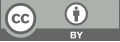
NASDAQ Forecast Based on ARIMA and ETS Models
- 1 College of Letters, Arts and Sciences, University of Southern California, Los Angeles, US
* Author to whom correspondence should be addressed.
Abstract
In the financial markets, stock price prediction has always been a classic, popular, and challenging research topic. The ability to effectively predict stock movements can allow investors to maximize returns and manage risks, which reinforces the efficiency of capital markets. Furthermore, understanding and improving prediction models contributes to the academic study of market behavior and the development of more robust financial models. However, stock prices are subject to various economic, political, and psychological factors, introducing high uncertainty and making accurate forecasting a complex endeavor. In this research, two forecasting methods will be used to forecast the NASDAQ index. Their prediction result will then be compared. The ARIMA model and the ETS model are the two forecasting models used in this study. The past 10 years (2013-2024) of NASDAQ index (open) will be used as the data for the training set and test set. The goal of this study is to compare and evaluate the accuracy of each model and determine the model that is better suited for financial forecasting. The result shows that the RMSE value of ARIMA model fitting is lower than ETS model fitting. The ARIMA model provides a better predicting result in predicting the NASDAQ index. This paper compares ARIMA and ETS models to provide insights for future investment decisions and show a new perspective on stock price forecasting.
Keywords
ARIMA, ETS, NASDAQ, Time series, Prediction
[1]. Kanwal, A., Lau, M. F., Ng, S. P. H., Sim, K. Y., & Chandrasekaran, S. (2022). BiCuDNNLSTM-1dCNN — A hybrid deep learning-based predictive model for stock price prediction. Expert Systems with Applications, 202, 117123
[2]. de Oliveira, F. A., Nobre, C. N., & Zárate, L. E. (2013). Applying Artificial Neural Networks to prediction of stock price and improvement of the directional prediction index – Case study of PETR4, Petrobras, Brazil. Expert Systems with Applications, 40(18), 7596–7606.
[3]. An, Y., & Chan, N. H. (2017). Short‐Term Stock Price Prediction Based on Limit Order Book Dynamics. Journal of Forecasting, 36(5), 541–556.
[4]. Sedighi, M., & Roodposhti, F. R. (2022). Designing a Novel Model for Stock Price Prediction Using an Integrated Multi-Stage Structure: The Case of the Bombay Stock Exchange. Australasian Accounting, Business & Finance Journal, 16(6), 70–85.
[5]. Chaudhary, A., Gupta, A., Pahariya, D., & Singh, S. K. (2023). Stock Price Prediction of Tesla & Apple using LSTM. ITM Web of Conferences, 56, 2006.
[6]. Wang, H., Wang, J., Cao, L., Li, Y., Sun, Q., & Wang, J. (2021). A Stock Closing Price Prediction Model Based on CNN-BiSLSTM. Complexity, 1.
[7]. Boru İpek, A. (2023). Stock price prediction using improved extreme learning machine methods during the Covid-19 pandemic and selection of appropriate prediction method. Kybernetes, 52(10), 4081–4109.
[8]. Alshara, M. A. (2022). Multilayer Graph-Based Deep Learning Approach for Stock Price Prediction. Security and Communication Networks, 2022, 1–8.
[9]. Lachaab, M., & Omri, A. (2023). Machine and deep learning-based stock price prediction during the COVID-19 pandemic: the case of CAC 40 index. EuroMed Journal of Business.
[10]. Jing, N., Wu, Z., & Wang, H. (2021). A hybrid model integrating deep learning with investor sentiment analysis for stock price prediction. Expert Systems with Applications, 178, 115019.
Cite this article
Cai,S. (2025). NASDAQ Forecast Based on ARIMA and ETS Models. Advances in Economics, Management and Political Sciences,144,44-51.
Data availability
The datasets used and/or analyzed during the current study will be available from the authors upon reasonable request.
Disclaimer/Publisher's Note
The statements, opinions and data contained in all publications are solely those of the individual author(s) and contributor(s) and not of EWA Publishing and/or the editor(s). EWA Publishing and/or the editor(s) disclaim responsibility for any injury to people or property resulting from any ideas, methods, instructions or products referred to in the content.
About volume
Volume title: Proceedings of ICFTBA 2024 Workshop: Finance's Role in the Just Transition
© 2024 by the author(s). Licensee EWA Publishing, Oxford, UK. This article is an open access article distributed under the terms and
conditions of the Creative Commons Attribution (CC BY) license. Authors who
publish this series agree to the following terms:
1. Authors retain copyright and grant the series right of first publication with the work simultaneously licensed under a Creative Commons
Attribution License that allows others to share the work with an acknowledgment of the work's authorship and initial publication in this
series.
2. Authors are able to enter into separate, additional contractual arrangements for the non-exclusive distribution of the series's published
version of the work (e.g., post it to an institutional repository or publish it in a book), with an acknowledgment of its initial
publication in this series.
3. Authors are permitted and encouraged to post their work online (e.g., in institutional repositories or on their website) prior to and
during the submission process, as it can lead to productive exchanges, as well as earlier and greater citation of published work (See
Open access policy for details).