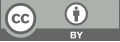
The Impact and Future of Generative Pre-trained Transformers: A Study of GPT in Enhancing Business and Technology
- 1 School of Finance & Investment, Guangdong University of Finance, Longdong Street, Guangzhou, China
* Author to whom correspondence should be addressed.
Abstract
With the development of artificial intelligence (AI), natural language processing (NIP) has clearly become the key to enhancing AI capabilities, especially through the deployment of GPT. This paper explores the major advances and related commercial applications of GPT, as it is at the cutting edge of AI technology with far-reaching capabilities in terms of text generation, language understanding, and human-machine interaction. Developed by OpenAI, GPT uses innovative transformer architecture to make breakthroughs in a variety of language tasks, marking a major evolution in the potential applications of AI in various industries. This research explores the integration of GPT in sectors, highlighting how its complex text processing capabilities can be utilized to improve efficiency and innovative service delivery. The study highlights the transformative impact of GPT on business efficiency, strategic decision-making, and customer engagement, thanks to its scalable and adaptable AI framework. Finally, the document reflects the need for a strong ethical framework and regulatory supervision to guide the deployment of GPT technologies. As the Global Trade Agreement evolves, it is committed to shaping the future of global industry by promoting innovation while calling for a balanced approach to ethical considerations and social impacts.
Keywords
Generative pre-trained transformers, Natural language processing, Commercial applications, Ethical AI implementation, Investment and financing
[1]. Bahja M. Natural language processing applications in business[J]. E-Business-higher education and intelligence applications, 2020.
[2]. Just J. Natural language processing for innovation search–Reviewing an emerging non-human innovation intermediary[J]. Technovation, 2024, 129: 102883.
[3]. Huang S. Exploring the Influence of Natural Language Processing Technology on Marketing Strategy Innovation Management in Emerging Markets for Multinational Corporations[J].
[4]. Sirignano J, Cont R. Universal features of price formation in financial markets: perspectives from deep learning[M]//Machine learning and AI in finance. Routledge, 2021: 5-15.
[5]. Gonçalves R, Ribeiro V M, Pereira F L, et al. Deep learning in exchange markets[J]. Information Economics and Policy, 2019, 47: 38-51.
[6]. Di Vaio A, Palladino R, Hassan R, et al. Artificial intelligence and business models in the sustainable development goals perspective: A systematic literature review[J]. Journal of Business Research, 2020, 121: 283-314.
[7]. Kulkov I. Next-generation business models for artificial intelligence start-ups in the healthcare industry[J]. International Journal of Entrepreneurial Behavior & Research, 2023, 29(4): 860-885.
[8]. Garbuio M, Lin N. Artificial intelligence as a growth engine for health care startups: Emerging business models[J]. California Management Review, 2019, 61(2): 59-83.
[9]. Burström T, Parida V, Lahti T, et al. AI-enabled business-model innovation and transformation in industrial ecosystems: A framework, model and outline for further research[J]. Journal of Business Research, 2021, 127: 85-95.
[10]. Weber M, Beutter M, Weking J, et al. AI startup business models: Key characteristics and directions for entrepreneurship research[J]. Business & Information Systems Engineering, 2022, 64(1): 91-109.
[11]. Åström J, Reim W, Parida V. Value creation and value capture for AI business model innovation: a three-phase process framework[J]. Review of Managerial Science, 2022, 16(7): 2111-2133.
[12]. Watanabe M, Uchihira N. Digital Business Model Analysis Using a Large Language Model[J]. arXiv preprint arXiv:2406.05741, 2024.
Cite this article
Chen,B. (2024). The Impact and Future of Generative Pre-trained Transformers: A Study of GPT in Enhancing Business and Technology. Advances in Economics, Management and Political Sciences,114,215-220.
Data availability
The datasets used and/or analyzed during the current study will be available from the authors upon reasonable request.
Disclaimer/Publisher's Note
The statements, opinions and data contained in all publications are solely those of the individual author(s) and contributor(s) and not of EWA Publishing and/or the editor(s). EWA Publishing and/or the editor(s) disclaim responsibility for any injury to people or property resulting from any ideas, methods, instructions or products referred to in the content.
About volume
Volume title: Proceedings of ICEMGD 2024 Workshop: Innovative Strategies in Microeconomic Business Management
© 2024 by the author(s). Licensee EWA Publishing, Oxford, UK. This article is an open access article distributed under the terms and
conditions of the Creative Commons Attribution (CC BY) license. Authors who
publish this series agree to the following terms:
1. Authors retain copyright and grant the series right of first publication with the work simultaneously licensed under a Creative Commons
Attribution License that allows others to share the work with an acknowledgment of the work's authorship and initial publication in this
series.
2. Authors are able to enter into separate, additional contractual arrangements for the non-exclusive distribution of the series's published
version of the work (e.g., post it to an institutional repository or publish it in a book), with an acknowledgment of its initial
publication in this series.
3. Authors are permitted and encouraged to post their work online (e.g., in institutional repositories or on their website) prior to and
during the submission process, as it can lead to productive exchanges, as well as earlier and greater citation of published work (See
Open access policy for details).