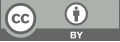
Technology Industry Stock Price Prediction Based on OLS, Random Forest, and Extreme Gradient Boosting
- 1 University of Wisconsin-Madison
* Author to whom correspondence should be addressed.
Abstract
Contemporarily, the marriage of artificial computer intelligence and the financial stock market has gained increasing interest in recent years. In recent years, forecasting stock prices has also been a more prevalent topic of conversation. Investors lack a coherent knowledge of the model mechanism and prediction results behind stock price forecasts. Hence, this paper will examine Apple, Microsoft, and Amazon, the three largest technology businesses. The three models OLS, Random Forest, and XGBoost were used to predict and evaluate historical data from the past five years. The OLS model has a superior performance structure when dealing with data sets with low data frequency, and its anticipated outcomes are also more accurate, according to the research. In addition, different machine learning models are employed for diverse data sets to produce predictions, hence enhancing the accuracy and dependability of the future predictions. Overall, these results shed light on guiding further exploration of investor investments in stocks and researcher studies theories and models.
Keywords
stock market prediction, machine learning, OLS, random forest, extreme gradient boosting
[1]. Ferreira, F. G., Gandomi, A. H., Cardoso, R. T.: Artificial intelligence applied to stock market trading: a review. IEEE Access, 9, 30898-30917 (2021).
[2]. Zhang, F.: Extreme learning machine for stock price prediction. The International Journal of Electrical Engineering & Education, 0020720920984675 (2021).
[3]. Official website for academic of NSSD. Retrieved from: https://www.nssd.cn/html/1/156/159/index.html?lngId=JGLLYSJ2022002027, last accessed 2023/2/21.
[4]. Bansal, M., Goyal, A., & Choudhary, A.: Stock Market Prediction with High Accuracy using Machine Learning Techniques. Procedia Computer Science, 215, 247-265 (2022).
[5]. Henrique, B. M., Sobreiro, V. A., Kimura, H.: Literature review: Machine learning techniques applied to financial market prediction. Expert Systems with Applications, 124, 226-251 (2019).
[6]. Vijh, M., Chandola, D., Tikkiwal, V. A., Kumar, A.: Stock closing price prediction using machine learning techniques. Procedia computer science, 167, 599-606 (2020).
[7]. Speiser, J. L., Miller, M. E., Tooze, J., Ip, E.: A comparison of random forest variable selection methods for classification prediction modeling. Expert systems with applications, 134, 93-101 (2019).
[8]. Fernández-Delgado, M., Cernadas, E., Barro, S., Amorim, D.: Do we need hundreds of classifiers to solve real world classification problems? The journal of machine learning research, 15(1), 3133-3181 (2014).
[9]. Yu, S., Tian, L., Liu, Y., Guo, Y.: LSTM-XGBoost Application of the Model to the Prediction of Stock Price. In Artificial Intelligence and Security: 7th International Conference, ICAIS 2021, Dublin, Ireland, July 19–23, 2021, Proceedings, Part I 7 (pp. 86-98). Springer International Publishing, (2021).
[10]. Gupta, R., Chen, M.: Sentiment analysis for stock price prediction. In 2020 IEEE conference on multimedia information processing and retrieval (MIPR) (pp. 213-218). IEEE (2020, August).
Cite this article
Guo,C. (2023). Technology Industry Stock Price Prediction Based on OLS, Random Forest, and Extreme Gradient Boosting. Advances in Economics, Management and Political Sciences,22,1-8.
Data availability
The datasets used and/or analyzed during the current study will be available from the authors upon reasonable request.
Disclaimer/Publisher's Note
The statements, opinions and data contained in all publications are solely those of the individual author(s) and contributor(s) and not of EWA Publishing and/or the editor(s). EWA Publishing and/or the editor(s) disclaim responsibility for any injury to people or property resulting from any ideas, methods, instructions or products referred to in the content.
About volume
Volume title: Proceedings of the 2023 International Conference on Management Research and Economic Development
© 2024 by the author(s). Licensee EWA Publishing, Oxford, UK. This article is an open access article distributed under the terms and
conditions of the Creative Commons Attribution (CC BY) license. Authors who
publish this series agree to the following terms:
1. Authors retain copyright and grant the series right of first publication with the work simultaneously licensed under a Creative Commons
Attribution License that allows others to share the work with an acknowledgment of the work's authorship and initial publication in this
series.
2. Authors are able to enter into separate, additional contractual arrangements for the non-exclusive distribution of the series's published
version of the work (e.g., post it to an institutional repository or publish it in a book), with an acknowledgment of its initial
publication in this series.
3. Authors are permitted and encouraged to post their work online (e.g., in institutional repositories or on their website) prior to and
during the submission process, as it can lead to productive exchanges, as well as earlier and greater citation of published work (See
Open access policy for details).