Z, Yahan Y, and Xiaofang X. Progress and Prospects of Building a Data Governance Policy System for Artificial Intelligence in China [J]. Library Forum,2025.
[3]. HELLERSTEIN J M, HEER J, KANDEL S. Self - service data preparation: research to practice [J]. IEEE Data Engineering Bulletin, 2018, 41 (2): 23 - 34.
[4]. HEER J, HELLERSTEIN J M, KANDEL S. Data wrangling [M]//Encyclopedia of big data technologies 2019. [S.l.:s.n.], 2019.
[5]. LI G L, ZHENG Y D, FAN J, et al. Crowdsourced data management: over view and challenges [C]//The 2017 ACM International Conference on Management of Data, May 14 - 19, 2017, Chicago, USA. New York: ACM Press,2017: 1711 - 1716.
[6]. DOAN A H, ARDALAN A, BALLARD J R, et al. Toward a system building agenda for data integration [J]. IEEE Data Engineering Bulletin, 2018, 41 (2): 35 - 46.
[7]. SONG X Y, WANG Y H. Data integration and application integration [M]. Beijing: China Water and Power Press, 2008.
[8]. ABEDJAN Z, CHU X, DENG D, et al. Detecting data errors: where are we and what needs to be done [J]. Proceedings of the VLDB Endowment, 2016, 9 (12): 993 - 1004.
[9]. BOHANNON P, FAN W F, GEERTS F, et al. Conditional functional dependencies for data cleaning [C]//2007 IEEE 23rd International Conference on Data Engineering, April 15 - 20, 2007, Istanbul, Turkey. Piscataway: IEEE Press, 2007: 746 - 755.
[10]. CHU X, ILYAS I F, PAPOTTI P. Holistic data cleaning: putting violations into context [C]//2013 IEEE 29th International Conference on Data Engineering (ICDE), April 8 - 12, 2013, Brisbane, Australia. Piscataway: IEEE Press, 2013: 458 - 469.
[11]. CHU X, MORCOS J, ILYAS I F, et al. KATARA: a data cleaning system powered by knowledge bases and crowdsourcing [C]//The 2015 ACM SIGMOD International Conference on Management of Data, May 31 - June 4, 2015, Melbourne, Australia. New York: ACM Press, 2015: 1247 - 1261.
[12]. YAKOUT M, BERTI - ÉQUILLE L, ELMAGARMID A K. Don’t be scared: use scalable automatic repairing with maximal likelihood and bounded changes [C]//The 2013 ACM SIGMOD International Conference on Management of Data, June 22 - 27, 2013, New York, USA. New York: ACM Press, 2013: 553 - 564.
[13]. REKATSINAS T, CHU X, ILYAS I F, et al. HoloClean: holistic data repairs with probabilistic inference [J]. Proceedings of the VLDB Endowment, 2017, 10 (11): 1190 - 1201.
Cite this article
Zhao,Z. (2025). Research on Key Technologies, System Construction and Application Outlook of Big Data Governance. Applied and Computational Engineering,146,60-66.
Data availability
The datasets used and/or analyzed during the current study will be available from the authors upon reasonable request.
Disclaimer/Publisher's Note
The statements, opinions and data contained in all publications are solely those of the individual author(s) and contributor(s) and not of EWA Publishing and/or the editor(s). EWA Publishing and/or the editor(s) disclaim responsibility for any injury to people or property resulting from any ideas, methods, instructions or products referred to in the content.
About volume
Volume title: Proceedings of SEML 2025 Symposium: Machine Learning Theory and Applications
© 2024 by the author(s). Licensee EWA Publishing, Oxford, UK. This article is an open access article distributed under the terms and
conditions of the Creative Commons Attribution (CC BY) license. Authors who
publish this series agree to the following terms:
1. Authors retain copyright and grant the series right of first publication with the work simultaneously licensed under a Creative Commons
Attribution License that allows others to share the work with an acknowledgment of the work's authorship and initial publication in this
series.
2. Authors are able to enter into separate, additional contractual arrangements for the non-exclusive distribution of the series's published
version of the work (e.g., post it to an institutional repository or publish it in a book), with an acknowledgment of its initial
publication in this series.
3. Authors are permitted and encouraged to post their work online (e.g., in institutional repositories or on their website) prior to and
during the submission process, as it can lead to productive exchanges, as well as earlier and greater citation of published work (See
Open access policy for details).