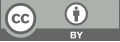
Wireless communication channel modeling based on ML
- 1 The Hong Kong University of Science and Technology
- 2 Jiangsu University of Technology
- 3 the state university of New Jersey
- 4 Beijing University of Posts and Telecommunications
* Author to whom correspondence should be addressed.
Abstract
Nowadays, wireless communication plays an important role in various fields of our lives, and in order to ensure good communication performance, reliable channel models are important. In this paper, we present a comprehensive survey of Machine Learning (ML) based channel modeling methods to help with the estimation and prediction accuracy on wireless channel modeling. To begin with, we start with a review of the traditional methods of wireless communication channel modeling, basically empirical method and deterministic method. Then, we introduce several ML methods to address limitations of traditional ways will be used for channel modeling, such as Support Vector Machine (SVM), Random Forests, autoencoder, Deep Learning, etc. Lastly, we demonstrate the application of ML methods including deep learning, SVM, and random forests on wireless channel modeling. Through learning the underlying channel distribution, Deep Learning methods have already demonstrated remarkable performance in channel modeling for different scenarios in some previous learning. Also, by training with suitable kernels and conducting sufficient training iterations, optimal hyperplanes can be identified, Support Vector Machines (SVM) can then be utilized to predict channel characteristics based on input features. Random Forests can identify the most relevant features influencing the channel and optimize system design by handling complex and high-dimensional data through iterative feature selection and splitting criteria. These methods achieve competitive accuracy with respect to traditional methods, however, there are still challenges and issues to be addressed in the application of machine learning methods for communication channel modeling.
Keywords
Channel modeling, Wireless communication, Machine Learning, Deep learning
[1]. S. M. Aldossari and K. -C. Chen, “Machine learning for wireless communication channel modeling: An overview,” Wireless Personal Communications, vol. 106, pp. 41-70, 2019.
[2]. F. Lara et al., “Modeling Wireless Propagation Channel: A Traditional Versus Machine Learning Approach,” XV Multidisciplinary International Congress on Science and Technology, Cham: Springer International Publishing, 2021.
[3]. K. A. Akpado, O. S. Oguejiofor, A. Adewale, and A. C. Ejiofor, “Pathloss Prediction for a typical mobil communication system in Nigeria using empirical models,” IRACST-Int. J. Comput. Netw. Wirel. Commun. (IJCNWC), vol. 3, pp. 207-211, 2013.
[4]. W. Khawaja, O. Ozdemir, and I. Guvenc, “UAV air-to-ground channel characterization for mmWave systems,” in Proc. IEEE Veh. Technol. Conf. (VTC-Fall), pp. 1–5, Sep. 2017.
[5]. M. Lecci et al., “Simplified ray tracing for the millimeter wave channel: A performance evaluation,” in Proc. Inf. Theory Appl. Workshop (ITA), pp. 1–6, Feb, 2020.
[6]. Z. Shi, P. Xia, Z. Gao, L. Huang, and C. Chen, “Modeling of wireless channel between UAV and vessel using the FDTD method,” in Proc. WiCOM , pp. 100–104, Sep. 2014.
[7]. T. Hu, Y. Huang, Q. Zhu, and Q. Wu, “Channel estimation enhancement with generative adversarial networks.” IEEE Transactions on Cognitive Communications and Networking, vol. 7.1, pp. 145-156, July 2020.
[8]. C. Zhang, P. Patras, and H. Haddadi, “Deep learning in mobile and wireless networking: A survey,” IEEE Commun. Surveys Tuts., vol. 21, no. 3, pp. 2224–2287, 2019.
[9]. V. Rajat, C. Gangal, M. Sharique, and M. Ansari “Deep Learning based Wireless Channel Prediction: 5G Scenario.” Procedia Computer Science, vol. 218, pp. 2626-2635, 2023.
[10]. M. Chen, U. Challita, W. Saad, C. Yin, and M. Debbah, “ML for wireless networks with artificial intelligence: A tutorial on neural networks,” CoRR, vol. abs/1710.02913, 2017.
[11]. H. He, C. Wen, S. Jin and G. Y. Li, “Deep learning-based channel estimation for beamspace mmWave massive MIMO systems,” IEEE Wireless Commun. Lett., vol. 7, no. 5, pp. 852-855, Oct. 2018.
[12]. A. Alkhateeb, S. Alex, P. Varkey, Y. Li, Q. Qu, and D. Tujkovic, “Deep learning coordinated beamforming for highly-mobile millimeter wave systems,” IEEE Access, vol. 6, pp. 37328–37348, 2018.
[13]. Mark A. Graham, Ayalvadi J. Ganesh, Robert J. Piechocki “Low Latency Allcast Over Broadcast Erasure Channels“. pp. 2023
Cite this article
Chen,X.;Zhu,W.;Shi,Y.;Zhong,Y. (2024). Wireless communication channel modeling based on ML. Applied and Computational Engineering,78,169-175.
Data availability
The datasets used and/or analyzed during the current study will be available from the authors upon reasonable request.
Disclaimer/Publisher's Note
The statements, opinions and data contained in all publications are solely those of the individual author(s) and contributor(s) and not of EWA Publishing and/or the editor(s). EWA Publishing and/or the editor(s) disclaim responsibility for any injury to people or property resulting from any ideas, methods, instructions or products referred to in the content.
About volume
Volume title: Proceedings of the 2nd International Conference on Mechatronics and Smart Systems
© 2024 by the author(s). Licensee EWA Publishing, Oxford, UK. This article is an open access article distributed under the terms and
conditions of the Creative Commons Attribution (CC BY) license. Authors who
publish this series agree to the following terms:
1. Authors retain copyright and grant the series right of first publication with the work simultaneously licensed under a Creative Commons
Attribution License that allows others to share the work with an acknowledgment of the work's authorship and initial publication in this
series.
2. Authors are able to enter into separate, additional contractual arrangements for the non-exclusive distribution of the series's published
version of the work (e.g., post it to an institutional repository or publish it in a book), with an acknowledgment of its initial
publication in this series.
3. Authors are permitted and encouraged to post their work online (e.g., in institutional repositories or on their website) prior to and
during the submission process, as it can lead to productive exchanges, as well as earlier and greater citation of published work (See
Open access policy for details).