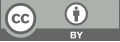
Intelligent optical transceiver technology based on federated learning traffic prediction
- 1 Beijing University of Posts and Telecommunications
* Author to whom correspondence should be addressed.
Abstract
With the development of optical network, modern optical network needs better performance. Because the traditional optical transceiver technology has a delay according to the flow switching transmission configuration, the delay optical network service still adopts the original configuration transmission, so a certain degree of frequency spectrum resources waste and high blocking rate will be caused. The above situation can be improved if the transmission configuration can be deployed in advance based on the predicted traffic. Federated learning is a scheme of distributed training model, which can train the traffic prediction model in distributed way under the premise of ensuring the privacy of client data, which is very suitable for the traffic prediction of optical network terminals. This paper proposes an intelligent optical transceiver technology based on federal learning traffic prediction, applies the federal learning on the traffic prediction of optical communication network terminal, distributed training traffic prediction model, and deploy the optical transceiver early transmission configuration such as modulation format and baud rate parameters, thus to weaken the delay of optical transceiver technology, reduce the network blocking rate and improve the transmission performance of optical network.
Keywords
federated learning, traffic prediction, optical transceiver technology
[1]. B. McMahan, E. Moore, D. Ramage, S. Hampson, and B. A. y Arcas, “Communication-Efficient Learning of Deep Networks from Decentralized Data,” PMLR, vol. 54, pp. 1273–1282, Apr. 2017.
[2]. S. Lin et al., “Wireless communication traffic prediction based on federated learning,” Computer application, vol. 0, Sep. 2022.
[3]. K. Yonenaga et al., “Bit-rate-flexible all-optical OFDM transceiver using variable multi-carrier source and DQPSK/DPSK mixed multiplexing,” IEEE Xplore, Mar. 2009.
[4]. S. Li, “Research on Optical Network Traffic Analysis and Intelligent Optical Transceiver Technology Based on Machine Learning - CNKI,”kns.cnki.net.
[5]. Q. Yang, Y. Liu, T. Chen, and Y. Tong, “Federated Machine Learning,” ACM Transactions on Intelligent Systems and Technology, vol. 10, no. 2, pp. 1–19, Feb. 2019.
[6]. J. K. Fischer et al., “Bandwidth-Variable Transceivers based on Four-Dimensional Modulation Formats,” Journal of Lightwave Technology, vol. 32, no. 16, pp. 2886–2895, Aug. 2014.
[7]. R. H. Shumway and D. S. Stoffer, Time Series Analysis and Its Applications. Cham: Springer International Publishing, 2017.
[8]. I. Rojas et al., “Soft-computing techniques and ARMA model for time series prediction,” Neurocomputing, vol. 71, no. 4–6, pp. 519–537, Jan. 2008.
[9]. J. T. Connor, R. D. Martin, and L. E. Atlas, “Recurrent neural networks and robust time series prediction,” IEEE Transactions on Neural Networks, vol. 5, no. 2, pp. 240–254, Mar. 1994.
[10]. S. Hochreiter and J. Schmidhuber, “Long Short-Term Memory,” Neural Computation, vol. 9, no. 8, pp. 1735–1780, Nov. 1997.
[11]. F. Tang, Z. Md. Fadlullah, B. Mao, and N. Kato, “An Intelligent Traffic Load Prediction-Based Adaptive Channel Assignment Algorithm in SDN-IoT: A Deep Learning Approach,” IEEE Internet of Things Journal, vol. 5, no. 6, pp. 5141–5154, Dec. 2018.
Cite this article
Zhang,Y. (2023). Intelligent optical transceiver technology based on federated learning traffic prediction. Applied and Computational Engineering,19,37-43.
Data availability
The datasets used and/or analyzed during the current study will be available from the authors upon reasonable request.
Disclaimer/Publisher's Note
The statements, opinions and data contained in all publications are solely those of the individual author(s) and contributor(s) and not of EWA Publishing and/or the editor(s). EWA Publishing and/or the editor(s) disclaim responsibility for any injury to people or property resulting from any ideas, methods, instructions or products referred to in the content.
About volume
Volume title: Proceedings of the 5th International Conference on Computing and Data Science
© 2024 by the author(s). Licensee EWA Publishing, Oxford, UK. This article is an open access article distributed under the terms and
conditions of the Creative Commons Attribution (CC BY) license. Authors who
publish this series agree to the following terms:
1. Authors retain copyright and grant the series right of first publication with the work simultaneously licensed under a Creative Commons
Attribution License that allows others to share the work with an acknowledgment of the work's authorship and initial publication in this
series.
2. Authors are able to enter into separate, additional contractual arrangements for the non-exclusive distribution of the series's published
version of the work (e.g., post it to an institutional repository or publish it in a book), with an acknowledgment of its initial
publication in this series.
3. Authors are permitted and encouraged to post their work online (e.g., in institutional repositories or on their website) prior to and
during the submission process, as it can lead to productive exchanges, as well as earlier and greater citation of published work (See
Open access policy for details).