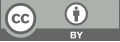
Comparing one-stage and two-stage learning strategy in object detection
- 1 Tianjin No.21 High School, Tianjin, 300400, China
- 2 The Affiliated High School of Fuzhou Institute of Education, Fuzhou, Fujian, 350108 China
- 3 International Department, The Affiliated High School of SCNU, Guangzhou, Guangdong, 510000, China
* Author to whom correspondence should be addressed.
Abstract
Object detection plays a vital role in computer social perception and computer vision. It could be applied to computer navigation, video monitoring, industrial detection, and so on. It greatly reduces the human labours by automatically locate and identify objects. Nowadays, the mainstream methods of object detection could be separated into the one- and two-stage method. The one-stage method leverages Convolutional Neural Network (CNN) for obtaining features and directly locate the target objects and their corresponding category probabilities. Different from the two-stage solutions, its accuracy is lower and the recognition speed is higher. The two-stage method is a straight forward solution, which process is mainly completed through a complete CNN, so CNN features will be leveraged to extract the feature description of the target among candidate regions through a CNN. The accuracy of the two-step method has been greatly improved, but the running speed is much slower than the one-step method. While the one-step method is less accurate, it is much faster. In this work, representative works for object detection are conducted and compared. The results could further demonstrate their respective advantages.
Keywords
Object Detection, Deep Learning, One-stage Learning, Two-stage Learning.
[1]. Redmon J, Divvala S, Girshick R, et al., 2016, You only look once: Unified, real-time object detection. In: Proceedings of the IEEE conference on computer vision and pattern recognition. pp: 779-788.
[2]. Redmon J, Farhadi A., 2017, YOLO9000: better, faster, stronger, In: Proceedings of the IEEE conference on computer vision and pattern recognition. pp 7263-7271.
[3]. Redmon J, Farhadi A., 2018, Yolov3: An incremental improvement. arXiv preprint arXiv:1804.02767.
[4]. Bochkovskiy A, Wang C Y, Liao H Y M., 2020, Yolov4: Optimal speed and accuracy of object detection. arXiv preprint arXiv:2004.10934.
[5]. Ge Z, Liu S, Wang F, et al., 2021, Yolox: Exceeding yolo series in 2021[J]. arXiv preprint arXiv:2107.08430.
[6]. Lin T Y, Goyal P, Girshick R, et al., 2017, Focal loss for dense object detection. In: Proceedings of the IEEE international conference on computer vision. pp 2980-2988.
[7]. Law H, Deng J., 2018, Cornernet: Detecting objects as paired keypoints. In: Proceedings of the European conference on computer vision, pp 734-750.
[8]. Duan K, Bai S, Xie L, et al., 2019, Centernet: Keypoint triplets for object detection. In: Proceedings of the IEEE/CVF international conference on computer vision. pp 6569-6578.
[9]. Tan M, Pang R, Le Q V., 2020, Efficientdet: Scalable and efficient object detection, In: Proceedings of the IEEE/CVF conference on computer vision and pattern recognition. pp 10781-10790.
[10]. Girshick R, Donahue J, Darrell T, et al., 2014, Rich feature hierarchies for accurate object detection and semantic segmentation. In: Proceedings of the IEEE conference on computer vision and pattern recognition. pp 580-587.
[11]. Ren S, He K, Girshick R, et al., 2015, Faster r-cnn: Towards real-time object detection with region proposal networks. In: Advances in neural information processing systems, 28.
Cite this article
Bi,H.;Wen,V.;Xu,Z. (2023). Comparing one-stage and two-stage learning strategy in object detection. Applied and Computational Engineering,5,171-177.
Data availability
The datasets used and/or analyzed during the current study will be available from the authors upon reasonable request.
Disclaimer/Publisher's Note
The statements, opinions and data contained in all publications are solely those of the individual author(s) and contributor(s) and not of EWA Publishing and/or the editor(s). EWA Publishing and/or the editor(s) disclaim responsibility for any injury to people or property resulting from any ideas, methods, instructions or products referred to in the content.
About volume
Volume title: Proceedings of the 3rd International Conference on Signal Processing and Machine Learning
© 2024 by the author(s). Licensee EWA Publishing, Oxford, UK. This article is an open access article distributed under the terms and
conditions of the Creative Commons Attribution (CC BY) license. Authors who
publish this series agree to the following terms:
1. Authors retain copyright and grant the series right of first publication with the work simultaneously licensed under a Creative Commons
Attribution License that allows others to share the work with an acknowledgment of the work's authorship and initial publication in this
series.
2. Authors are able to enter into separate, additional contractual arrangements for the non-exclusive distribution of the series's published
version of the work (e.g., post it to an institutional repository or publish it in a book), with an acknowledgment of its initial
publication in this series.
3. Authors are permitted and encouraged to post their work online (e.g., in institutional repositories or on their website) prior to and
during the submission process, as it can lead to productive exchanges, as well as earlier and greater citation of published work (See
Open access policy for details).