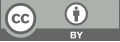
Comparison of ARIMA and LSTM in Different Industries
- 1 Shenzhen University
* Author to whom correspondence should be addressed.
Abstract
In the realm of finance, stock price prediction holds significant importance, and the breakthroughs in deep learning have provided new solutions to this problem. This study compares deep learning methods with traditional time series models by employing a time series model, namely Arima, and the neural network LSTM model to predict the closing stock data of Pfizer Inc. (PFE) in the healthcare sector, Alibaba Group Holding Limited in the e-commerce sector, EA Sports in the gaming industry, and NVIDIA Corporation in the AI industry over the past year. The aim is to identify the most suitable prediction method for each company in stock price forecasting. Through the comparison, it is observed that, regardless of the company, ARIMA outperforms LSTM in stock price prediction. Therefore, it can be concluded that, among these two models, ARIMA is a better fit for stock price prediction in these four companies. Furthermore, it is inferred that this method may also be applicable to other companies within the respective industries. However, further research and data collection are necessary to validate and support this inference. Overall, this study demonstrates the superiority of the ARIMA model over LSTM in stock price prediction for the selected companies in the healthcare, e-commerce, gaming, and AI industries.
Keywords
Arima, LSTM, MSE, stock price predictions
[1]. Upadhyay, A., Bandyopadhyay, G., and Dutta, A. (2012) Forecasting stock performance in indian market using multinomial logistic regression. Journal of Business Studies Quarterly, 3(3), 16.
[2]. Dutta, A., Bandopadhyay, G., and Sengupta, S. (2012) Prediction of stock performance in the Indian stock market using logistic regression. International Journal of Business and Information, 7(1), 105.
[3]. Zhong, X., and Enke, D. (2017) Forecasting daily stock market return using dimensionality reduction. Expert systems with applications, 67, 126-139.
[4]. Hiransha, M., Gopalakrishnan, E. A., Menon, V. K., and Soman, K. P. (2018). NSE stock market prediction using deep-learning models. Procedia computer science, 132, 1351-1362.
[5]. Adebiyi, A. A., Adewumi, A. O., and Ayo, C. K. (2014) Comparison of ARIMA and artificial neural networks models for stock price prediction. Journal of Applied Mathematics.
[6]. Jarrett, J. E., and Kyper, E. (2011). ARIMA modeling with intervention to forecast and analyze Chinese stock prices. International Journal of Engineering Business Management, 3, 17.
[7]. Ravikumar, S., and Saraf, P. (2020) Prediction of stock prices using machine learning (regression, classification) Algorithms. In 2020 International Conference for Emerging Technology, 1-5.
[8]. Patel, J., Shah, S., Thakkar, P., and Kotecha, K. (2015) Predicting stock and stock price index movement using trend deterministic data preparation and machine learning techniques. Expert systems with applications, 42(1), 259-268.
[9]. Lapedes, A., and Farber, R. (1987) Nonlinear signal processing using neural networks: Prediction and system modelling (No. LA-UR-87-2662; CONF-8706130-4).
[10]. Baştanlar, Y., and Özuysal, M. (2014) Introduction to machine learning. miRNomics: MicroRNA biology and computational analysis, 105-128.
[11]. Hastie, T., Tibshirani, R., Friedman, J. H., and Friedman, J. H. (2009) The elements of statistical learning: data mining, inference, and prediction, 2, 1-758. New York: springer.
Cite this article
Li,H. (2024). Comparison of ARIMA and LSTM in Different Industries. Advances in Economics, Management and Political Sciences,57,294-302.
Data availability
The datasets used and/or analyzed during the current study will be available from the authors upon reasonable request.
Disclaimer/Publisher's Note
The statements, opinions and data contained in all publications are solely those of the individual author(s) and contributor(s) and not of EWA Publishing and/or the editor(s). EWA Publishing and/or the editor(s) disclaim responsibility for any injury to people or property resulting from any ideas, methods, instructions or products referred to in the content.
About volume
Volume title: Proceedings of the 2nd International Conference on Financial Technology and Business Analysis
© 2024 by the author(s). Licensee EWA Publishing, Oxford, UK. This article is an open access article distributed under the terms and
conditions of the Creative Commons Attribution (CC BY) license. Authors who
publish this series agree to the following terms:
1. Authors retain copyright and grant the series right of first publication with the work simultaneously licensed under a Creative Commons
Attribution License that allows others to share the work with an acknowledgment of the work's authorship and initial publication in this
series.
2. Authors are able to enter into separate, additional contractual arrangements for the non-exclusive distribution of the series's published
version of the work (e.g., post it to an institutional repository or publish it in a book), with an acknowledgment of its initial
publication in this series.
3. Authors are permitted and encouraged to post their work online (e.g., in institutional repositories or on their website) prior to and
during the submission process, as it can lead to productive exchanges, as well as earlier and greater citation of published work (See
Open access policy for details).