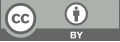
Deep learning-based artificial intelligence imaging probes for Alzheimer’s disease
- 1 Shanghai Pinghe School
* Author to whom correspondence should be addressed.
Abstract
Brain medical imaging is a main diagnosis method for Alzheimer’s disease (AD). But the method relies on the physician’s manual analysis which is subjective and time consuming. In recent years, artificial intelligence (AI) technology has been widely applied in clinical diagnosis. This thesis is about the deep learning model to be designed to realize the computer-aided diagnosis of medical images. A model of densely connected network (DenseNet) as an AI technology, automatically learns the semantic features related to AD diagnosis on the brain MRI images from ADNI data. At the same time, for solving the limited medical image samples problem, the effective transfer learning technology was applied in the experiment. The final model result achieves 90.8% accuracy, 82.2% sensitivity and 96.1% specificity on the diagnostic task of AD, and the diagnostic accuracy is better than prevailing methods. Besides 80.4% accuracy, 52.2% sensitivity, and 84.8% specificity are achieved in the task of distinguishing progressive from stable MCI patients. This method can provide more accurate diagnosis results of Alzheimer’s disease expected for the clinical early auxiliary diagnosis.
Keywords
AI-artificial intelligence, densely connected network, transfer learning, AD-Alzheimer’s disease, medical imaging
[1]. Frisoni, Giovanni B., et al. 2010. “The clinical use of structural MRI in Alzheimer disease.” Nature Reviews Neurology 6.2: 67-77.
[2]. Li Qingfeng, Xing Xiaodan, Feng Qianjin. 2020. The magnetic resonance diagnosis method of Alzheimer’s disease based on the coupled convolution-graph convolutional neural network [J]. Journal of Southern Medical University, 40 (4): 7.
[3]. Zhang, Jun, Mingxia Liu, and Dinggang Shen. 2019. “Detecting anatomical landmarks from limited medical imaging data using two-stage task-oriented deep neural networks.” IEEE Transactions on Image Processing 26.10: 4753-4764.
[4]. Yang, Jiancheng, Rui Shi, and Bingbing Ni. 2021. “Medmnist classification decathlon: A lightweight automl benchmark for medical image analysis.” 2021 IEEE 18th International Symposium on Biomedical Imaging (ISBI). IEEE.
[5]. Jack Jr, Clifford R., et al. 2008. “The Alzheimer’s disease neuroimaging initiative (ADNI): MRI methods.” Journal of Magnetic Resonance Imaging: An Official Journal of the International Society for Magnetic Resonance in Medicine 27.4: 685-691.
[6]. Chapman K R, Bing-Canar H, Alosco M L, et al. 2016. Mini Mental State Examination and Logical Memory scores for entry into Alzheimer’s disease trials [J]. Alzheimer’s research & therapy, 8(1): 1-11.
[7]. O’Bryant , Sid E., et al. 2010. “Validation of the new interpretive guidelines for the clinical dementia rating scale sum of boxes score in the national Alzheimer’s coordinating center database.” Archives of neurology 67.6: 746-749.
[8]. Liu, Mingxia, et al. 2018. “Joint classification and regression via deep multi-task multi-channel learning for Alzheimer’s disease diagnosis.” IEEE Transactions on Biomedical Engineering 66.5: 1195-1206.
[9]. Holmes, Colin J., et al. 1998. “Enhancement of MR images using registration for signal averaging.” Journal of computer assisted tomography 22.2: 324-333.
[10]. Huang G, Liu Z, Laurens V, et al. 2016. Densely Connected Convolutional Networks [C]// IEEE Computer Society. IEEE Computer Society.
Cite this article
Ji,Y. (2023). Deep learning-based artificial intelligence imaging probes for Alzheimer’s disease. Applied and Computational Engineering,17,1-9.
Data availability
The datasets used and/or analyzed during the current study will be available from the authors upon reasonable request.
Disclaimer/Publisher's Note
The statements, opinions and data contained in all publications are solely those of the individual author(s) and contributor(s) and not of EWA Publishing and/or the editor(s). EWA Publishing and/or the editor(s) disclaim responsibility for any injury to people or property resulting from any ideas, methods, instructions or products referred to in the content.
About volume
Volume title: Proceedings of the 5th International Conference on Computing and Data Science
© 2024 by the author(s). Licensee EWA Publishing, Oxford, UK. This article is an open access article distributed under the terms and
conditions of the Creative Commons Attribution (CC BY) license. Authors who
publish this series agree to the following terms:
1. Authors retain copyright and grant the series right of first publication with the work simultaneously licensed under a Creative Commons
Attribution License that allows others to share the work with an acknowledgment of the work's authorship and initial publication in this
series.
2. Authors are able to enter into separate, additional contractual arrangements for the non-exclusive distribution of the series's published
version of the work (e.g., post it to an institutional repository or publish it in a book), with an acknowledgment of its initial
publication in this series.
3. Authors are permitted and encouraged to post their work online (e.g., in institutional repositories or on their website) prior to and
during the submission process, as it can lead to productive exchanges, as well as earlier and greater citation of published work (See
Open access policy for details).