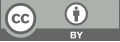
A Critical Review on CT Image denoising in Wavelet domain
- 1 Graphic Era Deemed to be University
- 2 Bennett University
- 3 University of Warwick
- 4 Jeonbuk National University
- 5 Uttaranchal University
- 6 Amity University Uttar Pradesh
* Author to whom correspondence should be addressed.
Abstract
Medical images are commonly used today by medical practitioners for the purposes of diagnosis. For the purposes of diagnosis, diagnostic images are widely used today by medical professionals. In general, MRI works on soft tissues, and CT works on hard tissues. Due to device and hardware limitations, mathematical calculations, transition mechanisms in computers, there are chances of creating noise in medical images.In yhis paper, a critical review on CT image denoising has been performed in wavelet domain. In the transform domain, the process of removing noise from an image starts with the image or data being divided up into a representation in scale space. It has methods for setting thresholds, rules for shrinking, and a way to clean up noise based on wavelets, among other things.
Keywords
CT image denoising, wavelet transform, entropy, thresholding, PSNR, SSIM
[1]. Goldstein T, Osher S (2009) The split bregman method for l1 regularized problems. SIAM J Imaging Sci 2(2):323–34
[2]. Elad M, Aharon M (2006) Image denoising via sparse and redundant representations over learned dictionaries. IEEE Trans Image Process 15(12):3736–45
[3]. Mustafa ZA, Kadah YM (2011) Multi resolution bilateral filter for MR image denoising. In: Proceeding on 1st Middle East conference on biomedical engineering (MECBME). Sharjah, pp 180–184
[4]. Borsdorf A, Raupach R, Flohr T, Hornegger J (2008) Wavelet based noise reduction in CT-images using correlation analysis. IEEE Trans Med Imaging 27(12):1685–1703
[5]. Rabbani H, Nezafat R, Gazor S (2009) Wavelet-domain medical image denoising using bivariate laplacian mixture model. IEEE Trans Biomed Eng 56(12):2826–2837
[6]. Blu, T., & Luisier, F. (2007). The SURE-LET approach to image denoising. IEEE Transactions on Image Processing, 16(11), 2778-2786.
[7]. Chen, M., Pu, Y. F., & Bai, Y. C. (2020). Low-dose CT image denoising using residual convolutional network with fractional TV loss. Neurocomputing.
[8]. Trung, N. T., Hoan, T. D., Trung, N. L., & Luong, M. (2020). Low-dose CT image denoising using image decomposition and sparse representation. REV Journal on Electronics and Communications, 9(3-4).
[9]. Li, Z., Zhou, S., Huang, J., Yu, L., & Jin, M. (2020). Investigation of low-dose CT image denoising using unpaired deep learning methods. IEEE Transactions on Radiation and Plasma Medical Sciences.
[10]. Kumar, B. S. (2013). Image denoising based on non-local means filter and its method noise thresholding. Signal, image and video processing, 7(6), 1211-1227.
[11]. Diwakar, M., Kumar, P., & Singh, A. K. (2020). CT image denoising using NLM and its method noise thresholding. Multimedia Tools and Applications, 79(21), 14449-14464.
[12]. Diwakar, M., Singh, P., Swarup, C., Bajal, E., Jindal, M., Ravi, V., Singh, K.U. and Singh, T., 2022. Noise Suppression and Edge Preservation for Low-Dose COVID-19 CT Images Using NLM and Method Noise Thresholding in Shearlet Domain. Diagnostics, 12(11), p.2766.
Cite this article
Diwakar,M.;Singh,P.;Shankar,A.;E.,S.V.;Joshi,K.;Kumar,M. (2023). A Critical Review on CT Image denoising in Wavelet domain. Applied and Computational Engineering,8,31-36.
Data availability
The datasets used and/or analyzed during the current study will be available from the authors upon reasonable request.
Disclaimer/Publisher's Note
The statements, opinions and data contained in all publications are solely those of the individual author(s) and contributor(s) and not of EWA Publishing and/or the editor(s). EWA Publishing and/or the editor(s) disclaim responsibility for any injury to people or property resulting from any ideas, methods, instructions or products referred to in the content.
About volume
Volume title: Proceedings of the 2023 International Conference on Software Engineering and Machine Learning
© 2024 by the author(s). Licensee EWA Publishing, Oxford, UK. This article is an open access article distributed under the terms and
conditions of the Creative Commons Attribution (CC BY) license. Authors who
publish this series agree to the following terms:
1. Authors retain copyright and grant the series right of first publication with the work simultaneously licensed under a Creative Commons
Attribution License that allows others to share the work with an acknowledgment of the work's authorship and initial publication in this
series.
2. Authors are able to enter into separate, additional contractual arrangements for the non-exclusive distribution of the series's published
version of the work (e.g., post it to an institutional repository or publish it in a book), with an acknowledgment of its initial
publication in this series.
3. Authors are permitted and encouraged to post their work online (e.g., in institutional repositories or on their website) prior to and
during the submission process, as it can lead to productive exchanges, as well as earlier and greater citation of published work (See
Open access policy for details).