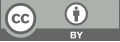
Predicting Customer Churn Rate in the Telecommunication Industry Using Machine Learning
- 1 Physical Science, University of California, Irvine, California, 92612, United States
* Author to whom correspondence should be addressed.
Abstract
The paper mainly focuses on predicting the customer churn rate by using Machine learning models. The customer churn rate becomes a serious problem because of the competitive market. Many companies care about the number of customer rates because the company wants to retain the current customers to let the current customers buy the company’s product and service. In addition, the cost of retaining an existing customer is lower than the cost of acquiring new customers. That means lots of telecommunication companies want to lower the customer attrition rate to make the company more beneficial. To find the attrition rate, companies must find the data and use models to analyze the data. The paper will use several models to analyze the data from Kaggle and find which models are better for analyzing the attrition rate by using Python. It will include several steps to analyze the data: Exploratory Data Analysis, Feature Engineering, Feature Selection, Model Tuning, Stacking model, and Final analysis and results. Lastly, the result showed that these models don’t overfit and it concluded that the logistic regression with the upsampling method is better than other models.
Keywords
Customer Churn, Telecommunication Industry, Logistic Regression
[1]. Sabbeh, S. F. (2018). Machine-learning techniques for customer retention: A comparative study. International Journal of advanced computer Science and applications, 9(2).
[2]. Ahn, J. H., Han, S. P., & Lee, Y. S. (2006). Customer churn analysis: Churn determinants and mediation effects of partial defection in the Korean mobile telecommunications service industry. Telecommunications policy, 30(10-11), 552-568.
[3]. Huang, B., Kechadi, M. T., & Buckley, B. (2012). Customer churn prediction in telecommunications. Expert Systems with Applications, 39(1), 1414-1425.
[4]. Sahoo, K., Samal, A. K., Pramanik, J., & Pani, S. K. (2019). Exploratory data analysis using Python. International Journal of Innovative Technology and Exploring Engineering, 8(12), 4727-4735.
[5]. Verdonck, T., Baesens, B., Óskarsdóttir, M., & vanden Broucke, S. (2024). Special issue on feature engineering editorial. Machine learning, 113(7), 3917-3928.
[6]. Dahouda, M. K., & Joe, I. (2021). A deep-learned embedding technique for categorical features encoding. IEEE Access, 9, 114381-114391.
[7]. Jain, H., Khunteta, A., & Srivastava, S. (2020). Churn prediction in telecommunication using LR and logit boost. Procedia Computer Science, 167, 101-112.
[8]. Lalwani, P., Mishra, M. K., Chadha, J. S., & Sethi, P. (2022). Customer churn prediction system: a machine learning approach. Computing, 104(2), 271-294.
[9]. Seyedzadeh, S., Rahimian, F. P., Rastogi, P., & Glesk, I. (2019). Tuning machine learning models for prediction of building energy loads. Sustainable Cities and Society, 47, 101484.
[10]. Bi, Q., Goodman, K. E., Kaminsky, J., & Lessler, J. (2019). What is machine learning? A primer for the epidemiologist. American journal of epidemiology, 188(12), 2222-2239.
[11]. Soni, B. (2023). Stacking to improve model performance: A comprehensive guide on ensemble learning in python. Medium. https://medium.com/@brijesh_soni/stacking-to-improve-model-performance-a-comprehensive-guide-on-ensemble-learning-in-python-9ed53c93ce28.
[12]. Prakathi. (2023). Predicting employee attrition: Comparing LR and decision tree models. Medium. https:// medium.com/@prakathi/predicting-employee-attrition-comparing-logistic-regression-and-decision-tree-models-b76748b36ed4#:~:text=Logistic%20Regression%20is%20a%20straightforward%20and%20interpretable%20model,impact%20of%20individual%20features%20on%20the%20attrition%20prediction.
Cite this article
Zhang,Z. (2025). Predicting Customer Churn Rate in the Telecommunication Industry Using Machine Learning. Advances in Economics, Management and Political Sciences,153,70-76.
Data availability
The datasets used and/or analyzed during the current study will be available from the authors upon reasonable request.
Disclaimer/Publisher's Note
The statements, opinions and data contained in all publications are solely those of the individual author(s) and contributor(s) and not of EWA Publishing and/or the editor(s). EWA Publishing and/or the editor(s) disclaim responsibility for any injury to people or property resulting from any ideas, methods, instructions or products referred to in the content.
About volume
Volume title: Proceedings of the 3rd International Conference on Financial Technology and Business Analysis
© 2024 by the author(s). Licensee EWA Publishing, Oxford, UK. This article is an open access article distributed under the terms and
conditions of the Creative Commons Attribution (CC BY) license. Authors who
publish this series agree to the following terms:
1. Authors retain copyright and grant the series right of first publication with the work simultaneously licensed under a Creative Commons
Attribution License that allows others to share the work with an acknowledgment of the work's authorship and initial publication in this
series.
2. Authors are able to enter into separate, additional contractual arrangements for the non-exclusive distribution of the series's published
version of the work (e.g., post it to an institutional repository or publish it in a book), with an acknowledgment of its initial
publication in this series.
3. Authors are permitted and encouraged to post their work online (e.g., in institutional repositories or on their website) prior to and
during the submission process, as it can lead to productive exchanges, as well as earlier and greater citation of published work (See
Open access policy for details).