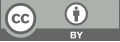
Real-time data warehousing in the big data environment: A comprehensive review of implementation in the internet industry
- 1 Beijing University of Posts and Telecommunications
- 2 Beijing University of Posts and Telecommunications
* Author to whom correspondence should be addressed.
Abstract
A real-time data warehouse is a crucial tool for information management and analysis, enabling the capture, processing, and analysis of vast amounts of data from diverse sources in real-time. It offers enterprises enhanced decision support through its efficient processing capabilities and timely data feedback. This paper reviews the technical characteristics and application scenarios of real-time data warehouses, with a particular focus on the Internet sector. It explores the evolution from traditional data warehouses to modern data lake and lakehouse architectures, emphasizing the advancements in data processing capabilities, including the separation of storage and compute functions. Real-time data warehouses, which enable immediate data processing and feedback, are essential for enterprises requiring up-to-the-minute insights. The study compares the Lambda and Kappa architectures, detailing their strengths and weaknesses in terms of data throughput, latency, and scalability. Innovations such as Apache Hudi and lakehouse architectures offer new opportunities for performance optimization and functional expansion. The emergence of hybrid architectures like HTAP (Hybrid Transactional/Analytical Processing) and HSAP (Hybrid Serving/Analytical Processing) represents a significant advancement in integrating transactional and analytical processing. Future research should focus on the impact of artificial intelligence and machine learning on real-time data warehouses to enhance their analytical and predictive capabilities, reduce complexity, and lower operational costs.
Keywords
Real-time data warehouse, Lambda architecture, Kappa architecture, Lakehouse architecture, Hybrid Serving and Analytical Processing
[1]. Sousa, R., Miranda, R., Moreira, A., Alves, C., Lori, N., & Machado, J. (2021). Software tools for conducting real-time information processing and visualization in industry: An up-to-date review. Applied Sciences, 11(11), 4800.
[2]. Gorhe, S. (2020). ETL in Near-Real Time Environment: Challenges and Opportunities. no. April.
[3]. Warren, J., & Marz, N. (2015). Big data: Principles and best practices of scalable realtime data systems. Simon and Schuster.
[4]. Hasani, Z., Kon-Popovska, M., & Velinov, G. (2014). Lambda architecture for real time big data analytic. In ICT Innovations (pp. 133–143).
[5]. Lin, J. (2017). The lambda and the kappa. IEEE Internet Computing, 21(05), 60–66.
[6]. Feick, M., Kleer, N., & Kohn, M. (2018). Fundamentals of real-time data processing architectures lambda and kappa. In SKILL 2018-Studierendenkonferenz Informatik (pp. 55-66). Gesellschaft für Informatik eV.
[7]. Nargesian, F., Zhu, E., Miller, R. J., et al. (2019). Data lake management: challenges and opportunities. Proceedings of the VLDB Endowment, 12(12), 1986-1989.
[8]. Apache Hudi. (n.d.). Retrieved from https://hudi.apache.org/
[9]. Huawei Cloud. (n.d.). FusionInsight. Retrieved from https://www.huaweicloud.com/product/FusionInsight.html
[10]. Lin, Q. I. (2021). Analysis and discussion of component in the ecosphere of Hadoop from Huawei’s FusionInsight. In 2021 IEEE 2nd International Conference on Big Data, Artificial Intelligence and Internet of Things Engineering (ICBAIE) (pp. 713-717). IEEE.
[11]. Huang, D., Liu, Q., Cui, Q., et al. (2020). TiDB: a Raft-based HTAP database. Proceedings of the VLDB Endowment, 13(12), 3072-3084.
[12]. Chen, J., Ding, Y., Liu, Y., et al. (2022). ByteHTAP: Bytedance's HTAP system with high data freshness and strong data consistency. Proceedings of the VLDB Endowment, 15(12), 3411-3424.
[13]. Jiang, X., Hu, Y., Xiang, Y., Jiang, G., Jin, X., Xia, C., Jiang, W., Yu, J., Wang, H., Jiang, Y., et al. (2020). Alibaba Hologres: A cloud-native service for hybrid serving/analytical processing. Proceedings of the VLDB Endowment, 13(12), 3272–3284.
Cite this article
Qu,J.;Wang,J. (2024). Real-time data warehousing in the big data environment: A comprehensive review of implementation in the internet industry. Applied and Computational Engineering,88,105-114.
Data availability
The datasets used and/or analyzed during the current study will be available from the authors upon reasonable request.
Disclaimer/Publisher's Note
The statements, opinions and data contained in all publications are solely those of the individual author(s) and contributor(s) and not of EWA Publishing and/or the editor(s). EWA Publishing and/or the editor(s) disclaim responsibility for any injury to people or property resulting from any ideas, methods, instructions or products referred to in the content.
About volume
Volume title: Proceedings of the 6th International Conference on Computing and Data Science
© 2024 by the author(s). Licensee EWA Publishing, Oxford, UK. This article is an open access article distributed under the terms and
conditions of the Creative Commons Attribution (CC BY) license. Authors who
publish this series agree to the following terms:
1. Authors retain copyright and grant the series right of first publication with the work simultaneously licensed under a Creative Commons
Attribution License that allows others to share the work with an acknowledgment of the work's authorship and initial publication in this
series.
2. Authors are able to enter into separate, additional contractual arrangements for the non-exclusive distribution of the series's published
version of the work (e.g., post it to an institutional repository or publish it in a book), with an acknowledgment of its initial
publication in this series.
3. Authors are permitted and encouraged to post their work online (e.g., in institutional repositories or on their website) prior to and
during the submission process, as it can lead to productive exchanges, as well as earlier and greater citation of published work (See
Open access policy for details).