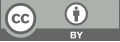
Research of Forecasting Chinese Gross Domestic Product Based on Auto Regressive Integrated Moving Average Algorithm and Linear Regression Model
- 1 University of California, Davis, Davis, California,95617, United States
* Author to whom correspondence should be addressed.
Abstract
Gross domestic product (GDP) is an important indicator for measuring a country's economic development level. By studying GDP, it can analyze the economic structure of a country or region, determine the development trend of a country. GDP is widely applied in fields such as macroeconomic analysis, policy formulation, and international economic comparison. After Chinese reform and opening up, the economy has developed rapidly, and the GDP has shown a trend of increasing year by year. Therefore, the analysis of Chinese GDP is meaningful. This article analyzes GDP data from 2001 to 2017. Linear regression method is used for prediction first but the goodness of fit for this model to predict GDP is not very high. So then the Auto Regressive Integrated Moving Average (ARIMA) model is used for prediction. The results indicate that the ARIMA algorithm has higher goodness of fit and can better illustrate the situation, which can be used for short-term prediction in China. All this model is realized in the python.
Keywords
GDP, ARIMA, Forecasting, Time Series
[1]. Yu Lianmin, The Application of ARIMA Model in China's GDP Forecast, Times Finance, no. 21, pp. 1, 2017
[2]. Rawski, T. G. (2001). What is happening to China’s GDP statistics?, China Economic Review, 12(4), 347–354. doi:10.1016/s1043-951x(01)00062-1
[3]. Chen, M., Kwok, C. L., Shan, H., & Yip, P. S. F. (2018). Decomposing and Predicting China’s GDP Growth: Past, Present, and Future, Population and Development Review, 44(1), 143–157. doi:10.1111/padr.12129
[4]. Asif Mahmud, Ian Hamilton, Vikash V. Gayah, Richard J. Porter, Estimation of VMT using heteroskedastic log-linear regression models, Transportation Letters, 2023, ISSN 1942-7867,
[5]. Chun-Hua Chien, Amy J.C. Trappey, Chien-Chih Wang, ARIMA-AdaBoost hybrid approach for product quality prediction in advanced transformer manufacturing, Advanced Engineering Informatics, Volume 57, 2023, 102055, ISSN 1474-0346
[6]. HHXUN, ARIMA model introduction, 2018-04-08 21:52:10
[7]. Jun Luo, Yaping Gong, Air pollutant prediction based on ARIMA-WOA-LSTM model, Atmospheric Pollution Research,Volume 14, Issue 6,2023,101761,ISSN 1309-1042,
[8]. Douglas C. Montgomery, Elizabeth A. Peck,G. Geoffrey Vining, Introduction to linear regression analysis, pp. 13 , 2021
[9]. Draper, N.R. and Smith, H. Applied Regression Analysis. Wiley Series in Probability and Statistics. 1998.
[10]. S.L. Ho and M. XIE, THE USE OF ARIMA MODELS FOR RELIABILITY FORECASTING AND ANALYSIS, Computers & industrial engineering, 1998 - Elsevier
Cite this article
Zhang,A. (2024). Research of Forecasting Chinese Gross Domestic Product Based on Auto Regressive Integrated Moving Average Algorithm and Linear Regression Model. Advances in Economics, Management and Political Sciences,101,151-160.
Data availability
The datasets used and/or analyzed during the current study will be available from the authors upon reasonable request.
Disclaimer/Publisher's Note
The statements, opinions and data contained in all publications are solely those of the individual author(s) and contributor(s) and not of EWA Publishing and/or the editor(s). EWA Publishing and/or the editor(s) disclaim responsibility for any injury to people or property resulting from any ideas, methods, instructions or products referred to in the content.
About volume
Volume title: Proceedings of the 2nd International Conference on Financial Technology and Business Analysis
© 2024 by the author(s). Licensee EWA Publishing, Oxford, UK. This article is an open access article distributed under the terms and
conditions of the Creative Commons Attribution (CC BY) license. Authors who
publish this series agree to the following terms:
1. Authors retain copyright and grant the series right of first publication with the work simultaneously licensed under a Creative Commons
Attribution License that allows others to share the work with an acknowledgment of the work's authorship and initial publication in this
series.
2. Authors are able to enter into separate, additional contractual arrangements for the non-exclusive distribution of the series's published
version of the work (e.g., post it to an institutional repository or publish it in a book), with an acknowledgment of its initial
publication in this series.
3. Authors are permitted and encouraged to post their work online (e.g., in institutional repositories or on their website) prior to and
during the submission process, as it can lead to productive exchanges, as well as earlier and greater citation of published work (See
Open access policy for details).