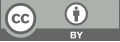
Data correlation and causal analysis for traffic flow prediction
- 1 Beijing Union University, 97 East North Fourth Ring Road, Chaoyang District, Beijing, China
* Author to whom correspondence should be addressed.
Abstract
Globally, traffic congestion has become a major issue due to several issues, including the rapid urban population increase, deteriorating infrastructure, improper and disorganized traffic signal timing, and a lack of real-time data. According to INRIX, a well-known provider of traffic data and analytics, the effects of this problem on U.S. travelers in 2017 were astronomical, totaling $305 billion in wasted fuel, lost time, and increased transportation costs in congested locations. Given the limitations of building new roads, communities must investigate cutting-edge tactics and technology to ease traffic while taking practical and economical restraints into account. This study employs the Granger causality test on a dataset of 48,120 entries, primarily focusing on the variables: number of VEHICLEs and number of intersection JUNCTIONs. The objective is to ascertain the potential mutual influence between these two variables. Initial results indicate a two-way Granger-causality between the variables, implying a feedback relationship. This discovery is fundamental in understanding traffic data dynamics and could be instrumental in enhancing traffic data prediction models.
Keywords
Correlation Analysis, Granger Causality Test, Traffic Flow
[1]. Bai, L., & Liu, R. (2023). Multistep Traffic Speed Forecasting Based on Dynamic Graph Convolutional Networks. UC San Diego.
[2]. T Osogami, T., Raymond, R., Suzuki, H., & Sato, H. (2023). Traffic Accident Prediction Based on Temporal Causal Discovery. University of Tokyo.
[3]. Li, Y., Prokhorov, D. (2022). Predicting Traffic Flow in Complex Networks Using Graph Neural Networks. Imperial College London.
[4]. Chen, J., Li, B., Liu, T., Wang, Y., & Song, G. (2019). Urban Road Traffic Flow Prediction: A Novel Hybrid Method Based on Wavelet Transform and Artificial Intelligence. IEEE Access journal.
[5]. Yang, J., Zhang, C., Wang, M., & Yang, J. (n.d.). Traffic Flow Forecasting Based on Wavelet Transform and Artificial Neural Network. Qingdao University of Science and Technology.
[6]. Lv, Y., Duan, L., Kang, W., & Li, H. (2018). Real-Time Traffic Flow Prediction Using Deep Learning for Intelligent Transportation Systems. IEEE Transactions on Intelligent Transportation Systems.
[7]. Guo, H., Zhang, Y., Wang, S., Fang, L., & Zhang, W. (2019). Traffic Flow Prediction Based on Improved LSTM. Mathematical Problems in Engineering.
[8]. Wu, B., Chen, Y., Yang, L., & Gong, Y. (2019). Traffic Flow Prediction Using LSTM and Attention Mechanism. IEEE Access.
[9]. Zhang, W., Wang, L., Cao, P., & Zhang, T. (n.d.). Modeling and Analysis of Traffic Flow in Urban Road Network Based on Complex Network Theory. China University of Transportation and Nanjing Normal University.
[10]. Bingyu Shen, Ying Tan, Department of Computer Science, Tsinghua University, "Traffic Flow Prediction Based on Graph Neural Networks", 2021.
[11]. Wang, S., Fang, Z., Li, J., & Xiang, Z. (Year of publication not provided). Traffic Flow Prediction Using Support Vector Machine and Particle Swarm Optimization.
[12]. Kang, Y., Qin, X., & Jia, J. (Year of publication not provided). A hybrid approach to short-term traffic flow forecasting using neural networks and ARIMA models.
[13]. Ma, X., Xia, X., & Yan, X. (Year of publication not provided). Real-time short-term traffic flow prediction with a seasonal autoregressive integrated moving average model.
[14]. Li, Y., Li, H., Wang, J., & Wang, Y. (2019). Road Traffic Flow Prediction Based on Machine Learning Techniques: A Survey. IEEE Access.
[15]. Al-Musawi, F. H., & Abdullah, A. H. (2019). A Review of Traffic Flow Prediction Using Machine Learning Techniques.
[16]. fedesoriano. (2021). Traffic Prediction Dataset [Data set]. Kaggle. https://www.kaggle.com/datasets/fedesoriano/traffic-prediction-dataset
Cite this article
Lan,G. (2024). Data correlation and causal analysis for traffic flow prediction. Applied and Computational Engineering,33,49-56.
Data availability
The datasets used and/or analyzed during the current study will be available from the authors upon reasonable request.
Disclaimer/Publisher's Note
The statements, opinions and data contained in all publications are solely those of the individual author(s) and contributor(s) and not of EWA Publishing and/or the editor(s). EWA Publishing and/or the editor(s) disclaim responsibility for any injury to people or property resulting from any ideas, methods, instructions or products referred to in the content.
About volume
Volume title: Proceedings of the 2023 International Conference on Machine Learning and Automation
© 2024 by the author(s). Licensee EWA Publishing, Oxford, UK. This article is an open access article distributed under the terms and
conditions of the Creative Commons Attribution (CC BY) license. Authors who
publish this series agree to the following terms:
1. Authors retain copyright and grant the series right of first publication with the work simultaneously licensed under a Creative Commons
Attribution License that allows others to share the work with an acknowledgment of the work's authorship and initial publication in this
series.
2. Authors are able to enter into separate, additional contractual arrangements for the non-exclusive distribution of the series's published
version of the work (e.g., post it to an institutional repository or publish it in a book), with an acknowledgment of its initial
publication in this series.
3. Authors are permitted and encouraged to post their work online (e.g., in institutional repositories or on their website) prior to and
during the submission process, as it can lead to productive exchanges, as well as earlier and greater citation of published work (See
Open access policy for details).