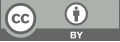
Machine learning-based DDoS detection for IoT networks
- 1 University College London
* Author to whom correspondence should be addressed.
Abstract
DDoS attacks are one of the most dangerous threats to IoT networks, and they involve using attacker-controlled botnets to flood the network with malicious traffic that denies legitimate services. The global DDoS landscape is rapidly evolving, and it has become increasingly important for devices to quickly identify the types of DDoS attacks they face so that they can choose and implement effective countermeasures against known attacks. Machine learning has emerged as a popular approach for detecting DDoS traffic in IoT networks. This paper implements four machine learning models, namely Support Vector Machine (SVM), Decision Tree, Long Short-Term Memory (LSTM), and Random Forest, to perform multiclass classification for DDoS attack detection. The study uses the CICDDoS2019 dataset for evaluation. The results show that all four models can detect most types of DDoS traffic effectively. The Random Forest model achieves the highest overall accuracy of 99.32%, followed by the Decision Tree model with an accuracy of 99.10%. The LSTM and SVM models have slightly lower accuracies at 98.20% and 93.00%, respectively. The study also evaluates the models' performance in terms of precision, recall, and F1 score. Decision Tree outperforms the other models in precision, while Random Forest has the highest recall score. Moreover, the Random Forest model performs the best in terms of the F1 score. In conclusion, this paper demonstrates the effectiveness of machine learning-based approaches for DDoS detection in IoT networks using four popular models. The results illustrate the potential for these models to provide reliable and accurate detection of DDoS traffic, thus enabling effective countermeasures to be taken against this type of attack.
Keywords
DDos, classifier, SVM, decision tree, LSTM, random forest
[1]. Iot-Analytics. (2022). Iot 2021 in review: The 10 most relevant iot developments of the year. https://iot-analytics.com/iot-2021-in-review/
[2]. Wei, W., Yang, A. T., Shi, W., et al. (2016). Security in internet of things: Opportunities and challenges. In 2016 International Conference on Identification, Information and Knowledge in the Internet of Things (IIKI) (pp. 512-518). IEEE.
[3]. Kolias, C., Kambourakis, G., Stavrou, A., et al. (2017). DDoS in the IoT: Mirai and Other Botnets. Computer, 50(7), 80-84.
[4]. Yoachimik. (2023). Cloudflare DDoS Threat Report for 2022 Q4. Cloudflare. https://blog.cloudflare.com/ddos-threat-report-2022-q4/
[5]. Microsoft Security. (2023). 2022 in review: DDOS attack trends and insights. https://www.microsoft.com/en-us/security/blog/2023/02/21/2022-in-review-ddos-attack-trends-and-insights/
[6]. Sharafaldin, I., Lashkari, A. H., Hakak, S., et al. (2019). Developing Realistic Distributed Denial of Service (DDoS) Attack Dataset and Taxonomy. In 2019 International Carnahan Conference on Security Technology (ICCST) (pp. 1-8). IEEE.
[7]. Kumari, P., & Jain, A. K. (2023). A comprehensive study of ddos attacks over IOT network and their countermeasures. Computers & Security, 127, 103096.
[8]. Vishwakarma, R., & Jain, A. K. (2019). A survey of ddos attacking techniques and defence mechanisms in the IOT network. Telecommunication Systems, 73(1), 3-25.
[9]. Suresh, M., & Anitha, R. (2011). Evaluating machine learning algorithms for detecting ddos attacks. In Advances in Network Security and Applications (pp. 441-452). Springer, Berlin, Heidelberg.
[10]. Doshi, R., Apthorpe, N., & Feamster, N. (2018). Machine Learning DDoS Detection for Consumer Internet of Things Devices. In 2018 IEEE Security and Privacy Workshops (SPW) (pp. 29-35). IEEE.
[11]. Tuan, T. A., Long, H. V., Son, L. H., et al. (2020). Performance evaluation of botnet ddos attack detection using machine learning. Evolutionary Intelligence, 13(2), 283-294.
[12]. Chen, Y.-W., Sheu, J.-P., Kuo, Y.-C., et al. (2020). Design and Implementation of IoT DDoS Attacks Detection System based on Machine Learning. In 2020 European Conference on Networks and Communications (EuCNC) (pp. 122-127). IEEE.
[13]. Gaur, V., & Kumar, R. (2021). Analysis of machine learning classifiers for early detection of ddos attacks on IOT devices. Arabian Journal for Science and Engineering, 47(2), 1353-1374.
[14]. Raschka S, Mirjalili V. Python machine learning: Machine learning and deep learning with Python, scikit-learn, and TensorFlow 2[M]. Packt Publishing Ltd, 2019.
[15]. Alves F R V, Vieira R P M. The Newton fractal’s Leonardo sequence study with the Google Colab[J]. International Electronic Journal of Mathematics Education, 2019, 15(2): em0575.
Cite this article
Xie,Y. (2023). Machine learning-based DDoS detection for IoT networks. Applied and Computational Engineering,29,99-107.
Data availability
The datasets used and/or analyzed during the current study will be available from the authors upon reasonable request.
Disclaimer/Publisher's Note
The statements, opinions and data contained in all publications are solely those of the individual author(s) and contributor(s) and not of EWA Publishing and/or the editor(s). EWA Publishing and/or the editor(s) disclaim responsibility for any injury to people or property resulting from any ideas, methods, instructions or products referred to in the content.
About volume
Volume title: Proceedings of the 5th International Conference on Computing and Data Science
© 2024 by the author(s). Licensee EWA Publishing, Oxford, UK. This article is an open access article distributed under the terms and
conditions of the Creative Commons Attribution (CC BY) license. Authors who
publish this series agree to the following terms:
1. Authors retain copyright and grant the series right of first publication with the work simultaneously licensed under a Creative Commons
Attribution License that allows others to share the work with an acknowledgment of the work's authorship and initial publication in this
series.
2. Authors are able to enter into separate, additional contractual arrangements for the non-exclusive distribution of the series's published
version of the work (e.g., post it to an institutional repository or publish it in a book), with an acknowledgment of its initial
publication in this series.
3. Authors are permitted and encouraged to post their work online (e.g., in institutional repositories or on their website) prior to and
during the submission process, as it can lead to productive exchanges, as well as earlier and greater citation of published work (See
Open access policy for details).