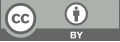
Finding the best opening in chess with multi-armed bandit algorithm
- 1 University of Virgina
* Author to whom correspondence should be addressed.
Abstract
With many gaming AI being developed and being able to defeat top human players in recent years, AI has once again become the hottest topic in research and even in our daily life. This paper also researches on gaming AI and chess. Instead of using deep learning and the Monte Carlo Search algorithm, this paper focuses on the opening only with multi-armed bandit algorithms to find the best moves and opening. Specifically, the method used in this paper is epsilon greedy and Thompson sampling. The dataset used in this paper is from Kaggle. This paper considers each move as a set of choices one needs to make and considers the big picture as a multi-armed bandit problem. This paper aims to develop a relative best strategy to counter those opening or make changes to a disadvantaged situation.
Keywords
multi-armed bandit, chess, epsilon greedy, Thompson sampling
[1]. Yajie Wang, Bingzhi Qi, Yunbo Zhang, Aodong Din. Research on Texas Hold’em Poker Algorithm Based on Expected Revenueand UCT Algorithm. Journal of Chongqing University of Technology (Natural Science), pp.167-168 (2016).
[2]. LECUNY, BENGIOY, HINTONG: Deep learning. Nature, pp.436 (2015).
[3]. Yajie Wang, Bingzhi Qi, Yunbo Zhang, Aodong Ding: Application of Improved UCT Algorithm Combined with Neural Network in Checkers. Journal of Chongqing University of Technology (Natural Science), (07), pp.260 (2021).
[4]. Demis Hassabis: Artificial Intelligence: Chess match of the century, Nature, 04, pp.1 (2017).
[5]. Tor Lattimore and Csaba Szepesv ́ari, Bandit Algorithm, Cambridge University Press, pp.7-8 (2020).
[6]. Alexandre Gilotte, Clément Calauzènes, Thomas Nedelec, Alexandre Abraham, Simon Dollé: Offline A/B testing for Recommender Systems, ACM, pp.203-204 (2018).
[7]. Will Dabney, Georg Ostrovski, Andre ́Barreto: Temporally-Extended ε-Greedy Exploration, arxiv, pp.4 (2020).
[8]. Tor Lattimore and Csaba Szepesv ́ari, Bandit Algorithm, Cambridge University Press, pp.459-461 (2020).
[9]. Agrawal, S. & Goyal, N.: Analysis of Thompson Sampling for the Multi-armed Bandit Problem. Mlr, pp.3-4 (2012).
[10]. Huan Gui, Ya Xu, Anmol Bhasin, J. Han.: Network A/B Testing: From Sampling to Estimation, ACM, pp.399-400 (2015).
Cite this article
Lin,Y. (2023). Finding the best opening in chess with multi-armed bandit algorithm. Applied and Computational Engineering,13,21-28.
Data availability
The datasets used and/or analyzed during the current study will be available from the authors upon reasonable request.
Disclaimer/Publisher's Note
The statements, opinions and data contained in all publications are solely those of the individual author(s) and contributor(s) and not of EWA Publishing and/or the editor(s). EWA Publishing and/or the editor(s) disclaim responsibility for any injury to people or property resulting from any ideas, methods, instructions or products referred to in the content.
About volume
Volume title: Proceedings of the 5th International Conference on Computing and Data Science
© 2024 by the author(s). Licensee EWA Publishing, Oxford, UK. This article is an open access article distributed under the terms and
conditions of the Creative Commons Attribution (CC BY) license. Authors who
publish this series agree to the following terms:
1. Authors retain copyright and grant the series right of first publication with the work simultaneously licensed under a Creative Commons
Attribution License that allows others to share the work with an acknowledgment of the work's authorship and initial publication in this
series.
2. Authors are able to enter into separate, additional contractual arrangements for the non-exclusive distribution of the series's published
version of the work (e.g., post it to an institutional repository or publish it in a book), with an acknowledgment of its initial
publication in this series.
3. Authors are permitted and encouraged to post their work online (e.g., in institutional repositories or on their website) prior to and
during the submission process, as it can lead to productive exchanges, as well as earlier and greater citation of published work (See
Open access policy for details).