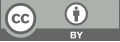
Research on methods and applications of question answering system in the context of ChatGPT
- 1 Wen-Zhou Kean University
* Author to whom correspondence should be addressed.
Abstract
In the 21st century, there has been a growing importance placed on the "body" of artificial intelligence, particularly as it relates to language processing. Researchers have developed various machine learning models with a focus on language understanding, including Large Language Model (LLM), Bidirectional Encoder Representation from Transformers (BERT), and Natural Language Processing (NLP). These models have led to the development of numerous applications, such as ChatGPT-3.5, which has recently gained widespread attention. In addition to ChatGPT, other applications have also benefited from these language processing models, including Question Answering Systems (QAS). This paper will examine three QAS that have been enhanced by the context of ChatGPT, discuss the relevant applications, and analyze these different applications in order to predict future trends in this field. One notable QAS is OpenAI's GPT-3-powered AI that can answer questions about any topic. This application leverages the capabilities of GPT-3 to provide accurate and informative responses to a wide range of questions. Another QAS is IBM's Watson, which utilizes natural language processing and machine learning algorithms to understand and respond to user queries. Watson has been used in various industries, including healthcare, finance, and retail. A third QAS is Google's BERT-based system, which uses pre-trained language models to improve its responses to user queries. This system has been integrated into Google Search and other products, allowing users to receive more precise and relevant search results. Overall, the development of these QAS and other language processing applications marks an exciting period of progress in the field of artificial intelligence. As researchers continue to refine these models and explore new applications, we can expect to see even more advanced and sophisticated language processing systems emerge in the future.
Keywords
Q&A system, NLP, deep learning, application, Ai
[1]. Lu Jing, Wu Chunlei, & Wang Leiqaun. (2021). Visual question answering System based on symmetrical attention mechanism. Computer System Application. http://www.c-s-a.org.cn/1003-3254/7925.html
[2]. Wu Ziming, Yang Fangyu, Liang Jun, & Lei Jianbo. (2023). Questions and Answers to Chinese diseases based on Model fusion method Research on text matching method. Health Information Management in China.
[3]. Zhang Kailiang & Chen Yun. (2022). Intelligent voice question answering system based on deep learning. China Computer & Communication.
[4]. Xu Chengwei, Jia Xiaoxia, & Ran Qingyun. (2023). Application of intelligent question answering system in equipment manufacturing industry. Manufacturing Automation.
[5]. Lu Zhiliang, Kuang Xianping, & Zeng Zhaojin. (2021). Research on Power Customer Service System based on natural Language processing. Internet and Security.
[6]. Guo Li. (2023). Application research of intelligent question answering system in campus Intelligence Service. Light Industry Science and Technology.
[7]. Gu Xinyun, Wan Yan, Jiang Runzhe, Zhang Yan, & Hu Cuihua. (2022). The Interactive research of network Teaching based on intelligent Question answering System. Computer Knowledge and Technology.
[8]. Omar, R., Mangukiya, O., Kalnis, P., et al. (2023). ChatGPT versus traditional question answering for knowledge graphs: Current status and future directions towards knowledge graph chatbots. arXiv preprint arXiv:2302.06466.
[9]. Tan, Y., Min, D., Li, Y., et al. (2023). Evaluation of ChatGPT as a Question Answering System for Answering Complex Questions. arXiv preprint arXiv:2303.07992.
[10]. Gilson, A., Safranek, C. W., Huang, T., et al. (2023). How does CHATGPT perform on the United States Medical Licensing Examination? The implications of large language models for medical education and knowledge assessment. JMIR Medical Education, 9(1), e45312.
Cite this article
Li,X. (2023). Research on methods and applications of question answering system in the context of ChatGPT. Applied and Computational Engineering,18,60-65.
Data availability
The datasets used and/or analyzed during the current study will be available from the authors upon reasonable request.
Disclaimer/Publisher's Note
The statements, opinions and data contained in all publications are solely those of the individual author(s) and contributor(s) and not of EWA Publishing and/or the editor(s). EWA Publishing and/or the editor(s) disclaim responsibility for any injury to people or property resulting from any ideas, methods, instructions or products referred to in the content.
About volume
Volume title: Proceedings of the 5th International Conference on Computing and Data Science
© 2024 by the author(s). Licensee EWA Publishing, Oxford, UK. This article is an open access article distributed under the terms and
conditions of the Creative Commons Attribution (CC BY) license. Authors who
publish this series agree to the following terms:
1. Authors retain copyright and grant the series right of first publication with the work simultaneously licensed under a Creative Commons
Attribution License that allows others to share the work with an acknowledgment of the work's authorship and initial publication in this
series.
2. Authors are able to enter into separate, additional contractual arrangements for the non-exclusive distribution of the series's published
version of the work (e.g., post it to an institutional repository or publish it in a book), with an acknowledgment of its initial
publication in this series.
3. Authors are permitted and encouraged to post their work online (e.g., in institutional repositories or on their website) prior to and
during the submission process, as it can lead to productive exchanges, as well as earlier and greater citation of published work (See
Open access policy for details).