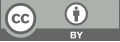
Gold Futures Price Forecast Based on Artificial intelligence
- 1 Woodsworth College, University of Toronto, Toronto, Ontario, Canada
* Author to whom correspondence should be addressed.
Abstract
Gold futures prices are affected by many factors such as macroeconomics, market supply and demand, and financial markets. Their volatility is crucial to investment decisions and market stability. However, the complexity of the market makes it difficult for traditional forecasting methods to accurately capture the law of price changes. It is of great significance to study efficient forecasting methods. This paper systematically reviews the factors affecting gold futures prices and forecasting methods. The key driving factors are analyzed from the aspects of inflation rate, interest rate, crude oil price, and US dollar index, and three main forecasting methods are summarized: traditional time series models (ARIMA, GARCH, ARDL), hybrid models (MS-MIDAS-CJ, VMD-ICSS-BiGRU) and deep learning methods (SGRU-AM, DBN). Finally, this paper explores the optimization directions of model computational efficiency, generalization ability, and market sentiment integration. This study provides a systematic analysis of gold futures market forecasting. In the future, the combination of deep learning and market sentiment analysis is expected to improve forecasting accuracy and provide support for investors and market decision-making.
Keywords
Gold futures, Prices, Forecasts, Gold
[1]. Zhu, X. H., Zou, J. W., Han, F. Y., & Chen, J. Y. (2018). Modeling and forecasting of high-frequency volatility in China's copper futures market with external shocks. Chinese Journal of Management Science, 26(9), 52-61.
[2]. Chen Xiaodong. (2014). Comparative study of high-frequency volatility prediction models in China's metal futures market. Financial Theory and Practice, (1), 73-79.
[3]. Chen Kaijie, Tang Zhenpeng, Wu Junchuan, Zhang Tingting, & Du Xiaoxu. (2022). Precious metal futures price forecasting method and empirical research. Chinese Journal of Management Science, 30(12), 245-253.
[4]. Kowalewski, P., & Skopiec, D. A. (2024). Price processes in the global gold market. See https://www. bankandcredit. nbp. pl/content/2024/04/BIK_04_2024_01. pdf.
[5]. Liang, C., Wei, Y., Ma, F., & Li, X. (2022). Forecasting volatility of China gold futures price: New evidence from model shrinkage methods. Chinese Journal of Management Science, 30(4), 30-41.
[6]. Wang, J., Li, Y., Wang, T., Li, J., Wang, H., & Liu, P. (2021). A gold futures price forecast model based on SGRU-AM. IEEE Access, 9, 146745-146754.
[7]. Madziwa, L., Pillalamarry, M., & Chatterjee, S. (2022). Gold price forecasting using multivariate stochastic model. Resources Policy, 76, 102544.
[8]. Zhang, P., & Ci, B. (2020). Deep belief network for gold price forecasting. Resources Policy, 69, 101806.
[9]. Li, Y., Wang, S., Wei, Y., & Zhu, Q. (2021). A new hybrid VMD-ICSS-BiGRU approach for gold futures price forecasting and algorithmic trading. IEEE Transactions on Computational Social Systems, 8(6), 1357-1368.
[10]. Guo Yangli, & Ma Feng. (2024). Research on the prediction of gold futures market volatility based on Markov and mixed data models. Chinese Journal of Management Science, 32(1), 13-22.
Cite this article
He,T. (2025). Gold Futures Price Forecast Based on Artificial intelligence. Advances in Economics, Management and Political Sciences,174,27-33.
Data availability
The datasets used and/or analyzed during the current study will be available from the authors upon reasonable request.
Disclaimer/Publisher's Note
The statements, opinions and data contained in all publications are solely those of the individual author(s) and contributor(s) and not of EWA Publishing and/or the editor(s). EWA Publishing and/or the editor(s) disclaim responsibility for any injury to people or property resulting from any ideas, methods, instructions or products referred to in the content.
About volume
Volume title: Proceedings of the 3rd International Conference on Management Research and Economic Development
© 2024 by the author(s). Licensee EWA Publishing, Oxford, UK. This article is an open access article distributed under the terms and
conditions of the Creative Commons Attribution (CC BY) license. Authors who
publish this series agree to the following terms:
1. Authors retain copyright and grant the series right of first publication with the work simultaneously licensed under a Creative Commons
Attribution License that allows others to share the work with an acknowledgment of the work's authorship and initial publication in this
series.
2. Authors are able to enter into separate, additional contractual arrangements for the non-exclusive distribution of the series's published
version of the work (e.g., post it to an institutional repository or publish it in a book), with an acknowledgment of its initial
publication in this series.
3. Authors are permitted and encouraged to post their work online (e.g., in institutional repositories or on their website) prior to and
during the submission process, as it can lead to productive exchanges, as well as earlier and greater citation of published work (See
Open access policy for details).