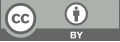
Emotion Recognition Based on the Human's Brain
- 1 University of Nottingham Malaysia
* Author to whom correspondence should be addressed.
Abstract
There are several different algorithms to train models in machine learning and for purpose of enhancing the accuracy of the models for emotion recognition systems by using EEG datasets, we can compare different algorithms and methods. To experience more intuitively the different impacts caused by different algorithms and methods, ‘Emotion classification by applying ‘Convolutional Neural Networks, Sparse Auto-encoder, Deep Neural Network’, and ‘ZTW-based epoch selection algorithm’, these three models of emotion recognition will be mentioned and compared in this essay to find out a way to improve the accuracy of the emotion recognition models based on EEG datasets, which also include the process and final results of these models.
Keywords
emotion recognition, EEG, human Brain-based, artificial intelligence, machine learning
[1]. Psychology is a little spiritual. (2022, July 12). What's killing nearly 100 million people with depression? The 2022 National Blue Book on Depression. _ Ifeng.com. https://i.ifeng.com/c/8Hb7dhuxkoz
[2]. Dolan, R. J. (2002, November 8). Emotion, Cognition, and Behavior. Science, 298(5596), 1191–1194.
[3]. Nicu Sebe (The Netherlands), Ira Cohen (USA), and Thomas S. Huang (USA)Handbook of Pattern Recognition and Computer Vision, MULTIMODAL EMOTION RECOGNITION 3rd. January 2005, 387-409
[4]. Hu, W., Huang, G., Li, L., Zhang, L., Zhang, Z., & Liang, Z. (2020, September).Video‐triggered EEG‐emotion public databases and current methods: A survey. Brain Science Advances, 6(3), 255–287.
[5]. NHS. (2022, January 5). Electroencephalogram (EEG). NHS choices. https://www.nhs.uk/conditions/electroencephalogram/
[6]. Sanei, S., & Chambers, J. A. (2022). Chapter 4 Fundamentals of EEG Signal Processing. In EEG signal processing and machine learning (pp. 77–113). essay, Wiley.
[7]. Topic, A., Russo, M., Stella, M., & Saric, M. (2022a, April 23). Emotion Recognition Using a Reduced Set of EEG Channels Based on Holographic Feature Maps. Sensors, 22(9), 3248.
[8]. Liu, J., Wu, G., Luo, Y., Qiu, S., Yang, S., Li, W., & Bi, Y. (2020). EEG-based emotion classification using a deep neural network and sparse autoencoder. Frontiers in Systems Neuroscience, 14, 43. https://www.frontiersin.org/articles/10.3389/fnsys.2020.00043/full
[9]. Gannouni, S., Aledaily, A., Belwafi, K., & Aboalsamh, H. (2021). Emotion detection using electroencephalography signals and a zero-time windowing-based epoch estimation and relevant electrode identification. Scientific Reports, 11. https://doi.org/10.1038/s41598-021-86345-5
Cite this article
Tang,L. (2023). Emotion Recognition Based on the Human's Brain. Applied and Computational Engineering,8,7-12.
Data availability
The datasets used and/or analyzed during the current study will be available from the authors upon reasonable request.
Disclaimer/Publisher's Note
The statements, opinions and data contained in all publications are solely those of the individual author(s) and contributor(s) and not of EWA Publishing and/or the editor(s). EWA Publishing and/or the editor(s) disclaim responsibility for any injury to people or property resulting from any ideas, methods, instructions or products referred to in the content.
About volume
Volume title: Proceedings of the 2023 International Conference on Software Engineering and Machine Learning
© 2024 by the author(s). Licensee EWA Publishing, Oxford, UK. This article is an open access article distributed under the terms and
conditions of the Creative Commons Attribution (CC BY) license. Authors who
publish this series agree to the following terms:
1. Authors retain copyright and grant the series right of first publication with the work simultaneously licensed under a Creative Commons
Attribution License that allows others to share the work with an acknowledgment of the work's authorship and initial publication in this
series.
2. Authors are able to enter into separate, additional contractual arrangements for the non-exclusive distribution of the series's published
version of the work (e.g., post it to an institutional repository or publish it in a book), with an acknowledgment of its initial
publication in this series.
3. Authors are permitted and encouraged to post their work online (e.g., in institutional repositories or on their website) prior to and
during the submission process, as it can lead to productive exchanges, as well as earlier and greater citation of published work (See
Open access policy for details).