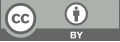
Customer Churn Analysis Prediction Based on Cluster Analysis and Machine Learning Algorithms
- 1 School of Economics and Management, Minjiang University, Fujian, Fuzhou, 350100, China
* Author to whom correspondence should be addressed.
Abstract
This paper focuses on the impact of customer churn on a company or organization and how to predict it. Customer churn refers to the loss of a company or organization's original customers, which can have a serious impact on a company's or organization's long-term growth and profitability. Therefore, it is important for a company or organization to understand the causes of customer churn and how to predict it. This paper statistically analyzes the customer churn rate for each category and finds that the difference between the churn rate and the non-churn rate for category 2 is very significant. The results of these analyses can help companies or organizations to better understand customer churn and take appropriate measures to reduce it. To predict customer churn, this paper uses two common machine learning models - decision tree and random forest model for prediction. The results show that the prediction accuracy of decision tree is 99%, while logistic regression is 90%. This indicates that the decision tree model has better performance in predicting customer churn. However, this paper also points out that the prediction results of different models may be different, so when predicting customer churn, multiple models should be considered and analyzed in context. In conclusion, customer churn is a serious problem faced by companies or organizations. This paper introduces some useful analytical methods and prediction models that can help companies or organizations better understand customer churn and take appropriate measures to reduce customer churn, thus improving the long-term growth and profitability of the company or organization.
Keywords
Cluster analysis, Decision tree, Machine learning algorithms
[1]. K. S W ,A. A A ,S. K W , et al.Customer churn prediction in telecom sector using machine learning techniques[J].Results in Control and Optimization,2024,14
[2]. Nisha M ,C. V J ,A. K , et al.A production inventory model with server breakdown and customer impatience[J].Annals of Operations Research,2023,331(2):1269-1304.
[3]. Asad K ,Zartashia M ,Hussain A , et al.Customer churn prediction using composite deep learning technique[J].Scientific Reports,2023,13(1):17294-17294.
[4]. Ele I S ,Alo* R U,Nweke F H, et al.Regression-Based Machine Learning Framework for Customer Churn Prediction in Telecommunication Industry[J].Journal of Advances in Information Technology,2023,14(5):
[5]. Yongkil A .Predicting customer attrition using binge trading patterns: Implications for the financial services industry[J].Journal of the Operational Research Society,2023,74(8):1878-1891.
[6]. Zahra S ,Omar H K ,Morteza S .Data-driven personalized assortment optimization by considering customers’ value and their risk of churning: Case of online grocery shopping[J]. Computers Industrial Engineering,2023,182.
[7]. Li J ,Bai X ,Xu Q , et al.Identification of Customer Churn Considering Difficult Case Mining[J].Systems,2023,11(7):
[8]. Yu F ,Bi W ,Cao N , et al.Customer Churn Prediction Framework of Inclusive Finance Based on Blockchain Smart Contract[J].Computer Systems Science and Engineering,2023,47(1):1-17.
[9]. MDS Global Launches Marketing Decision Intelligence Platform to Reduce Churn and Increase Revenue[J].Telecomworldwire,2023,
[10]. Soni K P ,Nelson L .PCP: Profit-Driven Churn Prediction using Machine Learning Techniques in Banking Sector[J].International Journal of Performability Engineering,2023,19(5):303-311.
Cite this article
Jiang,Y. (2024). Customer Churn Analysis Prediction Based on Cluster Analysis and Machine Learning Algorithms. Advances in Economics, Management and Political Sciences,77,192-198.
Data availability
The datasets used and/or analyzed during the current study will be available from the authors upon reasonable request.
Disclaimer/Publisher's Note
The statements, opinions and data contained in all publications are solely those of the individual author(s) and contributor(s) and not of EWA Publishing and/or the editor(s). EWA Publishing and/or the editor(s) disclaim responsibility for any injury to people or property resulting from any ideas, methods, instructions or products referred to in the content.
About volume
Volume title: Proceedings of the 3rd International Conference on Business and Policy Studies
© 2024 by the author(s). Licensee EWA Publishing, Oxford, UK. This article is an open access article distributed under the terms and
conditions of the Creative Commons Attribution (CC BY) license. Authors who
publish this series agree to the following terms:
1. Authors retain copyright and grant the series right of first publication with the work simultaneously licensed under a Creative Commons
Attribution License that allows others to share the work with an acknowledgment of the work's authorship and initial publication in this
series.
2. Authors are able to enter into separate, additional contractual arrangements for the non-exclusive distribution of the series's published
version of the work (e.g., post it to an institutional repository or publish it in a book), with an acknowledgment of its initial
publication in this series.
3. Authors are permitted and encouraged to post their work online (e.g., in institutional repositories or on their website) prior to and
during the submission process, as it can lead to productive exchanges, as well as earlier and greater citation of published work (See
Open access policy for details).