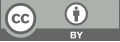
A Research of Fine-Tuning CNN-LSTM Model for Gold Price Prediction
- 1 School of Intelligent Systems Science and Engineering, Jinan University, Zhuhai, 519070, China
* Author to whom correspondence should be addressed.
Abstract
Gold price and foreign exchange price prediction are usually regarded as serialized data with strong time correlation. It is precisely because of this feature that the performance of traditional convolutional neural networks is often not as good as that of recurrent neural networks. This paper fine-tunes the CNN-LSTM model in two ways. The first is to simplify the input data, convert the time series into binary data, and change the model task from predicting prices to predicting price fluctuations. The second is to add a dropout layer and use the dropout layer to improve the model's overfitting problem. The results demonstrate that the improved model outperforms the first model, with prediction accuracy rising from 54% to 57%. Furthermore, the refined model is not limited to processing the gold price series; it can also be applied to other time series, and when paired with other models, it could yield even better outcomes.
Keywords
CNN-LSTM Model, Gold Price Prediction, Time Series Data
[1]. Zhu, J., Han, W., & Zhang, J. (2023). Does climate risk matter for gold price volatility? Finance Research Letters, 58, 104544.
[2]. Baur, D. G., & McDermott, T. K. (2010). Is gold a safe haven? international evidence. Journal of Banking & Finance, 34(8), 1886–1898.
[3]. Shafiee, S., & Topal, E. (2010). An overview of global gold market and gold price forecasting. Resources Policy, 35(3), 178–189.
[4]. Makala, D., & Li, Z. (2021). Prediction of gold price with ARIMA and SVM. In Journal of Physics: Conference Series (Vol. 1767, p. 012022). IOP Publishing.
[5]. Zhang, X., & Zhang, Q. (2020). Short-term traffic flow prediction based on LSTM-XGBoost combination model. Computer Modeling in Engineering & Sciences, 125(1), 95–109.
[6]. Livieris, I. E., Pintelas, E., & Pintelas, P. (2020). A CNN–LSTM model for gold price time-series forecasting. Neural Computing and Applications, 32, 17351–17360.
[7]. AbouHassan, I., Kasabov, N., Bankar, T., Garg, R., & Bhattacharya, B. S. (2023). PAMET-SNN: Predictive associative memory for multiple time series based on spiking neural networks with case studies in economics and finance. Authorea Preprints.
[8]. Li, Z., Liu, F., Yang, W., Peng, S., & Zhou, J. (2022). A survey of convolutional neural networks: Analysis, applications, and prospects. IEEE Transactions on Neural Networks and Learning Systems, 33(12), 6999–7019.
[9]. Graves, A., & Graves, A. (2012). Long short-term memory. In Supervised Sequence Labelling with Recurrent Neural Networks (pp. 37–45).
[10]. Srivastava, N., Hinton, G., Krizhevsky, A., Sutskever, I., & Salakhutdinov, R. (2014). Dropout: A simple way to prevent neural networks from overfitting. The Journal of Machine Learning Research, 15(1), 1929–1958.
Cite this article
Luo,Y. (2024). A Research of Fine-Tuning CNN-LSTM Model for Gold Price Prediction. Advances in Economics, Management and Political Sciences,94,157-163.
Data availability
The datasets used and/or analyzed during the current study will be available from the authors upon reasonable request.
Disclaimer/Publisher's Note
The statements, opinions and data contained in all publications are solely those of the individual author(s) and contributor(s) and not of EWA Publishing and/or the editor(s). EWA Publishing and/or the editor(s) disclaim responsibility for any injury to people or property resulting from any ideas, methods, instructions or products referred to in the content.
About volume
Volume title: Proceedings of ICFTBA 2024 Workshop: Finance in the Age of Environmental Risks and Sustainability
© 2024 by the author(s). Licensee EWA Publishing, Oxford, UK. This article is an open access article distributed under the terms and
conditions of the Creative Commons Attribution (CC BY) license. Authors who
publish this series agree to the following terms:
1. Authors retain copyright and grant the series right of first publication with the work simultaneously licensed under a Creative Commons
Attribution License that allows others to share the work with an acknowledgment of the work's authorship and initial publication in this
series.
2. Authors are able to enter into separate, additional contractual arrangements for the non-exclusive distribution of the series's published
version of the work (e.g., post it to an institutional repository or publish it in a book), with an acknowledgment of its initial
publication in this series.
3. Authors are permitted and encouraged to post their work online (e.g., in institutional repositories or on their website) prior to and
during the submission process, as it can lead to productive exchanges, as well as earlier and greater citation of published work (See
Open access policy for details).