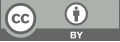
Tourism Resource Management and Allocation Strategies Based on Iterative Local Search and Sparrow Search Optimized Random Forest
- 1 College of Systems Engineering, National University of Defence Technology
- 2 College of Systems Engineering, National University of Defence Technology
- 3 College of Systems Engineering, National University of Defence Technology
* Author to whom correspondence should be addressed.
Abstract
In this study, an innovative prediction framework for intelligent management and allocation decisions of tourism resources is constructed by integrating the iterative local search strategy and the sparrow search algorithm to co-optimize the random forest model. The experimental results show that the tourism resource allocation level and the number of tourists show significant correlation (Pearson coefficient 0.73), and this indicator as a core driver directly affects the resource allocation decision; the resource utilization rate, as a secondary correlation variable (correlation coefficient 0.54), and the scenic spot operational efficiency form a two-way feedback mechanism, which together constitute the key influencing factors of the dynamic allocation of tourism resources. Through the quantitative analysis of the confusion matrix, the optimized model achieves 100% perfect fit in the training set, and maintains 95% prediction accuracy (190/200 samples are correctly classified) in the independent test set, and the performance degradation of only 5% fully proves the success of the algorithmic improvement strategy in avoiding overfitting, and its excellent generalization characteristic breaks through the limitations of the traditional prediction model in cross-scene applications.
Keywords
Iterative local search, Sparrow search, Random forests, Intelligent management of tourism resources
[1]. Huang, Arthur, et al. "When artificial intelligence meets the hospitality and tourism industry: an assessment framework to inform theory and management." Journal of Hospitality and Tourism Insights 5.5 (2022): 1080-1100.
[2]. Samara, Dimitra, Ioannis Magnisalis, and Vassilios Peristeras. "Artificial intelligence and big data in tourism: a systematic literature review." Journal of Hospitality and Tourism Technology 11.2 (2020): 343-367.
[3]. Knani, Mouna, Said Echchakoui, and Riadh Ladhari. "Artificial intelligence in tourism and hospitality: Bibliometric analysis and research agenda." International Journal of Hospitality Management 107 (2022): 103317.
[4]. Jabeen, Fauzia, Sameera Al Zaidi, and Maryam Hamad Al Dhaheri. "Automation and artificial intelligence in hospitality and tourism." Tourism Review 77.4 (2022): 1043-1061.
[5]. Filieri, Raffaele, et al. "Artificial intelligence (AI) for tourism: an European-based study on successful AI tourism start-ups." International Journal of Contemporary Hospitality Management 33.11 (2021): 4099-4125.
[6]. Doğan, Seden, and İlayda Zeynep Niyet. "Artificial intelligence (AI) in tourism." Future Tourism Trends Volume 2: Technology Advancement, Trends and Innovations for the Future in Tourism. Emerald Publishing Limited, 2024. 3-21.
[7]. Kırtıl, İsmail Gökay, and Volkan Aşkun. "Artificial intelligence in tourism: A review and bibliometrics research." Advances in Hospitality and Tourism Research (AHTR) 9.1 (2021): 205-233.
[8]. Tsaih, Rua-Huan, and Chih Chun Hsu. "Artificial intelligence in smart tourism: A conceptual framework." (2018).
[9]. Kazak, A. N., P. V. Chetyrbok, and N. N. Oleinikov. "Artificial intelligence in the tourism sphere." IOP Conference Series: Earth and Environmental Science. Vol. 421. No. 4. IOP Publishing, 2020.
[10]. Patrichi, Ioana Cristiana. "AI solutions for sustainable tourism management: a comprehensive review." Journal of Information Systems & Operations Management 18.1 (2024): 172-185.
Cite this article
Wei,Y.;Xie,Z.;Ge,B. (2025). Tourism Resource Management and Allocation Strategies Based on Iterative Local Search and Sparrow Search Optimized Random Forest. Applied and Computational Engineering,146,67-75.
Data availability
The datasets used and/or analyzed during the current study will be available from the authors upon reasonable request.
Disclaimer/Publisher's Note
The statements, opinions and data contained in all publications are solely those of the individual author(s) and contributor(s) and not of EWA Publishing and/or the editor(s). EWA Publishing and/or the editor(s) disclaim responsibility for any injury to people or property resulting from any ideas, methods, instructions or products referred to in the content.
About volume
Volume title: Proceedings of SEML 2025 Symposium: Machine Learning Theory and Applications
© 2024 by the author(s). Licensee EWA Publishing, Oxford, UK. This article is an open access article distributed under the terms and
conditions of the Creative Commons Attribution (CC BY) license. Authors who
publish this series agree to the following terms:
1. Authors retain copyright and grant the series right of first publication with the work simultaneously licensed under a Creative Commons
Attribution License that allows others to share the work with an acknowledgment of the work's authorship and initial publication in this
series.
2. Authors are able to enter into separate, additional contractual arrangements for the non-exclusive distribution of the series's published
version of the work (e.g., post it to an institutional repository or publish it in a book), with an acknowledgment of its initial
publication in this series.
3. Authors are permitted and encouraged to post their work online (e.g., in institutional repositories or on their website) prior to and
during the submission process, as it can lead to productive exchanges, as well as earlier and greater citation of published work (See
Open access policy for details).