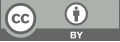
Optimized federated learning based on Adagrad algorithm and algorithm optimization
- 1 Xi’an University of Posts & Telecommunications
- 2 Xi’an University of Posts & Telecommunications
- 3 Xiamen University Malaysia
* Author to whom correspondence should be addressed.
Abstract
Federated learning allows you to train machine learning models without sharing your local data. Due to the No-iid problem, this paper is based on the Moon algorithm, which can have excellent performance in datasets of images with models that use deep learning and outperforms FedAvg, FedProx, and other algorithms, with the goal to decrease communication costs while enhancing efficiency more effectively. This study optimizes its gradient descent technique based on Moon's algorithm by utilizing Adaptive Gradient (AdaGrad) optimizer and combining with knowledge distillation to improve Moon's algorithm in order to better reduce communication costs and improve efficiency. That is, it reduces the loss and improves the accuracy faster and better in local training. In this paper, we experimentally show that the optimized moon can better solve the communication cost and improve the accuracy rate.
Keywords
AdaGrad, moon, federated learning, algorithm optimization
[1]. T. Zhang, L. Gao, C. He, M. Zhang, B. Krishnamachari, and A. S. Avestimehr, “Federated Learning for the Internet of Things: Applications, Challenges, and Opportunities,” IEEE Internet of Things Magazine, vol. 5, no. 1, pp. 24–29, Mar. 2022.
[2]. T. Li, A. K. Sahu, A. Talwalkar, and V. Smith, “Federated Learning: Challenges, Methods, and Future Directions,” IEEE Signal Processing Magazine, vol. 37, no. 3, pp. 50–60, May 2020.
[3]. E. by: P. Kairouz and H. B. McMahan, “Advances and Open Problems in Federated Learning,” Foundations and Trends® in Machine Learning, vol. 14, no. 1, 2021.
[4]. L. Fu, H. Zhang, G. Gao, H. Wang, M. Zhang, and X. Liu, “Client Selection in Federated Learning: Principles, Challenges, and Opportunities,” arXiv:2211.01549 [cs], Nov. 2022, Accessed: May 02, 2023. [Online].
[5]. X. Li, K. Huang, W. Yang, S. Wang, and Z. Zhang, “On the Convergence of FedAvg on Non-IID Data,” arXiv:1907.02189 [cs, math, stat], Jun. 2020.
[6]. Q. Li, B. He, and D. Song, “Model-Contrastive Federated Learning,” Computer Vision and Pattern Recognition, Jun. 2021.
[7]. T. Chen, S. Kornblith, M. Norouzi, and G. Hinton, “A Simple Framework for Contrastive Learning of Visual Representations,” arXiv:2002.05709 [cs, stat], Jun. 2020.
[8]. K. Sohn, “Improved Deep Metric Learning with Multi-class N-pair Loss Objective,” Neural Information Processing Systems, 2016.
[9]. G. Hinton, O. Vinyals, and J. Dean, “Distilling the Knowledge in a Neural Network,” arXiv:1503.02531 [cs, stat], Mar. 2015.
[10]. DuchiJohn, HazanElad, and SingerYoram, “Adaptive Subgradient Methods for Online Learning and Stochastic Optimization,” Journal of Machine Learning Research, Jul. 2011.
[11]. J. Zhang, S. Guo, X. Ma, H. Wang, W. Xu, and F. Wu, “Parameterized Knowledge Transfer for Personalized Federated Learning,” arXiv:2111.02862 [cs], Nov. 2021, Accessed: May 02, 2023. [Online].
Cite this article
Chu,E.;Li,D.;Tong,Y. (2023). Optimized federated learning based on Adagrad algorithm and algorithm optimization. Applied and Computational Engineering,19,9-17.
Data availability
The datasets used and/or analyzed during the current study will be available from the authors upon reasonable request.
Disclaimer/Publisher's Note
The statements, opinions and data contained in all publications are solely those of the individual author(s) and contributor(s) and not of EWA Publishing and/or the editor(s). EWA Publishing and/or the editor(s) disclaim responsibility for any injury to people or property resulting from any ideas, methods, instructions or products referred to in the content.
About volume
Volume title: Proceedings of the 5th International Conference on Computing and Data Science
© 2024 by the author(s). Licensee EWA Publishing, Oxford, UK. This article is an open access article distributed under the terms and
conditions of the Creative Commons Attribution (CC BY) license. Authors who
publish this series agree to the following terms:
1. Authors retain copyright and grant the series right of first publication with the work simultaneously licensed under a Creative Commons
Attribution License that allows others to share the work with an acknowledgment of the work's authorship and initial publication in this
series.
2. Authors are able to enter into separate, additional contractual arrangements for the non-exclusive distribution of the series's published
version of the work (e.g., post it to an institutional repository or publish it in a book), with an acknowledgment of its initial
publication in this series.
3. Authors are permitted and encouraged to post their work online (e.g., in institutional repositories or on their website) prior to and
during the submission process, as it can lead to productive exchanges, as well as earlier and greater citation of published work (See
Open access policy for details).