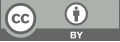
IOT and Deep Learning Assisted Smart Agriculture System for Plant Disease Identification
- 1 Dept. of Computer Science and Design, Kongu Engineering College, Perundurai, Tamilna-du-638060
- 2 Dept. of Information Technology, Kongu Engineering College, Perundurai, Tamilnadu-638060
- 3 Dept. of Computer Science and Design, Kongu Engineering College, Perundurai, Tamilna-du-638060
- 4 Dept. of Computer Science and Engineering, Kongu Engineering College, Perundurai, Tamilnadu-638060
* Author to whom correspondence should be addressed.
Abstract
Agriculture employed more than 50% of labours and contributed nearly 18% to country's GDP. India is the leading producers of Many crops, such as wheat, rice, lentils, cotton, peanuts and perishable products but in recent years, the percentage of people getting into agriculture has been reduced. Crop disease and incorrect maintenance of humidity and moisture is the major reason that makes new farmers frustrated. Developing a deep learning model, that can identify the crop disease with high accuracy, and using this model one can easily identify the crop disease which makes easier for them treat the crop with pesticide, so that we can’t wait for the experts. Using the IOT architecture to find the humidity and soil moisture of the field so that the farmer can maintain the correct level of humidity and soil moisture through irrigation, sometimes extreme high and very low moisture and humidity leads to diseases. An enhanced classification model was suggested in this paper to classify leaf diseases. Dataset with more than 10000 images is used for image classification. Classification accuracy is achieved using AlexNet model and it came out to be the highest in comparison to other models, and IOT part using the Raspberry pi 3 kit with soil moisture and humidity and soil moisture sensor.
Keywords
Convolutional Neural Network, Internet of Things, AlexNet.
[1]. Liu, Y., Sathishkumar, V. E., & Manickam, A. (2022). Augmented reality technology based on school physical education training. Computers and Electrical Engineering, 99, 107807.
[2]. Schalkoff, R.J., Artificial neural networks. 1997: McGraw-Hill Higher Education.
[3]. Rajalaxmi, R. R., Narasimha Prasad, L. V., Janakiramaiah, B., Pavankumar, C. S., Neelima, N., & Sathishkumar, V. E. (2022). Optimizing Hyperparameters and Performance Analysis of LSTM Model in Detecting Fake News on Social media. Transactions on Asian and Low-Resource Language Information Processing.
[4]. Prema K, Carmel Mary Belinda, “Smart Farming: IoT Based Plant Leaf Disease Detection and Prediction using Deep Neural Network with Image Processing”, International Journal of Innovative Technology and Exploring Engineering (IJITEE) ISSN: 2278-3075, Volume-8 Issue-9, July, 2019
[5]. Chauhan, Prachi, et al. "Plant diseases concept in smart agriculture using deep learning." Smart agricultural services using deep learning, big data, and IoT. IGI Global, 2021. 139-153.
[6]. Ale, Laha & Sheta, Alaa & Li, Longzhuang & Wang, Ye & Zhang, Ning. “Deep Learning Based Plant Disease Detection for Smart Agriculture”. 1-6. 10.1109/GCWkshps45667.2019.9024439, 2019.
[7]. Arathi Nair, Gouripriya J, Merry James, Sumi Mary Shibu, Shihabudeen H, “Smart Farming and Plant Disease Detection using IoT and ML, International Journal of Engineering Research & Technology (Ijert) Ncreis – 2021 (Volume 09 – Issue 13), 2021.
[8]. Yan Guo, Jin Zhang, Chengxin Yin, Xiaonan Hu, Yu Zou, Zhipeng Xue, Wei Wang, "Plant Disease Identification Based on Deep Learning Algorithm in Smart Farming", Discrete Dynamics in Nature and Society, vol. 2020, Article ID 2479172, 11 pages, 2020. https://doi.org/10.1155/2020/2479172
[9]. S., Sathiya, Antony, Cecil and Ghodke, Praveen Kumar. "Plant disease identification using IoT and deep learning algorithms". Artificial Intelligence for Signal Processing and Wireless Communication, edited by Abhinav Sharma, Arpit Jain, Ashwini Kumar Arya and Mangey Ram, Berlin, Boston: De Gruyter, 2022, pp. 11-46. https://doi.org/10.1515/9783110734652-002
[10]. Babu, J. C., Kumar, M. S., Jayagopal, P., Sathishkumar, V. E., Rajendran, S., Kumar, S., ... & Mahseena, A. M. (2022). IoT-Based Intelligent System for Internal Crack Detection in Building Blocks. Journal of Nanomaterials, 2022.
[11]. Easwaramoorthy, S., Sophia, F., & Prathik, A. (2016, February). Biometric Authentication using finger nails. In 2016 international conference on emerging trends in engineering, technology and science (ICETETS) (pp. 1-6). IEEE.
[12]. Seth, I., S.N. Panda, and K. Guleria. IoT based Smart Applications and Recent Research Trends. in 2021 6th International Conference on Signal Processing, Computing and Control (ISPCC). 2021. IEEE.
[13]. Selvaraj, S., R. Thangarajan, and M. Saravanan, Trust-Based and Optimized RPL Routing in Social Internet of Things Network, in Mobile computing and sustainable informatics. 2022, Springer. p. 513-529.
[14]. Sathishkumar V E, Changsun Shin, Youngyun Cho, “Efficient energy consumption prediction model for a data analytic-enabled industry building in a smart city”, Building Research & Information, Vol. 49. no. 1, pp. 127-143, 2021.
[15]. Sathishkumar V E, Youngyun Cho, “A rule-based model for Seoul Bike sharing demand prediction using Weather data”, European Journal of Remote Sensing, Vol. 52, no. 1, pp. 166-183, 2020.
[16]. Sathishkumar V E, Jangwoo Park, Youngyun Cho, “Seoul Bike Trip duration prediction using data mining techniques”, IET Intelligent Transport Systems, Vol. 14, no. 11, pp. 1465-1474, 2020.
[17]. Sathishkumar V E, Jangwoo Park, Youngyun Cho, “Using data mining techniques for bike sharing demand prediction in Metropolitan city”, Computer Communications, Vol. 153, pp. 353-366, 2020.
[18]. Sathishkumar V E, Yongyun Cho, “Season wise bike sharing demand analysis using random forest algorithm”, Computational Intelligence, pp. 1-26, 2020.
[19]. Sathishkumar, V. E., Wesam Atef Hatamleh, Abeer Ali Alnuaim, Mohamed Abdelhady, B. Venkatesh, and S. Santhoshkumar. "Secure Dynamic Group Data Sharing in Semi-trusted Third Party Cloud Environment." Arabian Journal for Science and Engineering (2021): 1-9.
[20]. Chen, J., Shi, W., Wang, X., Pandian, S., & Sathishkumar, V. E. (2021). Workforce optimisation for improving customer experience in urban transportation using heuristic mathematical model. International Journal of Shipping and Transport Logistics, 13(5), 538-553.
[21]. Pavithra, E., Janakiramaiah, B., Narasimha Prasad, L. V., Deepa, D., Jayapandian, N., & Sathishkumar, V. E., Visiting Indian Hospitals Before, During and After Covid. International Journal of Uncertainty, Fuzziness and Knowledge-Based Systems, 30 (1), 111-123, 2022.
[22]. Easwaramoorthy, S., Moorthy, U., Kumar, C. A., Bhushan, S. B., & Sadagopan, V. (2017, January). Content based image retrieval with enhanced privacy in cloud using apache spark. In International Conference on Data Science Analytics and Applications (pp. 114-128). Springer, Singapore.
[23]. Sathishkumar, V. E., Agrawal, P., Park, J., & Cho, Y. (2020, April). Bike Sharing Demand Prediction Using Multiheaded Convolution Neural Networks. In Basic & Clinical Pharmacology & Toxicology (Vol. 126, pp. 264-265). 111 RIVER ST, HOBOKEN 07030-5774, NJ USA: WILEY.
[24]. Subramanian, M., Shanmuga Vadivel, K., Hatamleh, W. A., Alnuaim, A. A., Abdelhady, M., & VE, S. (2021). The role of contemporary digital tools and technologies in Covid‐19 crisis: An exploratory analysis. Expert systems.
[25]. Sathishkumar, V. E., Rahman, A. B. M., Park, J., Shin, C., & Cho, Y. (2020, April). Using machine learning algorithms for fruit disease classification. In Basic & clinical pharmacology & toxicology (Vol. 126, pp. 253-253). 111 RIVER ST, HOBOKEN 07030-5774, NJ USA: WILEY.
[26]. Sathishkumar, V. E., & Cho, Y. (2019, December). Cardiovascular disease analysis and risk assessment using correlation based intelligent system. In Basic & clinical pharmacology & toxicology (Vol. 125, pp. 61-61). 111 RIVER ST, HOBOKEN 07030-5774, NJ USA: WILEY.
Cite this article
S.,S.;R.,T.;C.,S.;K.,V.R.T. (2023). IOT and Deep Learning Assisted Smart Agriculture System for Plant Disease Identification. Applied and Computational Engineering,2,717-726.
Data availability
The datasets used and/or analyzed during the current study will be available from the authors upon reasonable request.
Disclaimer/Publisher's Note
The statements, opinions and data contained in all publications are solely those of the individual author(s) and contributor(s) and not of EWA Publishing and/or the editor(s). EWA Publishing and/or the editor(s) disclaim responsibility for any injury to people or property resulting from any ideas, methods, instructions or products referred to in the content.
About volume
Volume title: Proceedings of the 4th International Conference on Computing and Data Science (CONF-CDS 2022)
© 2024 by the author(s). Licensee EWA Publishing, Oxford, UK. This article is an open access article distributed under the terms and
conditions of the Creative Commons Attribution (CC BY) license. Authors who
publish this series agree to the following terms:
1. Authors retain copyright and grant the series right of first publication with the work simultaneously licensed under a Creative Commons
Attribution License that allows others to share the work with an acknowledgment of the work's authorship and initial publication in this
series.
2. Authors are able to enter into separate, additional contractual arrangements for the non-exclusive distribution of the series's published
version of the work (e.g., post it to an institutional repository or publish it in a book), with an acknowledgment of its initial
publication in this series.
3. Authors are permitted and encouraged to post their work online (e.g., in institutional repositories or on their website) prior to and
during the submission process, as it can lead to productive exchanges, as well as earlier and greater citation of published work (See
Open access policy for details).