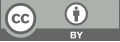
Predicting Housing Prices Using Supervised Machine Learning Models
- 1 University of Southern California
* Author to whom correspondence should be addressed.
Abstract
Variations in housing prices exert a profound impact on economic policies and personal financial decisions. This study aims to delve into the factors that affect housing prices and construct a predictive model using machine learning techniques. Machine learning enables computers to acquire knowledge from data and make predictions or decisions. This study forcasts house prices by analyzing the characteristics of data from Seattle, Washington using machine learning multiple linear regression, polynomial regression, and K-nearest neighbors regression (KNN). The findings of this investigation demonstrate that the polynomial regression model is the most accurate in predicting housing prices and can capture nonlinear relationships in the data more effectively than multiple linear regression and K-nearest neighbors regression. The main factors affecting housing prices are interior living space and the construction and design of buildings. These insights hold potential for enhancing government policies, facilitating effective land use decision-making by planners, and enabling investors to make more informed choices.
Keywords
Machine learning models, house price prediction, polynomial regression, K-nearest neighbors regression.
[1]. Hashim, Zainal Abidin. House price and affordability in housing in Malaysia. Akademika 78.1 (2010): 37-46.
[2]. Kadir Atalay, Rebecca Edwards, House prices, housing wealth and financial well-being, Journal of Urban Economics, (2022): 129. https://doi.org/10.1016/j.jue.2022.103438.
[3]. Miu, Peter, and C. Sherman Cheung. Home ownership decision in personal finance: Some empirical evidence. Financial Services Review 24.1 (2015): 51-76.
[4]. El Naqa, I, and, Murphy, M.J. What Is Machine Learning?. In: El Naqa, I., Li, R., Murphy, M. (eds) Machine Learning in Radiation Oncology. Cham: Springer. 2015. https://doi.org/10.1007/978-3-319-18305-3_1
[5]. Wang, L., Wang, G., Yu, H., and Wang, F. Prediction and analysis of residential house price using a flexible spatiotemporal model. Journal of Applied Economics, 25.1(2022): 503–22. https://doi.org/10.1080/15140326.2022.2045466
[6]. X., Cheri. Optimizations of Training Dataset on House Price Estimation, 2nd International Conference on Big Data Economy and Information Management, Sanya, China, 202: 197-203. doi: 10.1109/BDEIM55082.2021.00047.
[7]. Hastie, T., Tibshirani, R., and Friedman, J. The elements of statistical learning: Data mining, inference, and prediction (2nd ed.). Springer, 2009.
[8]. James, G., Witten, D., Hastie, T., Tibshirani, R., and Taylor, J. Linear regression. In An introduction to statistical learning: With applications in python. Cham: Springer International Publishing, 2023: 69-134.
[9]. Berrar, Daniel. Cross-validation. 2nd Edition, Encyclopedia of Bioinformatics and Computational Biology, (2019): 542-545.
[10]. B. Wang, X. Ding and F. -Y. Wang, Determination of polynomial degree in the regression of drug combinations, IEEE/CAA Journal of Automatica Sinica, 4.1(2017): 41-47. doi: 10.1109/JAS.2017.7510319.
[11]. Haiyan, Jiang, Jianzhou, Wang, Jie, Wu, and Wei, Geng. Comparison of numerical methods and metaheuristic optimization algorithms for estimating parameters for wind energy potential assessment in low wind regions, Renewable and Sustainable Energy Reviews, (69)2017: 1199-217. https://doi.org/10.1016/j.rser.2016.11.241.
[12]. Hastie, T., Tibshirani, R., and Friedman, J. The Elements of Statistical Learning: Data Mining, Inference, and Prediction. Springer, 2009.
[13]. Chicco D, Warrens MJ, and Jurman G. The coefficient of determination R-squared is more informative than SMAPE, MAE, MAPE, MSE and RMSE in regression analysis evaluation. PeerJ Computer Science, 7(2021): 623. https://doi.org/10.7717/peerj-cs.623
[14]. Chai, Tianfeng, and Roland R. Draxler. Root mean square error (RMSE) or mean absolute error (MAE). Geoscientific model development discussions, 7.1 (2014): 1525-34.
Cite this article
Zhang,H. (2024). Predicting Housing Prices Using Supervised Machine Learning Models. Advances in Economics, Management and Political Sciences,118,1-7.
Data availability
The datasets used and/or analyzed during the current study will be available from the authors upon reasonable request.
Disclaimer/Publisher's Note
The statements, opinions and data contained in all publications are solely those of the individual author(s) and contributor(s) and not of EWA Publishing and/or the editor(s). EWA Publishing and/or the editor(s) disclaim responsibility for any injury to people or property resulting from any ideas, methods, instructions or products referred to in the content.
About volume
Volume title: Proceedings of the 3rd International Conference on Financial Technology and Business Analysis
© 2024 by the author(s). Licensee EWA Publishing, Oxford, UK. This article is an open access article distributed under the terms and
conditions of the Creative Commons Attribution (CC BY) license. Authors who
publish this series agree to the following terms:
1. Authors retain copyright and grant the series right of first publication with the work simultaneously licensed under a Creative Commons
Attribution License that allows others to share the work with an acknowledgment of the work's authorship and initial publication in this
series.
2. Authors are able to enter into separate, additional contractual arrangements for the non-exclusive distribution of the series's published
version of the work (e.g., post it to an institutional repository or publish it in a book), with an acknowledgment of its initial
publication in this series.
3. Authors are permitted and encouraged to post their work online (e.g., in institutional repositories or on their website) prior to and
during the submission process, as it can lead to productive exchanges, as well as earlier and greater citation of published work (See
Open access policy for details).