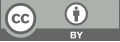
A Comparative Study of Stock Selection Models Based on Decision Tree Algorithms
- 1 School of Statistics and Mathematics, Central University of Finance and Economics, Beijing, 102206, China
* Author to whom correspondence should be addressed.
Abstract
In this paper, the decision tree model in data mining is applied to select stock characteristics that can be effectively used for stock selection by using the C4.5 algorithm and the CART algorithm, respectively, in combination with the strategies of fundamental analysis and technical analysis. The paper concludes that the decision tree models constructed by the C4.5 and CART algorithms both have better classification ability for stock selection and portfolio construction, but the decision tree model constructed by the C4.5 algorithm is simpler. The stock portfolios determined by the decision tree model are able to achieve an excess return of 13.4% relative to the CSI 300 index, thus proving that the decision tree model is effective in stock selection and stock portfolio construction.
Keywords
data mining, decision tree, C4.5 algorithm, CART algorithm, stock selection
[1]. Huang, C. J., Yang, D. X., Chuang, Y. T. (2008) Application of wrapper approach and composite classifier to the stock trend prediction. Expert Systems with Applications, 34(4): 2870-2878.
[2]. Basak, S., Kar, S., Saha, S., Khaidem, L., Dey, S. R. (2019) Predicting the direction of stock market prices using tree-based classifiers. The North American Journal of Economics and Finance, 47: 552-567.
[3]. Quinlan, J. R. (1992) C4. 5: programs for machine learning. Morgan Kaufmann, San Mateo, CA.
[4]. Breiman, L. (2017) Classification and regression trees. Routledge, New York.
[5]. Nottola, C., Condamin, L., & Naim, P. (1991, November). On the use of hard neural networks for symbolic learning application to company evaluation. In: [Proceedings] 1991 IEEE International Joint Conference on Neural Networks. Singapore. pp. 509-522.
[6]. Tsai, C. F., Wang, S. P. (2009) Stock price forecasting by hybrid machine learning techniques. In: Proceedings of the international multiconference of engineers and computer scientists. Hong Kong. Vol. 1, No. 755, pp. 60.
[7]. Nair, B. B., Dharini, N. M., Mohandas, V. P. (2010) A stock market trend prediction system using a hybrid decision tree-neuro-fuzzy system. In: 2010 international conference on advances in recent technologies in communication and computing. Kottayam. pp. 381-385.
[8]. Karim, R., Alam, M. K., Hossain, M. R. (2021) Stock market analysis using linear regression and decision tree regression. In: 2021 1st International Conference on Emerging Smart Technologies and Applications (eSmarTA). Sana'a. pp. 1-6.
[9]. Wu, M. C., Lin, S. Y., & Lin, C. H. (2006). An effective application of decision tree to stock trading. Expert Systems with applications, 31(2): 270-274.
[10]. Chen, M. Y. (2011) Predicting corporate financial distress based on integration of decision tree classification and logistic regression. Expert systems with applications, 38(9): 11261-11272.
Cite this article
Wang,Y. (2024). A Comparative Study of Stock Selection Models Based on Decision Tree Algorithms. Advances in Economics, Management and Political Sciences,82,182-194.
Data availability
The datasets used and/or analyzed during the current study will be available from the authors upon reasonable request.
Disclaimer/Publisher's Note
The statements, opinions and data contained in all publications are solely those of the individual author(s) and contributor(s) and not of EWA Publishing and/or the editor(s). EWA Publishing and/or the editor(s) disclaim responsibility for any injury to people or property resulting from any ideas, methods, instructions or products referred to in the content.
About volume
Volume title: Proceedings of the 2nd International Conference on Financial Technology and Business Analysis
© 2024 by the author(s). Licensee EWA Publishing, Oxford, UK. This article is an open access article distributed under the terms and
conditions of the Creative Commons Attribution (CC BY) license. Authors who
publish this series agree to the following terms:
1. Authors retain copyright and grant the series right of first publication with the work simultaneously licensed under a Creative Commons
Attribution License that allows others to share the work with an acknowledgment of the work's authorship and initial publication in this
series.
2. Authors are able to enter into separate, additional contractual arrangements for the non-exclusive distribution of the series's published
version of the work (e.g., post it to an institutional repository or publish it in a book), with an acknowledgment of its initial
publication in this series.
3. Authors are permitted and encouraged to post their work online (e.g., in institutional repositories or on their website) prior to and
during the submission process, as it can lead to productive exchanges, as well as earlier and greater citation of published work (See
Open access policy for details).