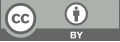
Network resilience: impact on small-world network
- 1 Lanzhou University
* Author to whom correspondence should be addressed.
Abstract
Lots of complex systems in the real world have network structures, and a number of these structures have small-world property. This kind of structures are called small-world networks. Examples include the world's air transportation system, electric power systems, and human functional brain network, and small-world property is one of the key reasons why these systems function efficiently. However, for complex systems, in addition to their efficiency, resilience or robustness is also one of the concerns, as these systems need to ensure that they do not completely collapse on their own in case of failure of a small number of their components. The purpose of this paper is to try to find and explain the factors that affect the robustness of small-world network by comparing different classes of small-world networks and analysing differences between them and possible causes of these differences, in order to get an idea to optimize the robustness of small-world networks while preserving their small-world property.
Keywords
small-world network, robustness, broad-scale network, single-scale network.
[1]. Van Leeuwen, J. (Ed.). (1991). Handbook of theoretical computer science (vol. A) algorithms and complexity. Mit Press.
[2]. Bollobás, B. (1998). Random graphs. In Modern graph theory (pp. 215-252). Springer, New York, NY.
[3]. Amaral, L. A. N., Scala, A., Barthelemy, M., & Stanley, H. E. (2000). Classes of small-world networks. Proceedings of the national academy of sciences, 97(21), 11149-11152.
[4]. Cohen, R., Erez, K., Ben-Avraham, D., & Havlin, S. (2000). Resilience of the internet to random breakdowns. Physical review letters, 85(21), 4626.
[5]. Cohen, R., & Havlin, S. (2003). Scale-free networks are ultrasmall. Physical review letters, 90(5), 058701.
[6]. Barabási, A. L., Albert, R., & Jeong, H. (1999). Mean-field theory for scale-free random networks. Physica A: Statistical Mechanics and its Applications, 272(1-2), 173-187.
[7]. Albert, R., Jeong, H., & Barabási, A. L. (2000). Error and attack tolerance of complex networks. nature, 406(6794), 378-382.
[8]. Barabási, A. L., & Albert, R. (1999). Emergence of scaling in random networks. science, 286(5439), 509-512.
[9]. Dorogovtsev, S. N., & Mendes, J. F. F. (2000). Evolution of networks with aging of sites. Physical Review E, 62(2), 1842.
[10]. Jeong, H., Néda, Z., & Barabási, A. L. (2003). Measuring preferential attachment in evolving networks. EPL (Europhysics Letters), 61(4), 567.
[11]. Joyce, K. E., Hayasaka, S., &Laurienti, P. J. (2013). The human functional brain network demonstrates structural and dynamical resilience to targeted attack. PLoS computational biology, 9(1), e1002885.
[12]. Barabási, A. L. (2013). Network science. Philosophical Transactions of the Royal Society A: Mathematical, Physical and Engineering Sciences, 371(1987), 20120375.
[13]. Cohen, R., Erez, K., Ben-Avraham, D., & Havlin, S. (2001). Breakdown of the internet under intentional attack. Physical review letters, 86(16), 3682.
[14]. Yuan, X., Shao, S., Stanley, H. E., & Havlin, S. (2015). How breadth of degree distribution influences network robustness: comparing localized and random attacks. Physical Review E, 92(3), 032122.
[15]. Paul, G., Tanizawa, T., Havlin, S., & Stanley, H. E. (2004). Optimization of robustness of complex networks. The European Physical Journal B, 38(2), 187-191.
Cite this article
Wang,C. (2023). Network resilience: impact on small-world network. Applied and Computational Engineering,6,1329-1335.
Data availability
The datasets used and/or analyzed during the current study will be available from the authors upon reasonable request.
Disclaimer/Publisher's Note
The statements, opinions and data contained in all publications are solely those of the individual author(s) and contributor(s) and not of EWA Publishing and/or the editor(s). EWA Publishing and/or the editor(s) disclaim responsibility for any injury to people or property resulting from any ideas, methods, instructions or products referred to in the content.
About volume
Volume title: Proceedings of the 3rd International Conference on Signal Processing and Machine Learning
© 2024 by the author(s). Licensee EWA Publishing, Oxford, UK. This article is an open access article distributed under the terms and
conditions of the Creative Commons Attribution (CC BY) license. Authors who
publish this series agree to the following terms:
1. Authors retain copyright and grant the series right of first publication with the work simultaneously licensed under a Creative Commons
Attribution License that allows others to share the work with an acknowledgment of the work's authorship and initial publication in this
series.
2. Authors are able to enter into separate, additional contractual arrangements for the non-exclusive distribution of the series's published
version of the work (e.g., post it to an institutional repository or publish it in a book), with an acknowledgment of its initial
publication in this series.
3. Authors are permitted and encouraged to post their work online (e.g., in institutional repositories or on their website) prior to and
during the submission process, as it can lead to productive exchanges, as well as earlier and greater citation of published work (See
Open access policy for details).