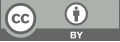
Loan Prediction Using Machine Learning Methods
- 1 West Vancouver Secondary School, West Vancouver, BC, Canada
- 2 College of Information Engineering, Zhejiang University of technology, Wenzhou, China
- 3 College of Information Engineering, Shenzhen University, Shenzhen, China
* Author to whom correspondence should be addressed.
Abstract
Credit risk has always been the most important risk faced by commercial banks. Credit risk management has important practical significance for preventing credit risk. With the emerging of machine learning algorithms, numerous frameworks, including linear regression, support vector machine, random forest and decision tree are proposed with satisfying performance and robust accuracy. This paper will focus on predicting credit outcomes and calculating forecast accuracy from a given dataset. This paper adopts three algorithms, decision tree, random forest and logistic regression, to calculate the dataset from the Bank of Portugal separately and obtain relevant conclusions. Finally, the authors evaluate the advantages and disadvantages of the three methods according to the accuracy of the prediction results, and the conclusion is described as follow, First, all three methods have great potential on handling loan prediction task. Second, the logistic regression algorithm is the most accurate, which obtains 86.4% accuracy.
Keywords
Loan prediction, Machine learning, Logistic regression
[1]. Li Yujia, & Lu Jun. (2007). Risk Premium, Expected loss and forecast loan loss Provision. Contemporary Finance and Economics (12), 7.
[2]. Jiang Zuobin, Xie Shuangqin, & Zhang Huan. (2010). Application of Arima model in the prediction of bank loan scale. Finance and Economics (7), 3.
[3]. Chen Xulan, Han Suwan, & Pang Jianhua. (2021). Enterprise loan default risk prediction based on machine learning method. Modeling and Simulation, 010(003), P.890-897.
[4]. Li Yunmeng, & Qian Xin. (2011). Long-term consumer loan forecasting based on co-integration and arma model. Statistics and Decision (11), 3.
[5]. Xiang Weixing, Li Hongjin, & Bai Dafan. (2007). Bank Credit Expansion and Real Estate Bubble: Lessons from the United States, Japan, and East Asian Countries and Regions. International Studies of Finance (3), 7.
[6]. Goodhart, C. , & Hofmann, B. . (2004). Monetary transmission in simple backward-looking models: the is puzzle.
[7]. Goyal, A., & Kaur, R. (2016). A survey on ensemble model for loan prediction. International Journal of Engineering Trends and Applications (IJETA), 3(1), 32-37.
[8]. Arun, K., Ishan, G., & Sanmeet, K. (2016). Loan approval prediction based on machine learning approach. IOSR J. Comput. Eng, 18(3), 18-21.
[9]. Gupta, A., Pant, V., Kumar, S., & Bansal, P. K. (2020, December). Bank Loan Prediction System using Machine Learning. In 2020 9th International Conference System Modeling and Advancement in Research Trends (SMART) (pp. 423-426). IEEE.
[10]. Ratadiya, P., Asawa, K., & Nikhal, O. (2020). A decentralized aggregation mechanism for training deep learning models using smart contract system for bank loan prediction. arXiv preprint arXiv:2011.10981.
Cite this article
Wang,S.;You,S.;Zhou,S. (2023). Loan Prediction Using Machine Learning Methods. Advances in Economics, Management and Political Sciences,5,210-215.
Data availability
The datasets used and/or analyzed during the current study will be available from the authors upon reasonable request.
Disclaimer/Publisher's Note
The statements, opinions and data contained in all publications are solely those of the individual author(s) and contributor(s) and not of EWA Publishing and/or the editor(s). EWA Publishing and/or the editor(s) disclaim responsibility for any injury to people or property resulting from any ideas, methods, instructions or products referred to in the content.
About volume
Volume title: Proceedings of the 2022 International Conference on Financial Technology and Business Analysis (ICFTBA 2022), Part 1
© 2024 by the author(s). Licensee EWA Publishing, Oxford, UK. This article is an open access article distributed under the terms and
conditions of the Creative Commons Attribution (CC BY) license. Authors who
publish this series agree to the following terms:
1. Authors retain copyright and grant the series right of first publication with the work simultaneously licensed under a Creative Commons
Attribution License that allows others to share the work with an acknowledgment of the work's authorship and initial publication in this
series.
2. Authors are able to enter into separate, additional contractual arrangements for the non-exclusive distribution of the series's published
version of the work (e.g., post it to an institutional repository or publish it in a book), with an acknowledgment of its initial
publication in this series.
3. Authors are permitted and encouraged to post their work online (e.g., in institutional repositories or on their website) prior to and
during the submission process, as it can lead to productive exchanges, as well as earlier and greater citation of published work (See
Open access policy for details).