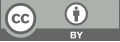
House Prices Prediction – Advanced Regression Techniques
- 1 International College, Beijing University of Posts and Telecommunications, Beijing, China
* Author to whom correspondence should be addressed.
Abstract
In recent years, with the development of the real estate industry, housing prices have continued to rise. The nation, society, and individuals are all concerned about these prices. For commodity housing prices, there are many factors that influence the housing prices. Apart from national regulations, factors such as lighting, layout, and environment of the houses themselves also have a certain impact on the prices, leading to significant fluctuations in the real estate market. Therefore, researching an accurate model for predicting housing prices has practical significance. It can guide residents in housing consumption and provide policy recommendations for government price regulation. Machine learning methods have become a new type of prediction method in this regard. Based on the theories of data analysis and machine learning, a dataset consisting of 2920 data points with 81 attributes was selected from the publicly available Kaggle housing dataset. The data was normalized and analyzed for feature selection. The ranking of attributes most correlated with housing prices was obtained. Subsequently, a neural network model was built, parameters were adjusted, and the trained network was used to predict housing prices. On the Kaggle leaderboard, the RMSE test result stands at 0.1198, positioning our model among the top performers among all machine learning methods.
Keywords
house prices predict, machine learning, regression problem
[1]. Jiang, J., & Liu, Y. (2016). A comparative study of machine learning methods for housing price prediction. Expert Systems with Applications, 60, 93-106.
[2]. Al-Mezel, S., Al-Dawood, M., & Al-Ajlan, S. (2012). Predicting House Prices Using Multiple Linear Regression Models. International Journal of Computer Science Issues (IJCSI), 9(2), 1-5.
[3]. Pramanik, S., Chow, U. N., Pram, B. K., & et al. (2010). A Comparative Study of Bagging, Boosting and C4.5: The Recent Improvements in Decision Tree Learning Algorithm. Asian Journal of Information Technology, 9(6), 300-306.
[4]. Tahir, Y., Tawab, T., Javed, S., & Naeem, M. (2018). House Price Prediction Using Machine Learning Techniques. International Journal of Computer Science and Network Security (IJCSNS), 18(1), 30-35.
[5]. Gu, X., & Li, C. (2012). House Price Prediction Research Based on Markov Chain. Consumer Economics, 2012(5), 4.
[6]. Hilber, C. A., & Vermeulen, W. (2016). The impact of supply constraints on house prices in England. The Economic Journal, 126(591), 358-405.
[7]. Gyourko, J., Mayer, C., & Sinai, T. (2013). Superstar cities. American Economic Journal: Economic Policy, 5(4), 167-199.
[8]. Dreger, C., & Kholodilin, K. A. (2013). An Early Warning System to Predict the House Price Bubbles. Social Science Electronic Publishing, 7(2013-8), 1-26. DOI:10.2139/ssrn.1898561.
[9]. Hayes, A. F. . (2013). Introduction to Mediation, Moderation, and Conditional Process Analysis: A Regression-Based Approach.
[10]. Breiman, L. I. , Friedman, J. H. , Olshen, R. A. , & Stone, C. J. . (2015). Classification and regression trees. Encyclopedia of Ecology, 57(3), 582-588.
[11]. Montoya, A., DataCanary, & et al. (2016). House Prices - Advanced Regression Techniques [Z]. Retrieved from https://kaggle.com/competitions/house-prices-advanced-regression-techniques.
Cite this article
Zong,Y. (2023). House Prices Prediction – Advanced Regression Techniques. Advances in Economics, Management and Political Sciences,50,181-189.
Data availability
The datasets used and/or analyzed during the current study will be available from the authors upon reasonable request.
Disclaimer/Publisher's Note
The statements, opinions and data contained in all publications are solely those of the individual author(s) and contributor(s) and not of EWA Publishing and/or the editor(s). EWA Publishing and/or the editor(s) disclaim responsibility for any injury to people or property resulting from any ideas, methods, instructions or products referred to in the content.
About volume
Volume title: Proceedings of the 2nd International Conference on Financial Technology and Business Analysis
© 2024 by the author(s). Licensee EWA Publishing, Oxford, UK. This article is an open access article distributed under the terms and
conditions of the Creative Commons Attribution (CC BY) license. Authors who
publish this series agree to the following terms:
1. Authors retain copyright and grant the series right of first publication with the work simultaneously licensed under a Creative Commons
Attribution License that allows others to share the work with an acknowledgment of the work's authorship and initial publication in this
series.
2. Authors are able to enter into separate, additional contractual arrangements for the non-exclusive distribution of the series's published
version of the work (e.g., post it to an institutional repository or publish it in a book), with an acknowledgment of its initial
publication in this series.
3. Authors are permitted and encouraged to post their work online (e.g., in institutional repositories or on their website) prior to and
during the submission process, as it can lead to productive exchanges, as well as earlier and greater citation of published work (See
Open access policy for details).