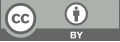
The Impact of AI Industry Growth on U.S. AI Sector Stocks: A Machine Learning Analysis
- 1 Faculty of Science, University of Melbourne, Melbourne, Australia
* Author to whom correspondence should be addressed.
Abstract
The rapid development of artificial intelligence (AI) since 2020 has significantly impacted the U.S. stock market, necessitating a deeper understanding of its influence on AI-related stocks. This study aims to analyze and predict the returns of the Global X Robotics & Artificial Intelligence ETF (BOTZ) as a proxy for AI industry performance. Employing Random Forest and XGBoost machine learning models, we trained on over a thousand data points to forecast BOTZ ETF returns. Our research reveals that AI-focused stocks and ETFs have outperformed the broader market since 2020, driven by increased AI adoption across industries, substantial research and development investments, and shifting investor sentiment towards tech-centric portfolios. The machine learning models demonstrated promising results in capturing complex market dynamics and providing reliable predictions. This study underscores the potential of integrating machine learning with financial analysis, offering valuable insights for investors and stakeholders in navigating the evolving landscape of AI-influenced markets.
Keywords
Machine Learning, AI Industry, US. Stock Market
[1]. Zhao, S. (2022). Stock Return Prediction Using Machine Learning Classifiers. In 2022 2nd International Conference on Enterprise Management and Economic Development (ICEMED 2022), 1347-1351.
[2]. Nguyen, T. N., Ho-Phuoc, T., Nguyen, D. T., & Mac, M. N. (2020). Stock Return Prediction using Machine Learning-Based Techniques. Journal of Science and Technology: Issue on Information and Communications Technology, 18(12.2), 49-56.
[3]. Choi, W., Jang, S., Kim, S., Park, C., Park, S., & Song, S. (2024). Return prediction by machine learning for the Korean stock market. Journal of the Korean Statistical Society, 53(1), 248-280.
[4]. Meher, B. K., Anand, A., Kumar, S., Birau, R., & Singh, M. (2024). Effectiveness of random forest model in predicting stock prices of solar energy companies in India. International Journal of Energy Economics and Policy, 14(2), 426-434.
[5]. Wang, J. (2022). The Comparsion of Stock Return Prediction for Random Forest, Ordinary Least Square, and XGBoost. BCP Business & Management, 26, 686-695.
[6]. Hongjoong, K. I. M. (2021). Mean-variance portfolio optimization with stock return prediction using XGBoost. Economic Computation & Economic Cybernetics Studies & Research, 55(4).
[7]. Ye, F., Wang, J., Li, Z., Jihan, Z., & Yang, C. (2021). Jane Street Stock prediction model based on LightGBM. 2021 6th International Conference on Intelligent Computing and Signal Processing (ICSP), 385-388.
[8]. Saetia, K., & Yokrattanasak, J. (2022). Stock movement prediction using machine learning based on technical indicators and Google trend searches in Thailand. International Journal of Financial Studies, 11(1), 5.
[9]. Xiao, H. (2023). Econometrics and Machine Learning Approach on Correlation in Stock Return between US Firms and Chinese Suppliers. Advances in Economics, Management and Political Sciences. Retrieved from: https://www.semanticscholar.org/search?q=Econometrics%20and%20Machine%20Learning%20Approach%20on%20Correlation%20in%20Stock%20Return%20between%20US%20Firms%20and%20Chinese%20Suppliers&sort=relevance.
[10]. Chen, X. (2024). Machine Learning Based Stock Return Prediction and Portfolio Research. Operations Research and Fuzziology. Retrieved from: https://www.semanticscholar.org/paper/Machine-Learning-Based-Stock-Return-Prediction-and-%E6%AC%A3/affe5fe897cf2fcb46239615a38ff2c5e70825f9.
Cite this article
Li,J. (2024). The Impact of AI Industry Growth on U.S. AI Sector Stocks: A Machine Learning Analysis. Advances in Economics, Management and Political Sciences,94,175-186.
Data availability
The datasets used and/or analyzed during the current study will be available from the authors upon reasonable request.
Disclaimer/Publisher's Note
The statements, opinions and data contained in all publications are solely those of the individual author(s) and contributor(s) and not of EWA Publishing and/or the editor(s). EWA Publishing and/or the editor(s) disclaim responsibility for any injury to people or property resulting from any ideas, methods, instructions or products referred to in the content.
About volume
Volume title: Proceedings of ICFTBA 2024 Workshop: Finance in the Age of Environmental Risks and Sustainability
© 2024 by the author(s). Licensee EWA Publishing, Oxford, UK. This article is an open access article distributed under the terms and
conditions of the Creative Commons Attribution (CC BY) license. Authors who
publish this series agree to the following terms:
1. Authors retain copyright and grant the series right of first publication with the work simultaneously licensed under a Creative Commons
Attribution License that allows others to share the work with an acknowledgment of the work's authorship and initial publication in this
series.
2. Authors are able to enter into separate, additional contractual arrangements for the non-exclusive distribution of the series's published
version of the work (e.g., post it to an institutional repository or publish it in a book), with an acknowledgment of its initial
publication in this series.
3. Authors are permitted and encouraged to post their work online (e.g., in institutional repositories or on their website) prior to and
during the submission process, as it can lead to productive exchanges, as well as earlier and greater citation of published work (See
Open access policy for details).