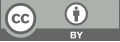
Bank Customer Churn Prediction Using Machine Learning
- 1 School of Statistics and Mathematics, Zhongnan University of Economics and Law, Wuhan, Hubei, 430073, China
* Author to whom correspondence should be addressed.
Abstract
The banking sector is fiercely competitive in the present difficult time. Banks concentrate on both customer retention and customer turnover to raise the caliber and degree of service. The classification issue in the banking sector is examined in this essay. It detects possible churners from among potential customers and primarily focuses on bank customers' worries around churn. The bank uses supervised machine learning to identify and forecast which of its clients are most likely to leave. Since it is necessary to define churn and non-churn clients, customer churn prediction can be used in this situation. To address the distinctions between churn and non-churn clients, this study uses logistic regression, decision trees, and random forest classifiers. Accuracy levels can be attained via several classifiers. The Kaggle dataset for bank customer churn modeling is used for the experiment. To identify an appropriate model with more accuracy and predictability, the outcomes are compared. The findings demonstrate that, upon oversampling, in terms of accuracy, the decision tree model outperforms other models.
Keywords
Customer churn prediction, Logistic regression, Random forest, Decision tree
[1]. Liu, D. R., & Shih, Y. Y. (2005). Integrating AHP and data mining for product recommendation based on customer lifetime value. Information & Management, 42(3), 387–400.
[2]. Canning, G. Jr. (1982). Do a value analysis of your customer base. Industrial Marketing Management, 11(2), 89–93.
[3]. Verbeke, W., Martens, D., Mues, C., & Baesens, B. (2011). Building comprehensible customer churn prediction models with advanced rule induction techniques. Expert Systems with Applications, 38(2), 2354–2364.
[4]. Capgemini. (2019). World retail banking report (last accessed on 03/28/2020). https://www.capgemini.com
[5]. PwC. (2014). Retail banking 2020: Evolution or revolution? (last accessed on 03/28/2020). https://www.pwc.com
[6]. Nie, G., Rowe, W., Zhang, L., Tian, Y., & Shi, Y. (2011). Credit card churn forecasting by logistic regression and decision tree. Expert Systems with Applications, 38(11), 15273–15285.
[7]. Bishop, C. M. (2006). Pattern recognition and machine learning (Vol. 1). Springer.
[8]. Breiman, L. (2001). Random forests. Machine Learning, 45(1), 5–32.
[9]. Quinlan, J. R. (1986). Induction of decision trees. Machine Learning, 1(1), 81–106.
[10]. Sagi, O., & Rokach, L. (2020). Explainable decision forest: Transforming a decision forest into an interpretable tree. Information Fusion, 61, 124–138.
Cite this article
Liu,Y. (2025). Bank Customer Churn Prediction Using Machine Learning. Advances in Economics, Management and Political Sciences,153,53-60.
Data availability
The datasets used and/or analyzed during the current study will be available from the authors upon reasonable request.
Disclaimer/Publisher's Note
The statements, opinions and data contained in all publications are solely those of the individual author(s) and contributor(s) and not of EWA Publishing and/or the editor(s). EWA Publishing and/or the editor(s) disclaim responsibility for any injury to people or property resulting from any ideas, methods, instructions or products referred to in the content.
About volume
Volume title: Proceedings of the 3rd International Conference on Financial Technology and Business Analysis
© 2024 by the author(s). Licensee EWA Publishing, Oxford, UK. This article is an open access article distributed under the terms and
conditions of the Creative Commons Attribution (CC BY) license. Authors who
publish this series agree to the following terms:
1. Authors retain copyright and grant the series right of first publication with the work simultaneously licensed under a Creative Commons
Attribution License that allows others to share the work with an acknowledgment of the work's authorship and initial publication in this
series.
2. Authors are able to enter into separate, additional contractual arrangements for the non-exclusive distribution of the series's published
version of the work (e.g., post it to an institutional repository or publish it in a book), with an acknowledgment of its initial
publication in this series.
3. Authors are permitted and encouraged to post their work online (e.g., in institutional repositories or on their website) prior to and
during the submission process, as it can lead to productive exchanges, as well as earlier and greater citation of published work (See
Open access policy for details).