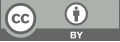
Research on the algorithm of image feature detection and matching
- 1 College of Landscape Achitecture, NanJing Forestry University, Nanjing, China
* Author to whom correspondence should be addressed.
Abstract
In-depth research on feature detection technology affects people's modern life. Modern artificial intelligence can act as the eyes of human beings and efficiently filter out effective information from complex pictures. Corner detection has now evolved into a tool for efficient image scanning. People's increasingly stringent requirements for image processing continue to promote the birth of new technologies. Corner detection methods have been improved and perfected, and have experienced detectors such as Harris, FAST, Scalriant Feature Transform (SIFT), Speeded Up Robust Feature (SURF), Binary Robust Invariant Scalable Keypoints (BRISK), KAZE, AKAZE and Oriented FAST and Rotated BRIEF (ORB). In the face of many mainstream detectors, the main research purpose of this paper is to rely on Python and Computer vision to study the detection efficiency of various detectors in different environments. In this experiment, thirteen groups of pictures were selected, and after being flipped, complicated, and blurred respectively, they were detected by different detectors to obtain the results. Finally, by comparing the feature detection points, detection time and other factors, this study found that the ORB detector can be competent in most situations and is currently the fastest and stable feature point detection and extraction algorithm. On the other hand, the BRISK detector can handle highly blurred images more efficiently.
Keywords
Computer vision, feature detection and matching
[1]. Senit_Co. (2017). Harris corner detection of image features. Senitco.github.io. https://senitco. github.io/2017/06/18/image-feature-harris/
[2]. CharlesWu123. (2021). OpenCV —— SIFT feature detector for feature point detection. CSDN. https://blog.csdn.net/m0_38007695/article/details/115524748
[3]. Bansal, Kumar, M., & Kumar, M. (2021). 2D object recognition: a comparative analysis of SIFT, SURF and ORB feature descriptors. Multi. Tools Appl., 80(12), 18839–18857.
[4]. SongpingWang. (2021). OpenCV + CPP Series (22) Image Feature Matching (KAZE/AKAZE). Electro. Des. Eng., 438
[5]. Dellinger, Delon, J., Gousseau, Y., Michel, J., & Tupin, F. (2015). SAR-SIFT: A SIFT-Like Algorithm for SAR Images. IEEE Trans. Geos. Re. Sens., 53(1), 453–466.
[6]. OpenCV school. (2019). Detailed explanation and use of OpenCV SIFT feature algorithm. cloud.tencent. https://cloud.tencent.com/developer/article/1419617
[7]. Alex777. (2019). ORB Feature Extraction Algorithm (Theory). Cnblogs. https://www.cnblogs. com/alexme/p/11345701.html
[8]. Matusiak, Skulimowski, P., & Strumillo, P. (2017). Unbiased evaluation of keypoint detectors with respect to rotation invariance. IET Comput. Vis., 11(7), 507–516.
[9]. SongpingWang. (2021). OpenCV + CPP Series (22) Image Feature Matching (KAZE/AKAZE). CSDN. https://blog.csdn.net/wsp_1138886114/article/details/119772358
[10]. Hujingshuang. (2015). BRISK Feature Extraction Algorithm. CSDN. https://blog.csdn.net/ hujingshuang/article/details/47045497
Cite this article
Zhang,J. (2023). Research on the algorithm of image feature detection and matching. Applied and Computational Engineering,5,527-535.
Data availability
The datasets used and/or analyzed during the current study will be available from the authors upon reasonable request.
Disclaimer/Publisher's Note
The statements, opinions and data contained in all publications are solely those of the individual author(s) and contributor(s) and not of EWA Publishing and/or the editor(s). EWA Publishing and/or the editor(s) disclaim responsibility for any injury to people or property resulting from any ideas, methods, instructions or products referred to in the content.
About volume
Volume title: Proceedings of the 3rd International Conference on Signal Processing and Machine Learning
© 2024 by the author(s). Licensee EWA Publishing, Oxford, UK. This article is an open access article distributed under the terms and
conditions of the Creative Commons Attribution (CC BY) license. Authors who
publish this series agree to the following terms:
1. Authors retain copyright and grant the series right of first publication with the work simultaneously licensed under a Creative Commons
Attribution License that allows others to share the work with an acknowledgment of the work's authorship and initial publication in this
series.
2. Authors are able to enter into separate, additional contractual arrangements for the non-exclusive distribution of the series's published
version of the work (e.g., post it to an institutional repository or publish it in a book), with an acknowledgment of its initial
publication in this series.
3. Authors are permitted and encouraged to post their work online (e.g., in institutional repositories or on their website) prior to and
during the submission process, as it can lead to productive exchanges, as well as earlier and greater citation of published work (See
Open access policy for details).