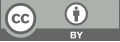
A Hybrid Data Decomposition and Deep Learning Approach for Solana Price Prediction Incorporating Market Factors
- 1 Vanderbilt University, Nashville, Tennessee, USA, 37235
* Author to whom correspondence should be addressed.
Abstract
In this study, a hybrid data decomposition model for predicting the Solana (SOL) price is proposed, integrated by advanced signal processing and machine learning techniques. The model utilizes Variational Mode Decomposition (VMD) to decompose the Solana price series into 10 intrinsic modes, each representing different frequency components of the data. These 10 decomposed modes are then used as features, along with other relevant market factors, including Solana volume, halving index, Solana trend, and Bitcoin closing price. Then, the Bidirectional Long Short-Term Memory (BiLSTM) network is chosen to capture the complex temporal dependencies in these features and forecast future prices. To evaluate the efficacy of the approach, this study compares the hybrid model’s performance with a baseline LSTM model, which uses only raw historical price data. The results demonstrate that the hybrid model outperforms the benchmark model, showing higher predictive accuracy and robustness. A trading simulation for models was then conducted, and the findings indicate a significant potential for the experiment model to function as an effective trading support system, reinforcing its applicability in practical market environments. This work contributes to the field of cryptocurrency price prediction by offering a more sophisticated methodology that combines time series decomposition with deep learning techniques, providing valuable insights for traders and investors.
Keywords
data decomposition, Solana market factors, Solana price prediction, machine learning
[1]. Yakovenko, A. (2020). Solana: A new architecture for high throughput blockchain. Solana Labs.
[2]. Solana Foundation. (2021). Solana whitepaper. Solana Foundation.
[3]. Sharma, P., Jain, R., Kapoor, A. (2020). Cryptocurrency Price Prediction Using Machine Learning. Computational Economics, 56(4), 945-963.
[4]. Zhang, Y., Chen, X., Zhao, J. (2021). Predicting Bitcoin Price Movement Using Machine Learning: A Comparative Study. Journal of Computational Finance, 22(2), 1-24.
[5]. Jiang, Z., Wang, Y., Li, Z. (2022). A Hybrid Deep Learning Approach for Cryptocurrency Price Prediction. Journal of Financial Engineering, 11(3), 347-362.
[6]. Dragomiretskiy, K., Zosso, D. (2014). Variational mode decomposition. IEEE Transactions on Signal Processing, 62(3), 531–544.
[7]. Zhang, L., Zhao, Y., Zhang, X. (2019). Variational mode decomposition and its application in signal processing. Signal Processing, 159, 47-58.
[8]. Wu, Z., Huang, N. E. (2009). Ensemble empirical mode decomposition: A noise-assisted data analysis method. Advanced Modeling and Analysis of Complex Systems, 7(3), 1-24.
[9]. Zhang, L., Liu, L., Zhang, L. (2020). A hybrid model based on VMD and LSTM for financial time series prediction. Applied Soft Computing, 94, 106477.
[10]. Corbet, S., Meegan, A., Larkin, C., Lucey, B., & Yarovaya, L. (2018). Exploring the dynamic relationships between cryptocurrencies and other financial assets. Economics Letters, 165, 28-34.
[11]. Halaburda, H., Gandal, N. (2014). The Role of Cryptocurrencies in the Future of Financial Systems. Journal of Financial Regulation and Compliance, 22(4), 297-308.
[12]. Hochreiter, S., Schmidhuber, J. (1997). Long short-term memory. Neural Computation, 9(8), 1735–1780.
Cite this article
Qiu,J. (2025). A Hybrid Data Decomposition and Deep Learning Approach for Solana Price Prediction Incorporating Market Factors. Advances in Economics, Management and Political Sciences,162,6-14.
Data availability
The datasets used and/or analyzed during the current study will be available from the authors upon reasonable request.
Disclaimer/Publisher's Note
The statements, opinions and data contained in all publications are solely those of the individual author(s) and contributor(s) and not of EWA Publishing and/or the editor(s). EWA Publishing and/or the editor(s) disclaim responsibility for any injury to people or property resulting from any ideas, methods, instructions or products referred to in the content.
About volume
Volume title: Proceedings of the 4th International Conference on Business and Policy Studies
© 2024 by the author(s). Licensee EWA Publishing, Oxford, UK. This article is an open access article distributed under the terms and
conditions of the Creative Commons Attribution (CC BY) license. Authors who
publish this series agree to the following terms:
1. Authors retain copyright and grant the series right of first publication with the work simultaneously licensed under a Creative Commons
Attribution License that allows others to share the work with an acknowledgment of the work's authorship and initial publication in this
series.
2. Authors are able to enter into separate, additional contractual arrangements for the non-exclusive distribution of the series's published
version of the work (e.g., post it to an institutional repository or publish it in a book), with an acknowledgment of its initial
publication in this series.
3. Authors are permitted and encouraged to post their work online (e.g., in institutional repositories or on their website) prior to and
during the submission process, as it can lead to productive exchanges, as well as earlier and greater citation of published work (See
Open access policy for details).