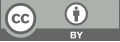
Mobile Phone Price Range Prediction Based on Machine Learning Algorithms
- 1 Beijing University of Posts and Telecommunications
* Author to whom correspondence should be addressed.
Abstract
Mobile phones with different price points on the market have different parameter configurations, which provide a lot of information related to the price. Mobile phone prices are also the focus of attention of the mobile phone market, manufacturers and consumers. This study used machine learning technology to investigate the issues in mobile phone sales, mainly the prediction of mobile phone price range and the feature contribution of the price range. The data resource includes the release price of mobile phones in the European market from 2018 to 2021. The data augmentation technologies are also applied in this study. Among the three established machine learning models, the accuracy rate of the training set of the Catboost model exceeds 80%, which is the model with the highest accuracy rate. The accuracy rate of Bagging Classifier is slightly lower than that of CatBoost, but the prediction result is the most stable. Such a result conforms to the characteristics of the algorithm itself. The conclusion of the analysis of feature contribution of numerical data to accuracy rate is that rear camera pixels, number of cameras and screen size have negligible impact on model prediction accuracy, which means feature contribution is minimal. The research results are reasonable and can provide references for merchants to set prices and for users to purchase mobile phones.
Keywords
mobile phone price prediction, feature analysis, bagging classifier, CatBoost, naive bayes
[1]. Sata, M. (2013). Factors affecting consumer buying behavior of mobile phone devices. Mediterranean Journal of Social Sciences, 4(12): 103.
[2]. Mihir, H., Patel, M. I., Jani, S., et al. (2021) Diamond price prediction using machine learning, 2021 2nd International Conference on Communication, Computing and Industry 4.0 (C2I4). IEEE, 1-5.
[3]. Muhammad, A., & Zafar, Khan. (2018). Mobile Price Class prediction using Machine Learning Techniques, International Journal of Computer Applications, 179(29): 6-11. doi: 10.5120 /ijca2018916555.
[4]. Nti, I. K., Adekoya, A. F., Weyori, B. A. (2020) A comprehensive evaluation of ensemble learning for stock-market prediction. Journal of Big Data, 2020, 7(1): 1-40.
[5]. Nasser, I. M., Al-Shawwa, M. O., Abu-Naser, S. S (2019) Developing Artificial Neural Network for Predicting Mobile Phone Price Range.
[6]. Çetın, M., & Koç. Y. (2021) Mobile Phone Price Class Prediction Using Different Classification Algorithms with Feature Selection and Parameter Optimization, 2021 5th International Symposium on Multidisciplinary Studies and Innovative Technologies (ISMSIT), Ankara, Turkey, pp. 483-487.
[7]. Kaggle (2023) Mobile Phone Price https://www.kaggle.com/datasets/rkiattisak/mobile-phone-price/code
[8]. Navid, K., Annan Z., et al. (2021) Improved prediction of slope stability using a hybrid stacking ensemble method based on finite element analysis and field data, Journal of Rock Mechanics and Geotechnical Engineering, Volume 13, Issue 1, Pages 188-201. ISSN 1674-7755.
[9]. Luo, M., et al. (2021) Combination of Feature Selection and CatBoost for Prediction: The First Application to the Estimation of Aboveground Biomass, Forests, 12: 216. doi:10.3390/f12020216.
[10]. Gao, H., et al. (2019). Application of improved distributed naive Bayesian algorithms in text classification, Journal of Supercomputing, 75: 5831-5847. doi:10.1007/s11227-019-02862-1.
[11]. Xu, J., et al. (2018) A survey of ensemble learning approaches (in Chinese). Journal of Yunnan University (Natural Sciences Edition), 40(06): 1082-1092.
Cite this article
Wang,J. (2023). Mobile Phone Price Range Prediction Based on Machine Learning Algorithms. Advances in Economics, Management and Political Sciences,46,260-268.
Data availability
The datasets used and/or analyzed during the current study will be available from the authors upon reasonable request.
Disclaimer/Publisher's Note
The statements, opinions and data contained in all publications are solely those of the individual author(s) and contributor(s) and not of EWA Publishing and/or the editor(s). EWA Publishing and/or the editor(s) disclaim responsibility for any injury to people or property resulting from any ideas, methods, instructions or products referred to in the content.
About volume
Volume title: Proceedings of the 2nd International Conference on Financial Technology and Business Analysis
© 2024 by the author(s). Licensee EWA Publishing, Oxford, UK. This article is an open access article distributed under the terms and
conditions of the Creative Commons Attribution (CC BY) license. Authors who
publish this series agree to the following terms:
1. Authors retain copyright and grant the series right of first publication with the work simultaneously licensed under a Creative Commons
Attribution License that allows others to share the work with an acknowledgment of the work's authorship and initial publication in this
series.
2. Authors are able to enter into separate, additional contractual arrangements for the non-exclusive distribution of the series's published
version of the work (e.g., post it to an institutional repository or publish it in a book), with an acknowledgment of its initial
publication in this series.
3. Authors are permitted and encouraged to post their work online (e.g., in institutional repositories or on their website) prior to and
during the submission process, as it can lead to productive exchanges, as well as earlier and greater citation of published work (See
Open access policy for details).