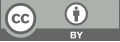
Quantitative Strategies Based on FinGPT’s Analysis of the Federal Reserve’s Attitude
- 1 University of Minnesota, 100 Church Street SE, Minneapolis, MN 55455, Twin City, USA
* Author to whom correspondence should be addressed.
Abstract
This paper explores the impact of the Federal Reserve (Fed) on financial markets, specifically through the sentiment scoring of Fed meeting minutes by the AI analysis model FinGPT and its correlation with market performance. The article first introduces the method of sentiment analysis using FinGPT and conducts an in-depth analysis of the meeting minutes from 1990 to 2020 using this method. Furthermore, key market data, such as the S&P 500 Index, real estate, and financial sector indices, are used to assess the actual impact of the Fed’s attitude on market dynamics. Through quantitative trading and backtesting analysis, this study verifies the performance and effectiveness of a single-factor strategy in different markets. The results show that while the Fed’s sentiment scores have high predictive validity and market adaptability in the financial sector, their performance in the real estate market and the broader stock market index is relatively weaker. The findings indicate that the market applicability of the strategy is significantly influenced by market type and economic environment. Future strategy optimization needs to consider these factors to enhance adaptability and efficiency across different markets.
Keywords
Federal Reserve, AI Analysis, FinGPT, Quantitative Trading Analysis
[1]. Ai, H., & Bansal, R. (2018). Risk preferences and the macroeconomic announcement premium. Econometrica, 86(4), 1383-1430.
[2]. Malkiel, B. G. (1989). Efficient Market Hypothesis. In J. Eatwell, M. Milgate, & P. Newman (Eds.), Finance. The New Palgrave (pp. 1-20). Palgrave Macmillan, London. https://doi.org/10.1007/978-1-349-20213-3_13
[3]. Rigobon, R., & Sack, B. (2004). The impact of monetary policy on asset prices. Journal of Monetary Economics, 51(8), 1553-1575. https://doi.org/10.1016/j.jmoneco.2004.02.004
[4]. Lee J, Stevens N, Han S C, et al. A survey of large language models in finance (finllms)[J]. arXiv preprint arXiv:2402.02315, 2024.
[5]. Wang, N., Yang, H., & Wang, C. D. (2023). Fingpt: Instruction tuning benchmark for open-source large language models in financial datasets. arXiv preprint arXiv:2310.04793.
[6]. Federal Reserve. (n.d.). Historical Materials by Year. Retrieved May 29, 2024, from https://www.federalreserve.gov/monetarypolicy/fomc_historical_year.htm
[7]. Antipova, T. (2021). Coronavirus Pandemic as Black Swan Event. In T. Antipova (Ed.), Integrated Science in Digital Age 2020. ICIS 2020. Lecture Notes in Networks and Systems, vol 136. Springer, Cham. https://doi.org/10.1007/978-3-030-49264-9_32
[8]. Morales, L., & Andreosso-O'Callaghan, B. (2020). Covid19: Global Stock Markets “Black Swan.” Critical Letters in Economics & Finance, 1(1), Article 1. https://doi.org/10.21427/gv7k-1c77. Retrieved from https://arrow.tudublin.ie/clef/vol1/iss1/1
Cite this article
Chen,S. (2024). Quantitative Strategies Based on FinGPT’s Analysis of the Federal Reserve’s Attitude. Advances in Economics, Management and Political Sciences,90,82-90.
Data availability
The datasets used and/or analyzed during the current study will be available from the authors upon reasonable request.
Disclaimer/Publisher's Note
The statements, opinions and data contained in all publications are solely those of the individual author(s) and contributor(s) and not of EWA Publishing and/or the editor(s). EWA Publishing and/or the editor(s) disclaim responsibility for any injury to people or property resulting from any ideas, methods, instructions or products referred to in the content.
About volume
Volume title: Proceedings of the 3rd International Conference on Financial Technology and Business Analysis
© 2024 by the author(s). Licensee EWA Publishing, Oxford, UK. This article is an open access article distributed under the terms and
conditions of the Creative Commons Attribution (CC BY) license. Authors who
publish this series agree to the following terms:
1. Authors retain copyright and grant the series right of first publication with the work simultaneously licensed under a Creative Commons
Attribution License that allows others to share the work with an acknowledgment of the work's authorship and initial publication in this
series.
2. Authors are able to enter into separate, additional contractual arrangements for the non-exclusive distribution of the series's published
version of the work (e.g., post it to an institutional repository or publish it in a book), with an acknowledgment of its initial
publication in this series.
3. Authors are permitted and encouraged to post their work online (e.g., in institutional repositories or on their website) prior to and
during the submission process, as it can lead to productive exchanges, as well as earlier and greater citation of published work (See
Open access policy for details).