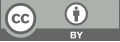
Comparative analysis of machine learning techniques for cryptocurrency price prediction
- 1 SWUFE-UD Institute of Data Science, Southwestern University of Finance, Chengdu, Sichuan, 611130, China.
* Author to whom correspondence should be addressed.
Abstract
The emergence of cryptocurrencies has revolutionized the concept of digital currencies and attracted significant attention from financial markets. Predicting the price dynamics of cryptocurrencies is crucial but challenging due to their highly volatile and non-linear nature. This study compares the performance of various models in predicting cryptocurrency prices using three datasets: Bitcoin (BTC), Litecoin (LTC), and Ethereum (ETH). The models analyzed include Moving Average (MA), Logistic Regression (LR), Autoregressive Integrated Moving Average (ARIMA), Long Short-Term Memory (LSTM), and Convolutional Neural Network-Long Short-Term Memory (CNN-LSTM). The objective is to uncover underlying patterns in cryptocurrency price movements and identify the most accurate and reliable approach for predicting future prices. Through the analysis, it could be observed that MA, LR, and ARIMA models struggle to capture the actual trend accurately. In contrast, LSTM and CNN-LSTM models demonstrate strong fit to the actual price trend, with CNN-LSTM exhibiting a higher level of granularity in its predictions. Results suggest that deep learning architectures, particularly CNN-LSTM, show promise in capturing the complex dynamics of cryptocurrency prices. These findings contribute to the development of improved methodologies for cryptocurrency price prediction.
Keywords
cryptocurrency, machine learning, ARIMA, neural network
[1]. Hameed, S., & Farooq, S. (2017). The art of crypto currencies: A comprehensive analysis of popular crypto currencies. arXiv preprint arXiv:1711.11073.
[2]. Rebane, J., Karlsson, I., Papapetrou, P., & Denic, S. (2018). Seq2Seq RNNs and ARIMA models for cryptocurrency prediction: A comparative study. In SIGKDD Fintech’18, 19-23, 2018.
[3]. Dyhrberg, A. H. (2016). Bitcoin, gold and the dollar–A GARCH volatility analysis. Finance Research Letters, 16, 85-92.
[4]. Vidal, A., & Kristjanpoller, W. (2020). Gold volatility prediction using a CNN-LSTM approach. Expert Systems with Applications, 157, 113481.
[5]. Livieris, I. E., Pintelas, E., & Pintelas, P. (2020). A CNN–LSTM model for gold price time-series forecasting. Neural computing and applications, 32, 17351-17360.
[6]. Naved, M., & Srivastava, P. (2015). The profitability of five popular variations of moving averages on Indian market Index S&P CNX Nifty 50 during January 2004-December 2014. SSRN. 1-6.
[7]. Lee, S. (2004). Application of likelihood ratio and logistic regression models to landslide susceptibility mapping using GIS. Environmental Management, 34, 223-232.
[8]. Dutta, A., Bandopadhyay, G., & Sengupta, S. (2012). Prediction of stock performance in the Indian stock market using logistic regression. International Journal of Business and Information, 7(1), 105.
[9]. Ghaderpour, E., Pagiatakis, S. D., & Hassan, Q. K. (2021). A survey on change detection and time series analysis with applications. Applied Sciences, 11(13), 6141.
[10]. Thakkar, A., & Chaudhari, K. (2021). A comprehensive survey on deep neural networks for stock market: The need, challenges, and future directions. Expert Systems with Applications, 177, 114800.
Cite this article
Yu,S. (2024). Comparative analysis of machine learning techniques for cryptocurrency price prediction. Applied and Computational Engineering,32,1-12.
Data availability
The datasets used and/or analyzed during the current study will be available from the authors upon reasonable request.
Disclaimer/Publisher's Note
The statements, opinions and data contained in all publications are solely those of the individual author(s) and contributor(s) and not of EWA Publishing and/or the editor(s). EWA Publishing and/or the editor(s) disclaim responsibility for any injury to people or property resulting from any ideas, methods, instructions or products referred to in the content.
About volume
Volume title: Proceedings of the 2023 International Conference on Machine Learning and Automation
© 2024 by the author(s). Licensee EWA Publishing, Oxford, UK. This article is an open access article distributed under the terms and
conditions of the Creative Commons Attribution (CC BY) license. Authors who
publish this series agree to the following terms:
1. Authors retain copyright and grant the series right of first publication with the work simultaneously licensed under a Creative Commons
Attribution License that allows others to share the work with an acknowledgment of the work's authorship and initial publication in this
series.
2. Authors are able to enter into separate, additional contractual arrangements for the non-exclusive distribution of the series's published
version of the work (e.g., post it to an institutional repository or publish it in a book), with an acknowledgment of its initial
publication in this series.
3. Authors are permitted and encouraged to post their work online (e.g., in institutional repositories or on their website) prior to and
during the submission process, as it can lead to productive exchanges, as well as earlier and greater citation of published work (See
Open access policy for details).