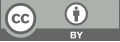
Predictive Analytics in Consumer Behavior Forecasting: A Literature Review
- 1 Nanjing Foreign Language School
* Author to whom correspondence should be addressed.
Abstract
This paper introduces non-experts to the process of using predictive analytics models to solve real-world classification and prediction problems. There are two types of models: decision trees and regression models. This article summarizes the previous understanding and application of predictive models and consumer behavior, and also introduces the better use of predictive analysis models, including the accuracy and efficiency of each model. Predictive analytics models are widely used in our lives. Anyone interested in either business or medical science can learn this technology to better explore different fields. The results of this paper suggest that people can further innovate such models, so as to make the use of methods simpler and more efficient.
Keywords
Predictive analytics, Regression model, Logit model, Decision tree method, Consumer behaviour forecasting
[1]. Kursuncu, U., Gaur, M., Lokala, U., Thirunarayan, K., Sheth, A., & Arpinar, I. B. (2019). Predictive Analysis on Twitter: Techniques and Applications. In N. Agarwal, N. Dokoohaki, & S. Tokdemir (Eds.), Emerging Research Challenges and Opportunities in Computational Social Network Analysis and Mining (pp. 67–104). Springer International Publishing.
[2]. Lee, C. S., Cheang, P. Y. S., & Moslehpour, M. (2022). Predictive analytics in business analytics: decision tree. Advances in Decision Sciences, 26(1), 1-29.
[3]. Jahnke, H. N. (2003). A history of analysis (No. 24). American Mathematical Soc.
[4]. Elkan, C. (2013). Predictive analytics and data mining (Vol. 600). San Diego: University of California.
[5]. Kumar, V., & Garg, M. L. (2018). Predictive analytics: a review of trends and techniques. International Journal of Computer Applications, 182(1), 31-37.
[6]. 14Jacoby, J. (1976). Consumer psychology: An octennium. Annual review of psychology, 27(1), 331-358.
[7]. Jacoby, J., Szybillo, G. J., & Berning, C. K. (1976). Time and consumer behavior: An interdisciplinary overview. Journal of consumer research, 2(4), 320-339.
[8]. Mincer, J. (1963). Market prices, opportunity costs, and income effects. Measurement in economics, 67-82.
[9]. Zufryden, F. S., Pedrick, J. H., & Sankaralingam, A. (1993). Zapping and its impact on brand purchase behavior. Journal of Advertising Research, 33(1), 58-67.
[10]. Jacoby, J., Johar, G. V., & Morrin, M. (1998). Consumer behavior: A quadrennium. Annual review of psychology, 49(1), 319-344.
[11]. Schmitt, B. H., Pan, Y., & Tavassoli, N. T. (1994). Language and consumer memory: The impact of linguistic differences between Chinese and English. Journal of consumer research, 21(3), 419-431.
[12]. Sundie, J. M., Pandelaere, M., Lens, I., & Warlop, L. (2020). Setting the bar: The influence of women’s conspicuous display on men’s affiliative behavior. Journal of Business Research, 120, 569–585.
[13]. Hunt, J. M., Kernan, J. B., & Mitchell, D. J. (1996). Materialism as social cognition: People, possessions, and perception. Journal of Consumer Psychology, 5(1), 65-83.
[14]. Değirmencioğlu, Y., & Akyurt, İ. Z. (2023). Forecasting. Smart and Sustainable Operations and Supply Chain Management in Industry 4.0.
[15]. Boone, T., Ganeshan, R., Jain, A., & Sanders, N. R. (2019). Forecasting sales in the supply chain: Consumer analytics in the big data era. International Journal of Forecasting, 35(1), 170–180.
[16]. Kamiński, B., Jakubczyk, M., & Szufel, P. (2018). A framework for sensitivity analysis of decision trees. Central European journal of operations research, 26, 135-159.
[17]. Borgonovo, E., & Marinacci, M. (2015). Decision analysis under ambiguity. European Journal of Operational Research, 244(3), 823-836
[18]. Armstrong, J. S. (2012). Illusions in regression analysis. International Journal of Forecasting, 28(3), 689–694.
[19]. 33Armstrong, J. S. (2012). Illusions in regression analysis. International Journal of Forecasting, 28(3), 689–694.
[20]. Pant, P. N., & Starbuck, W. H. (1990). Innocents in the forest: Forecasting and research methods. Journal of Management, 16(2), 433-460.
[21]. Zellner, A. (2001). 14 Keep it sophisticatedly simple. This page intentionally left blank, 242.
[22]. Nusinovici, S., Tham, Y. C., Chak Yan, M. Y., Wei Ting, D. S., Li, J., Sabanayagam, C., Wong, T. Y., & Cheng, 36C.-Y. (2020). Logistic regression was as good as machine learning for predicting major chronic diseases. Journal of Clinical Epidemiology, 122, 56–69.
[23]. Tom, E., & Schulman, K. A. (1997). Mathematical models in decision analysis. Infection Control & Hospital Epidemiology, 18(1), 65-73.
[24]. Ciotti, M., Ciccozzi, M., Terrinoni, A., Jiang, W. C., Wang, C. B., & Bernardini, S. (2020). The COVID-19 pandemic. Critical reviews in clinical laboratory sciences, 57(6), 365-388.
[25]. Safara, F. (2022). A computational model to predict consumer behaviour during COVID-19 pandemic. Computational Economics, 59(4), 1525-1538.
[26]. Ropuszyńska-Surma, E. (2020). The Behavioural Profiles of Energy Consumers: Comparison of the Decision Tree Method and the Logit Model. In W. Sroka (Ed.), & M. Węglarz (Trans.), Perspectives on Consumer Behaviour (pp. 211–239). Springer International Publishing.
Cite this article
Wang,R. (2024). Predictive Analytics in Consumer Behavior Forecasting: A Literature Review. Advances in Economics, Management and Political Sciences,106,34-41.
Data availability
The datasets used and/or analyzed during the current study will be available from the authors upon reasonable request.
Disclaimer/Publisher's Note
The statements, opinions and data contained in all publications are solely those of the individual author(s) and contributor(s) and not of EWA Publishing and/or the editor(s). EWA Publishing and/or the editor(s) disclaim responsibility for any injury to people or property resulting from any ideas, methods, instructions or products referred to in the content.
About volume
Volume title: Proceedings of the 3rd International Conference on Business and Policy Studies
© 2024 by the author(s). Licensee EWA Publishing, Oxford, UK. This article is an open access article distributed under the terms and
conditions of the Creative Commons Attribution (CC BY) license. Authors who
publish this series agree to the following terms:
1. Authors retain copyright and grant the series right of first publication with the work simultaneously licensed under a Creative Commons
Attribution License that allows others to share the work with an acknowledgment of the work's authorship and initial publication in this
series.
2. Authors are able to enter into separate, additional contractual arrangements for the non-exclusive distribution of the series's published
version of the work (e.g., post it to an institutional repository or publish it in a book), with an acknowledgment of its initial
publication in this series.
3. Authors are permitted and encouraged to post their work online (e.g., in institutional repositories or on their website) prior to and
during the submission process, as it can lead to productive exchanges, as well as earlier and greater citation of published work (See
Open access policy for details).