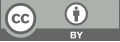
Portfolio Optimization Strategy Based on Fama-French and LightGBM
- 1 Fisher College of Business, The Ohio State University, Columbus, Ohio, 43210, The United States of America
* Author to whom correspondence should be addressed.
Abstract
Financial portfolio optimization is the process of selecting the optimal combination of assets to generate returns within a specified risk threshold. Investors and financial institutions put a lot of time into this process, trying to find the optimal weights. This study selects eight top performers from different industry sectors. The study first uses the Fama-French model to process the first 83.33% of data and make predictions for the remaining 16.67% directly at once. Next, the LightGBM model is applied to forecast returns. This approach enables researchers to identify the maximum return model and the minimum risk model on the efficient frontier using the mean variance model and Monte Carlo simulations. By comparing the performance of the models with the benchmark S&P 500 over the same period, the study concludes that all models in the study delivered superior results. This research highlights a novel portfolio method that applies the Fama-French model or LightGBM model for prediction. The results underscore the strength of integrating these two models in portfolio optimization and showcase the useful applications of CPAM and machine learning in the financial field.
Keywords
Portfolio Optimization, Mean-Variance Model, Mento Carlo Simulation, Fama-French Model, LightGBM Model
[1]. Kapoor, N. (2014). Financial Portfolio management: Overview and Decision Making in investment Process. International Journal of Research (IJR), 1.
[2]. Constantinides, G. M., Malliaris, A. G. (1995). Portfolio Theory. Handbooks in OR & MS, 9.
[3]. Halim, N. A., Yuliati, A. (2020). Markowitz Model Investment Portfolio Optimization: a Review Theory. International Journal of Research in Community Service, 1(3), 14-18.
[4]. Putra, J. M., Soehaditama, J. P., Hernawan, M. A., Yulihapsari, I. U., Sova, M. (2023). Implementing the Capital Asset Pricing Model in Forecasting Stock Returns: A Literature Review. Indonesian Journal of Business Analytics (IJBA), 3(2), 171-182.
[5]. Karp, A., & Vuuren, G. V. (2017). The capital asset pricing model and fama-french three factor model in an emerging market environment. International Business & Economics Research Journal, 16(3).
[6]. Chen, L., Pelger, M., & Zhu, J. (2021). Deep Learning in Asset Pricing. Journal of Financial Economics, 142(3), 994-1015.
[7]. Heaton, J. B., Polson, N. G., & Witte, J. H. (2017). Deep learning for finance: deep portfolios. Applied Stochastic Models in Business and Industry, 33(1), 3-12.
[8]. Rasekhschaffe, K. C., & Jones, R. (2019). Machine learning for stock selection. Financial Analysts Journal, 75(3), 70-88.
[9]. Ke, G., Meng, Q., Finley, T., Wang, T., Chen, W., Ma, W., Ye, Q., & Liu, T. Y. (2017). LightGBM: A Highly Efficient Gradient Boosting Decision Tree. NIPS, 3149-3157.
[10]. Far, M. S., & Wang, Y. (2016). Approximation of the Monte Carlo Sampling Method for Reluability Analysis of Structures. Reasearhgate, 9.
[11]. Kent, L. W., & Ying, Z. (2003). Understanding Risk and Return, the CAPM, and the Fama-French Three-Factor Model. SSRN.
Cite this article
Xiao,M. (2024). Portfolio Optimization Strategy Based on Fama-French and LightGBM. Advances in Economics, Management and Political Sciences,90,169-179.
Data availability
The datasets used and/or analyzed during the current study will be available from the authors upon reasonable request.
Disclaimer/Publisher's Note
The statements, opinions and data contained in all publications are solely those of the individual author(s) and contributor(s) and not of EWA Publishing and/or the editor(s). EWA Publishing and/or the editor(s) disclaim responsibility for any injury to people or property resulting from any ideas, methods, instructions or products referred to in the content.
About volume
Volume title: Proceedings of the 3rd International Conference on Financial Technology and Business Analysis
© 2024 by the author(s). Licensee EWA Publishing, Oxford, UK. This article is an open access article distributed under the terms and
conditions of the Creative Commons Attribution (CC BY) license. Authors who
publish this series agree to the following terms:
1. Authors retain copyright and grant the series right of first publication with the work simultaneously licensed under a Creative Commons
Attribution License that allows others to share the work with an acknowledgment of the work's authorship and initial publication in this
series.
2. Authors are able to enter into separate, additional contractual arrangements for the non-exclusive distribution of the series's published
version of the work (e.g., post it to an institutional repository or publish it in a book), with an acknowledgment of its initial
publication in this series.
3. Authors are permitted and encouraged to post their work online (e.g., in institutional repositories or on their website) prior to and
during the submission process, as it can lead to productive exchanges, as well as earlier and greater citation of published work (See
Open access policy for details).