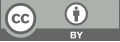
Application of SARIMA Model: Forecasting CPI and PPI Inflation Rates in the U.S.
- 1 Department of Actuarial Science and Statistics, University of Toronto, Toronto, Canada
* Author to whom correspondence should be addressed.
Abstract
This research seeks to improve the precision of future inflation rate forecasts through a comprehensive analysis of the time series data of the U.S. Consumer Price Index (CPI) and Producer Price Index (PPI). This holds significant reference value for the government when formulating economic policies, monetary policies, and fiscal decisions, especially when facing economic fluctuations or external shocks, as it can provide more accurate inflation expectations, thereby enabling the formulation of more forward-looking regulatory measures. The data for this research is sourced from the FRED database, which contains detailed historical CPI and PPI data. This research utilized the traditional SARIMA model for time series analysis and performed thorough data preprocessing and trend analysis to guarantee the stability and predictability of the data. The results indicate that the SARIMA model exhibits different effects in capturing the trend changes of CPI and PPI inflation rates: first, the SARIMA model demonstrates high accuracy in identifying and predicting the long-term trend of CPI; second, it shows stronger adaptability and accuracy in short-term predictions of PPI change rates. This indicates that different economic indicators respond differently to the model. In the future, it may be worth considering the introduction of other advanced forecasting models, such as machine learning algorithms and hybrid models, to further enhance the predictive capability regarding changes in the inflation rate, thereby providing policymakers with more precise data support.
Keywords
CPI, PPI, SARIMA model, Time series analysis
[1]. Fama, E. F., & Schwert, G. William. (1977). Asset Returns and Inflation. Journal of Financial Economics, 5(2), 115–146.
[2]. Stock, J. H., & Watson, M. W. (2003). Forecasting Output and Inflation: The Role of Asset Prices. Journal of Economic Literature, 41(3), 788–829.
[3]. Ang, A., Bekaert, G., & Wei, M. (2007). Do macro variables, asset markets, or surveys forecast inflation better? Journal of Monetary Economics, 54(4), 1163–1212.
[4]. Federal Reserve Bank of St. Louis. (2023). FRED Economic Data. Stlouisfed.org; Federal Reserve Bank of St. Louis. Retrie from https://fred.stlouisfed.org/
[5]. Hyndman, R. J., & Khandakar, Y. (2008). Automatic Time Series Forecasting: the Forecast Package for R. Journal of Statistical Software, 27(3).
[6]. Janacek, G. (2003). Non-linear Time Series Models in Empirical Finance. Journal of the Royal Statistical Society: Series D (The Statistician), 52(4), 696–696.
[7]. Qiu, J., Wang, B., & Zhou, C. (2020). Forecasting stock prices with long-short term memory neural network based on attention mechanism. PLOS ONE, 15(1), e0227222.
[8]. Moghar, A., & Hamiche, M. (2020). Stock Market Prediction Using LSTM Recurrent Neural Network. Procedia Computer Science, 170, 1168–1173.
[9]. Zhang, G. Peter. (2003). Time Series Forecasting Using a Hybrid ARIMA and Neural Network Model. Neurocomputing, 50, 159–175.
[10]. Young, P., & Shellswell, S. (1972). Time series analysis, forecasting and control. IEEE Transactions on Automatic Control, 17(2), 281–283.
Cite this article
Yao,X. (2024). Application of SARIMA Model: Forecasting CPI and PPI Inflation Rates in the U.S.. Advances in Economics, Management and Political Sciences,141,116-122.
Data availability
The datasets used and/or analyzed during the current study will be available from the authors upon reasonable request.
Disclaimer/Publisher's Note
The statements, opinions and data contained in all publications are solely those of the individual author(s) and contributor(s) and not of EWA Publishing and/or the editor(s). EWA Publishing and/or the editor(s) disclaim responsibility for any injury to people or property resulting from any ideas, methods, instructions or products referred to in the content.
About volume
Volume title: Proceedings of ICFTBA 2024 Workshop: Finance's Role in the Just Transition
© 2024 by the author(s). Licensee EWA Publishing, Oxford, UK. This article is an open access article distributed under the terms and
conditions of the Creative Commons Attribution (CC BY) license. Authors who
publish this series agree to the following terms:
1. Authors retain copyright and grant the series right of first publication with the work simultaneously licensed under a Creative Commons
Attribution License that allows others to share the work with an acknowledgment of the work's authorship and initial publication in this
series.
2. Authors are able to enter into separate, additional contractual arrangements for the non-exclusive distribution of the series's published
version of the work (e.g., post it to an institutional repository or publish it in a book), with an acknowledgment of its initial
publication in this series.
3. Authors are permitted and encouraged to post their work online (e.g., in institutional repositories or on their website) prior to and
during the submission process, as it can lead to productive exchanges, as well as earlier and greater citation of published work (See
Open access policy for details).