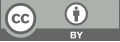
The study and analysis related to the evolution of the knowledge tracing research
- 1 Hefei University of Technology
* Author to whom correspondence should be addressed.
Abstract
In the field of education, the level of awareness of students' knowledge status largely affects students' learning efficiency. Knowledge Tracing, as a method of modeling students' learning status, obtaining their knowledge status to predict their future learning can provide more targeted learning solutions, which is extremely useful for improving students' learning efficiency and implementing tailor-made education. This paper introduces the related research methods and their core ideas in the order of probability-based models, logistic function-based models, and deep learning-based models by investigating the development of this field since its introduction. Among the probability-based models, the mainstream methods include BKT and DBKT, which are based on Hidden Markov Models with certain assumptions and are not ideal in practical application scenarios. In contrast, logistic-based models consider a variety of factors in the student learning process and have achieved good results. After that, deep learning-based model has achieved excellent assessment results through its powerful feature extraction ability, and many variants have been derived. This paper introduces DKT in detail and briefly introduces its variant models. At last, this paper summarizes some of the mainstream evaluation criteria in this field and gives the widely used datasets and their categories. Finally, this paper proposes some suggestions for the future development of the field in view of the shortcomings of the current research status for reference.
Keywords
knowledge tracing, BKT, LFA, DKT
[1]. Corbett A T Anderson J R 1994 User modeling and user-adapted interaction (Modeling the acquisition of procedural knowledge) p 253-278
[2]. Abdelrahman G Wang Q Nunes B 2023 Knowledge Tracing: A Survey (ACM Computing Surveys) p 1-37
[3]. Liu Q Shen S Huang Z et al 2021 A survey of knowledge tracing (arXiv preprint arXiv:210515106)
[4]. Käser T Klingler S Schwing a G Gross M 2017 Dynamic Bayesian networks for student modelling (IEEE Transactions on Learning Technologies) p 450-462
[5]. Cen H Koedinger K & Junker B 2006 Learning factors analysis–a general method for cognitive model evaluation and improvement (Intelligent Tutoring Systems: 8th International Conference) p 164-175
[6]. Pavlik Jr P I Cen H & Koedinger K R 2009 Performance Factors Analysis--A New Alternative to Knowledge Tracing (Online Submission)
[7]. Vie J J & Kashima H 2019 Knowledge tracing machines: Factorization machines for knowledge tracing (Proceedings of the AAAI Conference on Artificial Intelligence) p 750-757
[8]. Piech C Bassen J Huang J Ganguli S Sahami M Guibas L J & Sohl-Dickstein J 2015 Deep knowledge tracing (Advances in neural information processing systems)
[9]. [Pandey S Karypis G 2019 A self-attentive model for knowledge tracing (arXiv preprint arXiv:190706837)
[10]. Dalianis H 2018 Evaluation metrics and evaluation Clinical text mining: secondary use of electronic patient records 45-53
Cite this article
Bai,Y. (2023). The study and analysis related to the evolution of the knowledge tracing research. Applied and Computational Engineering,16,171-179.
Data availability
The datasets used and/or analyzed during the current study will be available from the authors upon reasonable request.
Disclaimer/Publisher's Note
The statements, opinions and data contained in all publications are solely those of the individual author(s) and contributor(s) and not of EWA Publishing and/or the editor(s). EWA Publishing and/or the editor(s) disclaim responsibility for any injury to people or property resulting from any ideas, methods, instructions or products referred to in the content.
About volume
Volume title: Proceedings of the 5th International Conference on Computing and Data Science
© 2024 by the author(s). Licensee EWA Publishing, Oxford, UK. This article is an open access article distributed under the terms and
conditions of the Creative Commons Attribution (CC BY) license. Authors who
publish this series agree to the following terms:
1. Authors retain copyright and grant the series right of first publication with the work simultaneously licensed under a Creative Commons
Attribution License that allows others to share the work with an acknowledgment of the work's authorship and initial publication in this
series.
2. Authors are able to enter into separate, additional contractual arrangements for the non-exclusive distribution of the series's published
version of the work (e.g., post it to an institutional repository or publish it in a book), with an acknowledgment of its initial
publication in this series.
3. Authors are permitted and encouraged to post their work online (e.g., in institutional repositories or on their website) prior to and
during the submission process, as it can lead to productive exchanges, as well as earlier and greater citation of published work (See
Open access policy for details).