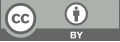
TabTranSELU: A transformer adaptation for solving tabular data
- 1 University of Edinburgh
* Author to whom correspondence should be addressed.
Abstract
Tabular data are most prevalent datasets in real world, yet the integration of deep learning algorithms in tabular data often garners less attention despite their widespread utilization in other field. This phenomenon could be attributed to the dominance of the classical algorithms in their simplicity and interpretability, and the superior performance of the gradient boosting tree models in tabular data. In this paper, a simple yet affective adaptation of the Transformer architecture tailored specifically for tabular data is presented, not only achieving good performance but also retains a high degree of explain ability. The model encodes both continuous and categorical features, alongside their respective names, and feed them into an enhanced Transformer structure enriched with Scaled Exponential Linear Unit activation. Through rigorous experimentation, our model not only outperforms classical algorithms and similar Transformer-based counterparts, but also are comparable to the performance of gradient boosting tree models.
Keywords
SELU, Tabular Data, Transformer, Deep Learning, Embedding
[1]. Chen T and Guestrin C 2016 XGBoost: A scalable tree boosting system Proceedings of the 22nd acm sigkdd international conference on knowledge discovery and data mining 785-94
[2]. Ke G, Meng Q, Finley T, Wang T, Chen W, Ma W, Ye Q and Liu TY 2017 Lightgbm: A highly efficient gradient boosting decision tree Advances in neural information processing systems, 30
[3]. Prokhorenkova L, Gusev G, Vorobev A, Dorogush A and Gulin A 2018 CatBoost: unbiased boosting with categorical features Advances in neural information processing systems 31
[4]. Friedman J H 2001 Greedy function approximation: a gradient boosting machine Annals of statistics 1189-232
[5]. He K, Zhang X, Ren S and Sun J 2016 Deep residual learning for image recognition Proceedings of the IEEE conference on computer vision and pattern recognition 770-8.
[6]. Vaswani A, Shazeer N, Parmar N, Uszkoreit J, Jones L, Gomez AN, Kaiser Ł and Polosukhin I 2017 Attention is all you need Advances in neural information processing systems 30
[7]. Klambauer G, Unterthiner T, Mayr A and Hochreiter S 2017 Self-normalizing neural networks Advances in neural information processing systems 30
[8]. Dosovitskiy A, Beyer L, Kolesnikov A, Weissenborn D, Zhai X, Unterthiner T, Dehghani M, Minderer M, Heigold G, Gelly S et al 2020 An image is worth 16x16 words: Transformers for image recognition at scale arXiv preprint arXiv:2010.11929
[9]. Gorishniy Y, Rubachev I, Khrulkov V and Babenko A 2021 Revisiting deep learning models for tabular data Advances in Neural Information Processing Systems 34 18932-43
[10]. Huang X, Khetan A, Cvitkovic M and Karnin Z 2020 Tabtransformer: Tabular data modeling using contextual embeddings arXiv preprint arXiv:2012.06678
[11]. Somepalli G, Goldblum M, Schwarzschild A, Bruss CB and Goldstein T 2021 Saint: Improved neural networks for tabular data via row attention and contrastive pre-training arXiv preprint arXiv:2106.01342
[12]. Chen J, Yan J, Chen DZ and Wu J 2023 ExcelFormer: A Neural Network Surpassing GBDTs on Tabular Data arXiv preprint arXiv:2301.02819
[13]. Wang Z and Sun J 2022 Transtab: Learning transferable tabular transformers across table Advances in Neural Information Processing Systems 35 2902-15
[14]. Hollmann N, Müller S, Eggensperger K and Hutter F 2022 Tabpfn: A transformer that solves small tabular classification problems in a second arXiv preprint arXiv:2207.01848
Cite this article
Mao,Y. (2024). TabTranSELU: A transformer adaptation for solving tabular data. Applied and Computational Engineering,51,81-88.
Data availability
The datasets used and/or analyzed during the current study will be available from the authors upon reasonable request.
Disclaimer/Publisher's Note
The statements, opinions and data contained in all publications are solely those of the individual author(s) and contributor(s) and not of EWA Publishing and/or the editor(s). EWA Publishing and/or the editor(s) disclaim responsibility for any injury to people or property resulting from any ideas, methods, instructions or products referred to in the content.
About volume
Volume title: Proceedings of the 4th International Conference on Signal Processing and Machine Learning
© 2024 by the author(s). Licensee EWA Publishing, Oxford, UK. This article is an open access article distributed under the terms and
conditions of the Creative Commons Attribution (CC BY) license. Authors who
publish this series agree to the following terms:
1. Authors retain copyright and grant the series right of first publication with the work simultaneously licensed under a Creative Commons
Attribution License that allows others to share the work with an acknowledgment of the work's authorship and initial publication in this
series.
2. Authors are able to enter into separate, additional contractual arrangements for the non-exclusive distribution of the series's published
version of the work (e.g., post it to an institutional repository or publish it in a book), with an acknowledgment of its initial
publication in this series.
3. Authors are permitted and encouraged to post their work online (e.g., in institutional repositories or on their website) prior to and
during the submission process, as it can lead to productive exchanges, as well as earlier and greater citation of published work (See
Open access policy for details).