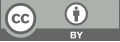
Empirical performances comparison for ETC algorithm
- 1 University of Nottingham
* Author to whom correspondence should be addressed.
Abstract
Explore-then-commit (ETC) algorithm is a widely used algorithm in bandit problems, which are used to identify the optimal choice among a series of choices that yield random outcomes. The ETC algorithm is adapted from A/B testing, a popular procedure in decision-making process. This paper explores the multi-armed bandit problem and some related algorithms to tackle the multi-armed bandit problem. In particular, this paper focuses on the explore-then-commit (ETC) algorithm, a simple algorithm that has an exploration phase, and then commits the best action. To evaluate the performance of ETC, a variety of settings is made in the experiment, such as the number of arms and input parameter m, i.e., how many times each arm is pulled in the exploration phase. The result shows that the average cumulative regret increases when the number of arms gets larger. With the increase of parameter m, the cumulative regret decreases in the beginning, until reaching the minimum value, and then starts increasing. The purpose of this paper is to empirically evaluate the performance of the ETC algorithm and investigate the relationships between the parameter settings and the overall performance of the algorithm.
Keywords
multi-armed bandit, stochastic bernoulli bandit, explore-then-commit algorithm
[1]. Lattimore, T. and Szepesvári, C.: Bandit algorithms. Cambridge, United Kingdom: Cambridge University Press, (2020).
[2]. Slivkins, A.: Introduction to multi-armed bandits. Boston: Now, (2019).
[3]. Garivier, A., Kaufmann, E., Lattimore, T., On Explore-Then-Commit Strategies. Advances in Neural Information Processing Systems 29, (2016).
[4]. T. Jin, P. Xu, X. Xiao, and Q. Gu, “Double explore-then-commit: Asymptotic optimality and beyond,” in Conference on Learning Theory, PMLR, July, pp. 2584-2633 (2021).
[5]. V. Kuleshov and D. Precup, “Algorithms for multi-armed bandit problems,” arXiv preprint arXiv:1402.6028, 2014.
[6]. H. Gui, Y. Xu, A. Bhasin, and J. Han, “Network a/b testing: From sampling to estimation,” in Proceedings of the 24th International Conference on World Wide Web, pp. 399–409, 2015.
[7]. E. Kaufmann, O. Cappe ́, and A. Garivier, “On the complexity of a/b testing,” in Conference on Learning Theory, pp. 461–481, PMLR, 2014.
[8]. Y. Xu, N. Chen, A. Fernandez, O. Sinno, and A. Bhasin, “From infrastructure to culture: A/b testing challenges in large scale social networks,” in Proceedings of the 21th ACM SIGKDD International Conference on Knowledge Discovery and Data Mining, pp. 2227–2236, 2015.
[9]. S. W. Young, “Improving library user experience with a/b testing: Principles and process,” Weave: Journal of Library User Experience, vol. 1, no. 1, 2014.
[10]. A. Gilotte, C. Calauzenes, T. Nedelec, A. Abraham, and S. Dolle, “Offline a/b testing for recommender systems,” in Proceedings of the Eleventh ACM International Conference on Web Search and Data Mining, pp. 198–206, 2018.
Cite this article
Chen,T. (2023). Empirical performances comparison for ETC algorithm. Applied and Computational Engineering,13,29-36.
Data availability
The datasets used and/or analyzed during the current study will be available from the authors upon reasonable request.
Disclaimer/Publisher's Note
The statements, opinions and data contained in all publications are solely those of the individual author(s) and contributor(s) and not of EWA Publishing and/or the editor(s). EWA Publishing and/or the editor(s) disclaim responsibility for any injury to people or property resulting from any ideas, methods, instructions or products referred to in the content.
About volume
Volume title: Proceedings of the 5th International Conference on Computing and Data Science
© 2024 by the author(s). Licensee EWA Publishing, Oxford, UK. This article is an open access article distributed under the terms and
conditions of the Creative Commons Attribution (CC BY) license. Authors who
publish this series agree to the following terms:
1. Authors retain copyright and grant the series right of first publication with the work simultaneously licensed under a Creative Commons
Attribution License that allows others to share the work with an acknowledgment of the work's authorship and initial publication in this
series.
2. Authors are able to enter into separate, additional contractual arrangements for the non-exclusive distribution of the series's published
version of the work (e.g., post it to an institutional repository or publish it in a book), with an acknowledgment of its initial
publication in this series.
3. Authors are permitted and encouraged to post their work online (e.g., in institutional repositories or on their website) prior to and
during the submission process, as it can lead to productive exchanges, as well as earlier and greater citation of published work (See
Open access policy for details).