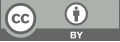
Logistics Demand Forecasting and Simulation Based on Support Vector Machine
- 1 Dalian Maritime University
* Author to whom correspondence should be addressed.
Abstract
The need for regional logistics has increased dramatically due to the logistics industry's rapid development, underscoring the need for accurate forecasting. The deployment of a precise logistics demand forecasting model has the potential to mitigate expenses, augment logistics efficacy, and furnish direction for forthcoming logistics sector planning. This research suggests an approach based on the Support Vector Machine (SVM) model to increase the accuracy of logistics demand forecasting in light of the complexity of regional logistics demand forecasting. First, in order to determine the primary factors influencing regional logistics demand, grey relational analysis is utilized. Subsequently, the sample data is input, and the Support Vector Machine model is utilized for learning, establishing a relationship model between regional logistics demand and influencing indicators. The predictions produced by a Back Propagation (BP) neural network are then contrasted with this. Lastly, data on logistics demand from Jiangsu Province covering the years 2004 to 2022 is used for simulation study. The results demonstrate that the Support Vector Machine performs better in regional logistics demand forecasting when compared to the BP neural network. The study’s findings support the advancement of scientific demand forecasting for regional logistics, offer a vital basis for decision-making in logistics system design, and strengthen the area’s potential for sustainable growth.
Keywords
Regional logistics demand forecasting, Support vector machine, BP neural network, Grey relational analysis
[1]. Gao, M., & Feng, Q. (2009). Modeling and forecasting of urban logistics demand based on support vector machine. In 2009 Second International Workshop on Knowledge Discovery and Data Mining (pp. 793-796). IEEE.
[2]. Yan, Y. (2011). Demand forecasting method in logistics management based on support vector machine. In 2011 International Conference on E-Business and E-Government (ICEE) (pp. 1-4). IEEE.
[3]. Li, H. Z., Guo, S., & Li, C. J. (2012). Fruit fly optimization and least squares support vector machine hybrid forecasting model: A case study of China's logistics demand forecasting. Economic Mathematics, 29(3), 103-106.
[4]. Zhang, H., & Xing, P. Z. (2012). Modeling and predictive simulation of regional logistics demand. Computer Simulation, 29(6), 351-354, 393.
[5]. Lü, Y. (2018). Research on regional logistics demand forecasting based on support vector machine. China Market, 2, 144-144.
[6]. Ma, Q., Sun, D., Shi, J., He, X., & Zhai, Y. (2020). Research on predicting the risk of tumor incidence in adults based on support vector machine and XGboost. Chinese General Practice, 23(12), 1486.
[7]. Sun, Z., Pan, J., Zhang, Z., Zhang, L., & Ding, Q. (2009). Traffic flow prediction based on the combination of principal component analysis and support vector machine. Journal of Highway and Transportation Research and Development (English Edition), 26(5), 127-131.
[8]. Liang, Y., & Xu, C. F. (2024). Modeling and predictive simulation of regional logistics demand based on SVM: A case study of Zhejiang Province. Logistics Research, 3, 54-60.
[9]. Yu, K. L. (2022). Research on regional logistics demand forecasting based on support vector machine. China Economic and Trade Herald, 5, 85-87.
Cite this article
Li,N. (2024). Logistics Demand Forecasting and Simulation Based on Support Vector Machine. Advances in Economics, Management and Political Sciences,139,143-148.
Data availability
The datasets used and/or analyzed during the current study will be available from the authors upon reasonable request.
Disclaimer/Publisher's Note
The statements, opinions and data contained in all publications are solely those of the individual author(s) and contributor(s) and not of EWA Publishing and/or the editor(s). EWA Publishing and/or the editor(s) disclaim responsibility for any injury to people or property resulting from any ideas, methods, instructions or products referred to in the content.
About volume
Volume title: Proceedings of the 3rd International Conference on Financial Technology and Business Analysis
© 2024 by the author(s). Licensee EWA Publishing, Oxford, UK. This article is an open access article distributed under the terms and
conditions of the Creative Commons Attribution (CC BY) license. Authors who
publish this series agree to the following terms:
1. Authors retain copyright and grant the series right of first publication with the work simultaneously licensed under a Creative Commons
Attribution License that allows others to share the work with an acknowledgment of the work's authorship and initial publication in this
series.
2. Authors are able to enter into separate, additional contractual arrangements for the non-exclusive distribution of the series's published
version of the work (e.g., post it to an institutional repository or publish it in a book), with an acknowledgment of its initial
publication in this series.
3. Authors are permitted and encouraged to post their work online (e.g., in institutional repositories or on their website) prior to and
during the submission process, as it can lead to productive exchanges, as well as earlier and greater citation of published work (See
Open access policy for details).