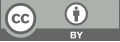
Comparative Analysis of the Effectiveness of Different Algorithms for House Price Prediction in Machine Learning
- 1 Faculty of Data Science, City University of Macau, Macau, China
* Author to whom correspondence should be addressed.
Abstract
In today's economic climate, where housing is a major focus, predicting house prices has become essential for both buyers and investors. Accurate price prediction allows for more informed decision-making, helping to minimize losses and maximize potential profits. The paper explores the different machine learning algorithms to predict house prices, utilizing the classic Boston Housing dataset from Kaggle. The study compares the performance of four algorithms: Random Forest (RF), Decision Tree (DT), Gradient Boosting (GB), and Extreme GB (XGBoost). Experimental results demonstrate that GB and XGBoost outperform the other models, delivering the highest prediction accuracy. These algorithms excel in capturing complex, nonlinear relationships in the data, making them particularly effective for house price prediction. The findings suggest the models like GB and XGBoost can enhance the accuracy of prediction, offering valuable insights into the real estate market. The study also highlights the importance of continuously refining machine learning models to adapt to changing market conditions. By improving predictive models, the study contributes to an understanding of home price dynamics, which is critical for both home buyers and real estate investors, which is crucial for both homebuyers and real estate investors.
Keywords
House Price Prediction, Machine Learning, Gradient Boosting, XGBoost
[1]. Madhuri, C. R., Anuradha, G., & Pujitha, M. V. (2019) House price prediction using regression techniques: A comparative study. In 2019 International conference on smart structures and systems, 1-5.
[2]. Ghosalkar, N. N., & Dhage, S. N. (2018) Real estate value prediction using linear regression. In 2018 fourth international conference on computing communication control and automation, 1-5.
[3]. Varma, A., Sarma, A., Doshi, S., & Nair, R. (2018) House price prediction using machine learning and neural networks. In 2018 second international conference on inventive communication and computational technologies, 1936-1939.
[4]. Phan, T. D. (2018) Housing price prediction using machine learning algorithms: The case of Melbourne city, Australia. In 2018 International conference on machine learning and data engineering, 35-42.
[5]. Soltani, A., Heydari, M., Aghaei, F., & Pettit, C. J. (2022) Housing price prediction incorporating spatio-temporal dependency into machine learning algorithms. Cities, 131, 103941.
[6]. Sibindi, R., Mwangi, R. W., & Waititu, A. G. (2023) A boosting ensemble learning based hybrid light gradient boosting machine and extreme gradient boosting model for predicting house prices. Engineering Reports, 5(4), e12599.
[7]. Armona, L., Fuster, A., & Zafar, B. (2019) Home price expectations and behaviour: Evidence from a randomized information experiment. The Review of Economic Studies, 86(4), 1371-1410.
[8]. Wu, J., Gyourko, J., & Deng, Y. (2012) Evaluating conditions in major Chinese housing markets. Regional Science and Urban Economics, 42(3), 531-543.
[9]. Natekin, A., & Knoll, A. (2013) Gradient boosting machines, a tutorial. Frontiers in neurorobotics, 7, 21.
[10]. Chen, T., & Guestrin, C. (2016) Xgboost: A scalable tree boosting system. In Proceedings of the 22nd acm sigkdd international conference on knowledge discovery and data mining, 785-794.
Cite this article
Deng,Z. (2024). Comparative Analysis of the Effectiveness of Different Algorithms for House Price Prediction in Machine Learning. Advances in Economics, Management and Political Sciences,136,101-107.
Data availability
The datasets used and/or analyzed during the current study will be available from the authors upon reasonable request.
Disclaimer/Publisher's Note
The statements, opinions and data contained in all publications are solely those of the individual author(s) and contributor(s) and not of EWA Publishing and/or the editor(s). EWA Publishing and/or the editor(s) disclaim responsibility for any injury to people or property resulting from any ideas, methods, instructions or products referred to in the content.
About volume
Volume title: Proceedings of the 3rd International Conference on Financial Technology and Business Analysis
© 2024 by the author(s). Licensee EWA Publishing, Oxford, UK. This article is an open access article distributed under the terms and
conditions of the Creative Commons Attribution (CC BY) license. Authors who
publish this series agree to the following terms:
1. Authors retain copyright and grant the series right of first publication with the work simultaneously licensed under a Creative Commons
Attribution License that allows others to share the work with an acknowledgment of the work's authorship and initial publication in this
series.
2. Authors are able to enter into separate, additional contractual arrangements for the non-exclusive distribution of the series's published
version of the work (e.g., post it to an institutional repository or publish it in a book), with an acknowledgment of its initial
publication in this series.
3. Authors are permitted and encouraged to post their work online (e.g., in institutional repositories or on their website) prior to and
during the submission process, as it can lead to productive exchanges, as well as earlier and greater citation of published work (See
Open access policy for details).