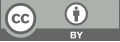
A study of human pose estimation in low-light environments using YOLOv8 model
- 1 International Engineering College, Xi’an University of Technology, Xi’an, 710054, China
- 2 School of Intelligent Engineering, Zhengzhou University of Aeronautics, Zhengzhou, 450046, China
* Author to whom correspondence should be addressed.
Abstract
Human pose estimation is a formidable task in the field of computer vision., often constrained by limited training samples and various complexities encountered during target detection, including complex backgrounds, object occlusion, crowded scenes, and varying perspectives. The primary objective of this research paper is to explore the performance disparities of the recently introduced YOLOv8 model in the context of human pose estimation. We conduct a comprehensive evaluation of six different models with varying complexities on the same low-light photograph to assess their precision and speed. The objective is to determine the suitability of each model for specific environmental contexts. The experimental results reveal that our findings demonstrate a partial regression in accuracy for the yolov8s-pose and yolov8m-pose models when tested on our sampled images. The increase in model layers indicates enhanced complexity and expressive power, while additional parameters signify improved learning capabilities at the expense of increased computational resource requirements.
Keywords
human detection, pose estimation, YOLOv8, low-light environments
[1]. Toshev, A., & Szegedy, C. DeepPose: Human Pose Estimation via Deep Neural Networks. In IEEE Conf. Comp. Vis. Patt. Recogn. 2014, 1653-1660.
[2]. LeCun, Y., Bottou, L., Bengio, Y., & Haffner, P. Gradient-Based Learning Applied to Document Recognition, Proceedings of the IEEE, 1998, 86(11), 2278-2324.
[3]. Zhang, H., Cui, Y., Zhang, L., Wu, S., & Zhang, H. CPM: A Large-scale Generative Chinese Pre-trained Language Model. arXiv preprint arXiv:2012.00413,2020.
[4]. Newell, A., Yang, K., & Deng, J. Stacked Hourglass Networks for Human Pose Estimation. Euro. Conf. Comp. Vis., 2020, 483-499.
[5]. Redmon, J., Divvala, S., Girshick, R., & Farhadi, A. You Only Look Once: Unified, Real-Time Object Detection. In IEEE Conf. Comp. Vis. Patt. Recogn., 2016, 779-788.
[6]. Zhou, X., Wang, D., & Krähenbühl, P. Objects as Points. arXiv preprint arXiv:1904.07850, 2019.
[7]. Haitong Lou, Xuehu Duan, Junmei Guo, DC-YOLOv8: Small-Size Object Detection Algorithm Based on Camera Sensor. Electronics 2023, 12(10), 2323.
[8]. Y. Chen et al., "CSPDarkNet53: A Light-weight Convolutional Neural Network for Object Detection with a Comprehensive Evaluation," IEEE Trans. Cir. Sys. Video Tech., 2020, 30 1, 1-14.
[9]. He, K., Zhang, X., Ren, S., & Sun, J.. Deep Residual Learning for Image Recognition. In In IEEE Conf. Comp. Vis. Patt. Recogn., 2021, 37-51.
[10]. Ren, S., He, K., Girshick, R., & Sun, J. Faster R-CNN: Towards real-time object detection with region proposal networks. In Adv. Neur. Inform. Proc. Sys. 2015, 91-99.
[11]. Zhu, X., He, C., & Zhang, J. Distribution Focal Loss for Dense Object Detection. In IEEE Conf. Comp. Vis. Patt. Recogn. 2019, 12814-12823.
Cite this article
Gu,K.;Su,B. (2024). A study of human pose estimation in low-light environments using YOLOv8 model. Applied and Computational Engineering,32,136-142.
Data availability
The datasets used and/or analyzed during the current study will be available from the authors upon reasonable request.
Disclaimer/Publisher's Note
The statements, opinions and data contained in all publications are solely those of the individual author(s) and contributor(s) and not of EWA Publishing and/or the editor(s). EWA Publishing and/or the editor(s) disclaim responsibility for any injury to people or property resulting from any ideas, methods, instructions or products referred to in the content.
About volume
Volume title: Proceedings of the 2023 International Conference on Machine Learning and Automation
© 2024 by the author(s). Licensee EWA Publishing, Oxford, UK. This article is an open access article distributed under the terms and
conditions of the Creative Commons Attribution (CC BY) license. Authors who
publish this series agree to the following terms:
1. Authors retain copyright and grant the series right of first publication with the work simultaneously licensed under a Creative Commons
Attribution License that allows others to share the work with an acknowledgment of the work's authorship and initial publication in this
series.
2. Authors are able to enter into separate, additional contractual arrangements for the non-exclusive distribution of the series's published
version of the work (e.g., post it to an institutional repository or publish it in a book), with an acknowledgment of its initial
publication in this series.
3. Authors are permitted and encouraged to post their work online (e.g., in institutional repositories or on their website) prior to and
during the submission process, as it can lead to productive exchanges, as well as earlier and greater citation of published work (See
Open access policy for details).